The Role of Data Quality Management in Healthcare Innovation
The Role of Data Quality Management in Healthcare Innovation https://www.dqlabs.ai/wp-content/uploads/2024/09/thumbnail-10-1024x575.webp 1024 575 DQLabs DQLabs https://www.dqlabs.ai/wp-content/uploads/2024/09/thumbnail-10-1024x575.webpData quality management is essential in any field, but in healthcare, it is critical. In an industry where patient information is both highly sensitive and critical to care delivery, maintaining accurate and reliable data is important. If medical records are incomplete or inaccurate, it can lead to misdiagnosis, incorrect treatments, and in some cases, life-threatening errors.
When healthcare providers have access to accurate, complete information, they can make smarter decisions. Patients receive better care, outcomes improve, and trust in the system strengthens. This shows high-quality data isn’t just a box to check—it’s the basis for better healthcare analytics and quality patient care.
Without trustworthy, reliable data, even the most advanced AI applications won’t deliver the desired results. And that’s where the challenge lies. AI, in particular, faces a tough time with the public perception. Pew Research found that 52% of Americans are more worried than excited about AI in their daily lives, with only 10% expressing more excitement than concern. A recent poll by MITRE-Harris shows that just 39% of American adults believe AI technologies are safe and secure, which is a drop of nine percentage points since late 2022.
For AI to succeed, especially in healthcare, the data fueling these technologies must be of the highest quality—complete, governed, and accessible. Whether for doctors, insurers, researchers, or patients, the value and outcomes AI promises are only as strong as the data behind it.
Paradigm Shifts In The Healthcare Industry
While the healthcare sector is undergoing a major transformation, organizations are turning to data and AI to navigate these four key shifts.
- Growing Regulatory Pressure: Regulatory initiatives like the 21st Century Cures Act and FHIR standards are pushing for real-time data exchange across systems, enabling a holistic patient view and improved care coordination. Providence, a U.S. healthcare network, uses real-time data streaming to enhance patient care and improve emergency department performance.
- Increasing Acceptance of Real-World Evidence (RWE): Real-world evidence, derived from data outside clinical trials, offers new insights into drug effectiveness and safety. Regulators, like the FDA, have embraced RWE through guidelines on its use. Analysts in this industry must adopt flexible data platforms and ensure transparency in data governance to support RWE’s rise.
- Innovations in Personalized Medicine and Prevention: Personalized medicine is evolving, with genomic sequencing now available at a fraction of its original cost. Genomics, along with image data and liquid biopsies, are reshaping drug development and cancer detection. As personalized care evolves, data growth is expected to rise, requiring modernized data platforms to deal with them.
