What is Data Observability? Why it Matters for AI Readiness?
What is Data Observability? Why it Matters for AI Readiness? https://www.dqlabs.ai/wp-content/uploads/2024/04/2-1024x575.png 1024 575 DQLabs DQLabs https://www.dqlabs.ai/wp-content/uploads/2024/04/2-1024x575.pngWhile data quality is the foundation for effective AI, it’s not enough on its own. Just like a high-performance car needs a well-maintained road network to function optimally, high-quality data requires data observability to deliver its full potential for AI initiatives.
So what is data observability, and why is it crucial for organizations gearing up for the AI revolution?
Data observability refers to the ability to observe, monitor, and understand the behavior and performance of data systems in real-time. It encompasses the visibility into data pipelines, processes, and infrastructure, allowing organizations to ensure data reliability, quality, and availability.
In simpler terms, it’s like having a comprehensive radar system that tracks every data point’s journey, from its source to its utilization, providing insights into its health and performance along the way. Imagine a complex data pipeline as a network of roads. Data observability acts like a sophisticated traffic management system. It tracks data flow, identifies bottlenecks, and monitors the health of the entire infrastructure. This allows you to proactively address issues and ensure smooth data delivery for your AI models.
Five Pillars of Data Observability
Based on latest Gartner’s definition of Data Observability it touches five main pillars that includes:
TYPES OF OBSERVATION | DESCRIPTION | EXAMPLE – WHAT IS OBSERVED? |
Data Content | Monitor the quality, accuracy, and completeness of your data. Identifies anomalies, errors, and patterns. | Profiling historical data to detect changes in values or unexpected trends. |
Data Flow & Pipeline | Tracks how data moves through pipelines, identifying bottlenecks and configuration issues. | Notifying when a data pipeline job fails to complete. |
Infrastructure & Compute | Checks the performance and health of your data infrastructure (servers, storage, networks). | Monitoring CPU usage spikes on database servers. Or – Monitoring resource utilization and triggering alerts if consumption reaches critical levels. |
Users, Usage & Utilization | Tracks who uses your data, how often, and what queries they run. Helps identify unusual activity. | Identifying users with unexpectedly high data access or query volumes. (e.g., User activity monitoring within a DQLabs platform) |
Financial Allocation | Tracks the cost associated with data storage, processing, and pipelines. Helps optimize spending. | Identifying expensive datasets or inefficient pipelines for cost optimization. |
The Need for Data Observability
Data quality, ensuring clean and accurate data for AI training, remains a crucial foundation. But even with high-quality data, questions linger:
Is the AI model functioning as intended?
- How can we ensure that our AI models are performing optimally in real-world scenarios?
- Are there any specific indicators we should monitor to detect potential issues or anomalies in our data & AI systems
- How can we identify deviations in AI model behavior – what is the root cause of it, how can we fix these issues and where did it originate?
Are data pipelines delivering clean data consistently?
- What measures are in place to ensure the reliability and consistency of our data pipelines?
- How do we monitor the quality and integrity of data flowing through our pipelines in real-time?
- What are the key metrics in place to track the root cause of problems, are there any possible problems we can predict and stop from happening?
Are there hidden biases or errors impacting results?
- How do we identify and mitigate potential biases or errors in our data that may affect AI model outcomes?
- What techniques or methodologies can we employ to uncover hidden biases or disparities in our datasets?
Data observability goes beyond data quality —it works as an indispensable companion to data quality in the quest for AI readiness. While data quality focuses on the intrinsic characteristics of data, such as accuracy and completeness, data observability broadens the scope, offering insights into the operational health of both data and AI systems. It functions as a continuous monitoring system, providing real-time visibility into the performance of data pipelines, the efficacy of AI models, and the presence of potential issues that could impact outcomes.
Benefits of Data Observability for AI Readiness
Here’s how data observability empowers you to build robust and reliable AI systems:
- Proactive Problem Identification: Early detection of anomalies or data quality issues within pipelines allows for swift intervention, preventing downstream impacts on AI models.
For instance, consider a scenario where a sudden spike in data latency is detected within a data ingestion pipeline. Through data observability, organizations can promptly identify this anomaly, investigate its root cause, and take corrective action to rectify the issue before it cascades downstream, potentially impacting the performance of AI models. By proactively addressing such issues, organizations can ensure the integrity and reliability of their AI-driven insights and decision-making processes.
- Improved Model Explainability: By understanding data flow and feature behavior, data observability helps explain model outputs, fostering trust and interpretability.
For example, suppose an AI model tasked with predicting customer churn rates generates unexpected results. By leveraging data observability techniques, organizations can trace the lineage of input features, identify influential factors or biases, and elucidate the rationale behind the model’s predictions. This enhanced model explainability fosters trust among stakeholders, enabling them to comprehend and validate the decisions made by AI systems.
- Enhanced Model Performance: Continuous monitoring allows for data lineage analysis and fine-tuning of data pipelines, optimizing model performance and driving better results.
For instance, consider a machine learning model deployed for fraud detection in financial transactions. By scrutinizing the data flow and transformations within the model’s pipeline, organizations can identify inefficiencies or bottlenecks that impede performance. Armed with this insight, organizations can fine-tune data preprocessing steps, adjust model parameters, or incorporate additional features to enhance the model’s predictive accuracy and efficacy. Ultimately, data observability empowers organizations to unleash the full potential of their AI models, driving superior results and business outcomes.
- Reduced Downtime and Costs: Proactive issue identification minimizes downtime and potential financial losses associated with inaccurate AI outputs.