- Rise of Telemedicine and Digital Care Models: The pandemic fast-tracked the shift from traditional care settings to virtual environments, like telemedicine and at-home care. Wearable devices and digital health platforms are seeing widespread adoption, relying on real-time patient data collection and analysis to support these new models of care.
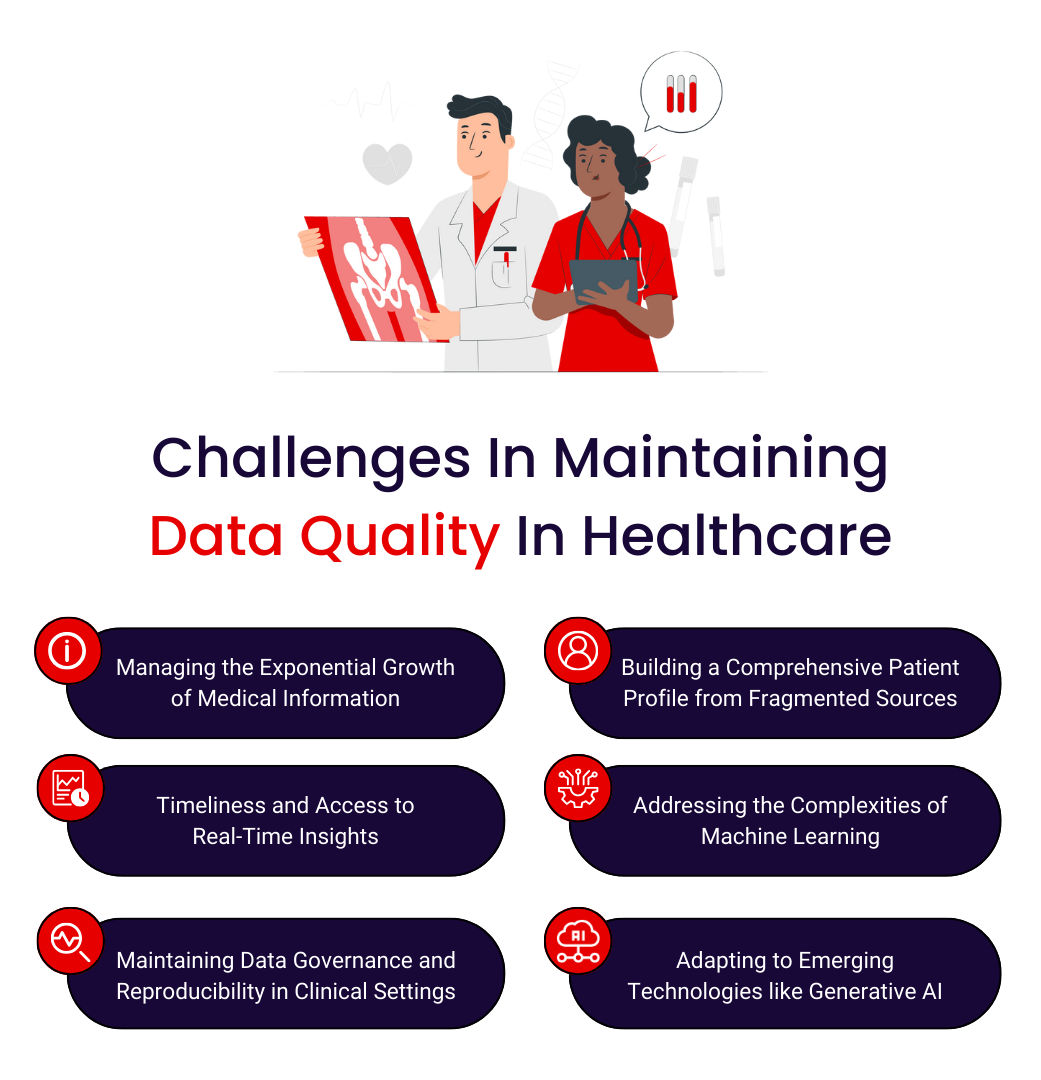
Ways To Ensure Data Quality In Healthcare
The quality of data can be measured through various characteristics that highlight its value. These traits may shift depending on the specific needs the data is meant to meet. However, certain key dimensions are crucial for the accurate and effective use of data, especially in healthcare. Below, we outline some core data quality attributes in healthcare, complete with definitions and real-world examples. While this list isn’t exhaustive, it offers a solid foundation for understanding the essential elements of data quality in this industry. Understanding these basics is important before diving into strategies for ensuring data quality.
No | Characteristic | Meaning | Example |
1 | Availability and accessibility | Data is readily available when required and accessible to authorized users | In an electronic health record system, clinical information is instantly accessible when needed |
2 | Accuracy | Data accurately reflects reality and truth | Patient monitors’ vital signs are precisely recorded in the patient’s medical record |
3 | Validation | Data conforms to the correct format and falls within the appropriate domain | Vital statistics like body temperature and blood pressure are within medically acceptable ranges |
4 | Completeness | Data is comprehensive and includes all necessary information | Prescriptions include all required details: drug names, prescriber, dosage, issue date, and expiration |
5 | Freshness | Data is up-to-date and reflects the most recent information | Patient’s electronic health record is promptly updated with new diagnosis information |
6 | Consistency | Data maintains uniformity across different sources and systems | Patient information remains consistent whether accessed via EHR or at a community health center |
7 | Identifiability | Data uniquely identifies entities without duplication | Each electronic health record has a unique identifier, preventing duplicate entries for the same patient |
8 | Provenance | Data is stored with its metadata, including origin and update history | EHR systems maintain a comprehensive history of record creation, updates, and modifications |
9 | Usability | Data is presented in a format easily understood by intended users | Healthcare records use standardized, approved abbreviations and codes that are clear to medical professionals |
10 | Security and confidentiality | Data is protected from unauthorized access, and patient privacy is maintained | Patient records are accessible only to authorized medical staff, with sensitive information securely masked in public records |
Now, let’s explore some key processes that can identify and resolve data quality issues, ensuring that health data is accurate and reliable. These steps form an end-to-end framework designed to maintain consistency and trust in your data.
- Profile Health Data Sources: Start by profiling your data. This involves assessing its structure, identifying gaps, and finding out the hidden issues. Profiling helps detect missing information, duplicates, incorrect formats, or values that fall outside acceptable ranges. It’s the first step in spotting areas that need cleansing or correction.
- Fill in Missing Information: Once you’ve identified gaps, the next step is to address them. Missing data can often be filled in using other datasets or by reaching out to staff or patients. The goal is to ensure the data is as complete as possible to avoid errors later on.
- Clean and Standardize Data: Data cleansing is all about ensuring consistency. This includes removing or replacing empty values, correcting formatting issues, merging similar columns, and transforming data to a standard format. It’s essential for creating a unified view across multiple datasets, making the data easier to use and analyze.
- Match Duplicate Patient Records: Duplicate patient records can lead to confusion and errors. Patient data matching compares records to determine if they belong to the same person. If unique identifiers like Social Security numbers aren’t available, fuzzy matching algorithms help estimate whether two records are for the same patient.
- Eliminate Duplicate Records: Deduplication is the process of removing redundant entries while keeping the most accurate version. It’s crucial for maintaining clean, organized databases that don’t overload systems with unnecessary data.
- Merge and Retain Important Data: Finally, merging records and using survivorship rules ensures that valuable information isn’t lost during deduplication. This step ensures you retain the most relevant data while eliminating duplicates, preserving the accuracy and integrity of patient records.
Factors To Consider While Implementing Data Quality Management
When ensuring data quality in healthcare, there are several critical aspects besides the standard data management practices. Here are eight important factors to consider:
- Privacy and Security: Data quality efforts must prioritize patient privacy and data security. Implement solid encryption, access controls, and monitoring systems to protect sensitive information from breaches or unauthorized access.
- Data Lineage and Provenance: Knowing where your data comes from and how it’s transformed is crucial. Keeping clear records of data history ensures trust, transparency, and traceability.
- Integrating Multiple Data Sources: Healthcare data often comes from diverse systems—EHRs, labs, imaging, and billing. Invest in integration tools that maintain data consistency and smooth data exchange across these platforms.
- Real-Time Data Quality: As real-time data becomes more critical for patient care and decision-making, data quality processes need to be just as responsive. Focus on ensuring accuracy across both real-time streams and historical data.
- Patient-Generated Data: Wearable devices and remote monitoring produce increasing amounts of patient data. Establish procedures to validate and standardize this data to ensure its usefulness and reliability.
- AI and Machine Learning: Data quality is foundational for AI and machine learning. Poor data leads to flawed predictions and biased results, so ensure your data is clean and trustworthy for advanced analytics.
- Continuous Improvement: Data quality isn’t a one-time task. Regular audits and improvements are vital. Create feedback loops to learn from errors and refine your processes over time.
- Change Management: Introducing data quality initiatives can bring cultural shifts. Develop a change management strategy to engage stakeholders and smooth the transition to new practices.
Improving Data Quality Management In Healthcare With DQLabs
When choosing a data platform, the benefits often revolve around the strength of its performance, ease of use, and collaborative capabilities. Here’s a breakdown of how these key advantages enhance the overall platform experience:
Scalability and Compute Performance
DQLabs excels in scalability and compute performance by leveraging advanced techniques like parallelization, grid computing, and load balancing. These capabilities enable efficient distribution of data quality tasks across multiple nodes, significantly enhancing performance even with large datasets. The platform also supports clustered environments, ensuring high availability and fault tolerance. With real-time insights and proactive incident management, DQLabs maintains optimal performance while preventing bottlenecks. This scalable architecture allows businesses to seamlessly handle growing data volumes, ensuring data quality and reliability across various environments, from on-premises systems to modern cloud infrastructures.
Smart Data Curation for Holistic Patient View
Offering tailored support for industries like healthcare, DQLabs simplifies data curation with domain-specific data types. The platform automates data curation using AI/ML and semantic tagging, ensuring that data is organized with relevant context. For healthcare, this creates a holistic patient view by integrating diverse data sources, from clinical records to IoT and genomic data. By making high-quality, domain-specific data easily accessible, DQLabs enables healthcare professionals to make informed decisions, improving patient outcomes.