For example, suppose an AI-powered recommendation engine experiences a sudden drop in performance due to data quality issues in the underlying recommendation dataset. With data observability tools in place, organizations can swiftly pinpoint the source of the problem, whether it’s data drift, schema changes, or input feature anomalies. By taking immediate corrective action, organizations can minimize downtime, prevent revenue loss, and safeguard their reputation. Additionally, by preemptively addressing data quality issues, organizations can mitigate the financial risks associated with erroneous AI outputs, such as misinformed business decisions or regulatory non-compliance.
Building a Data Observability Culture
Implementing data observability requires a strategic approach:
- Define Metrics and KPIs: Establish key performance indicators (KPIs) to monitor data flow, model performance, and potential biases. These metrics serve as yardsticks for monitoring the health and performance of data pipelines, AI models, and the overall data ecosystem. Examples of key metrics include data quality scores, pipeline throughput, latency, error rates, model accuracy, bias detection, and adherence to regulatory compliance requirements. By defining and tracking these metrics over time, organizations can gain actionable insights into the operational efficiency, reliability, and compliance of their data-driven systems.
- Invest in Tools and Technologies: Utilize platforms designed to offer real-time insights into data pipelines and model behavior. Organizations should invest in purpose-built platforms like DQLabs Modern Data Quality Platform equipped with features such as data lineage tracking, anomaly detection, automated alerting, and visualization dashboards. These tools empower data teams, data scientists, and business stakeholders to monitor data flows, detect deviations or anomalies, and troubleshoot issues in real-time. Moreover, leveraging technologies like machine learning and artificial intelligence can augment data observability efforts by automating repetitive tasks, identifying patterns, and predicting potential data quality issues or model failures before they occur.
With its comprehensive suite of features, DQLabs enables organizations to gain end-to-end visibility into their data ecosystem, from data ingestion to model deployment. By leveraging advanced algorithms and analytics, DQLabs empowers organizations to detect, diagnose, and resolve data quality issues, ensuring the reliability and accuracy of their AI-driven insights. Additionally, DQLabs fosters collaboration and knowledge sharing across teams through its intuitive user interface, interactive dashboards, and customizable workflows. With DQLabs, organizations can build a culture of data observability that drives continuous improvement and innovation across their AI initiatives.
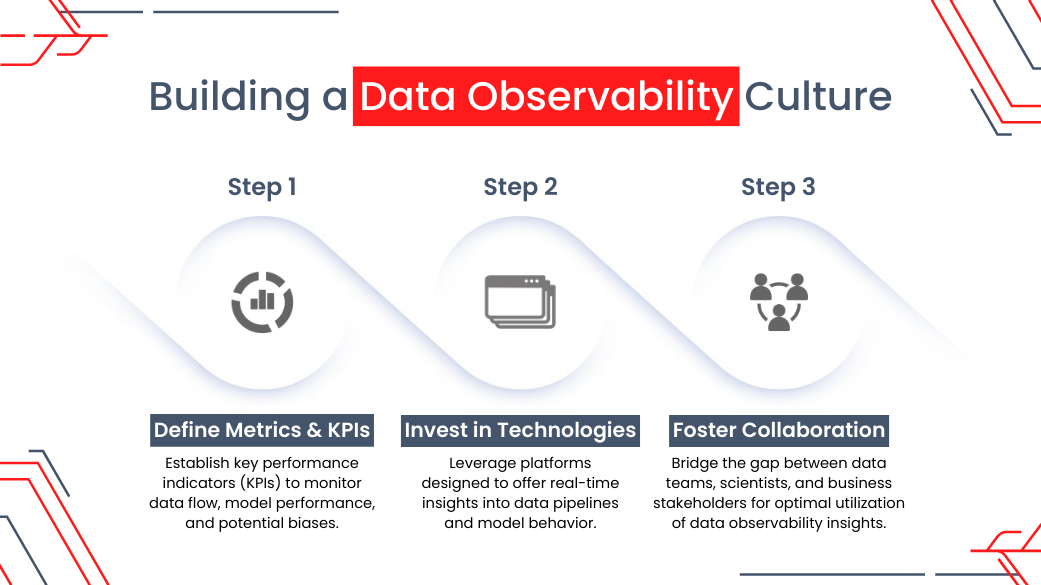
- Foster Collaboration: Break down silos between data teams, data scientists, and business stakeholders to ensure everyone utilizes data observability insights effectively. By breaking down silos and fostering cross-functional collaboration, organizations can ensure that data observability insights are leveraged effectively to drive business value and inform decision-making. For example, data engineers can work closely with data scientists to monitor and optimize data pipelines, while business stakeholders can provide domain expertise and context to interpret the implications of data observability findings. Additionally, fostering a culture of transparency and accountability encourages all stakeholders to take ownership of data quality and contribute to continuous improvement efforts.
Trustworthy AI starts with data observability
Data observability empowers organizations to move beyond a “black box” approach to AI. By shedding light on data flow, model behavior, and potential issues, it fosters trust in AI outputs and paves the way for responsible and successful AI implementation. By enforcing data observability as a core component of their AI strategy, organizations can leverage proactive monitoring and a culture of data transparency to harness the power of AI with confidence.
As we conclude our exploration of data observability and its critical role in AI readiness, it’s essential to highlight the role of solutions like DQLabs in this landscape. DQLabs offers a full stack Data Observability solution as part of its Modern Data Quality Platform, tailored to meet the evolving needs of today’s enterprises. With DQLabs, organizations can proactively monitor and analyze their data pipelines, model performance, and potential biases in real-time. By leveraging automation, contextual insights, and advanced analytics, DQLabs empowers businesses to unlock the full potential of their data, driving informed decision-making and fostering AI-driven innovation at scale. Embrace the power of data observability with DQLabs and embark on a journey towards AI readiness and success.