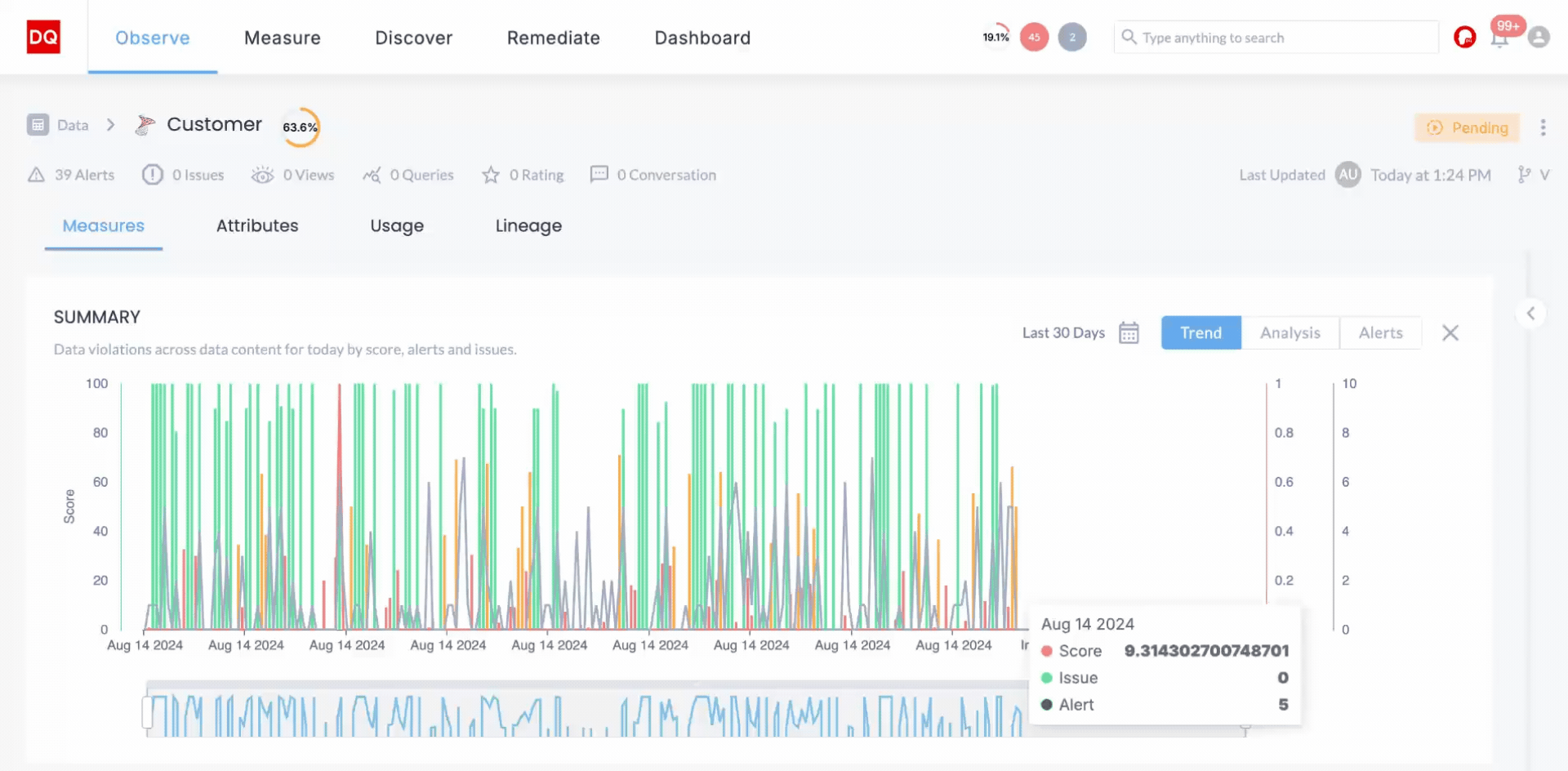
Near Real-Time Insights and Proactive Data Incident Management
DQLabs delivers near real-time insights and proactive data incident management, ensuring businesses can stay ahead of potential data quality issues. DQLabs monitors your data both at rest and in motion. This capability ensures that data is continuously assessed for quality, even as it is being generated, which is crucial for dynamic environments like healthcare and finance.
Anomaly detection is another key feature of DQLabs, powered by AI-augmented models. The platform identifies issues such as unexpected missing values, variance deviations, and changes in attribute distribution. It uses machine learning techniques, to detect distribution changes across multiple attributes, ensuring anomalies are caught early. This proactive monitoring is further enhanced by real-time alerting through integrations with Slack, Teams, and email, allowing teams to respond quickly.
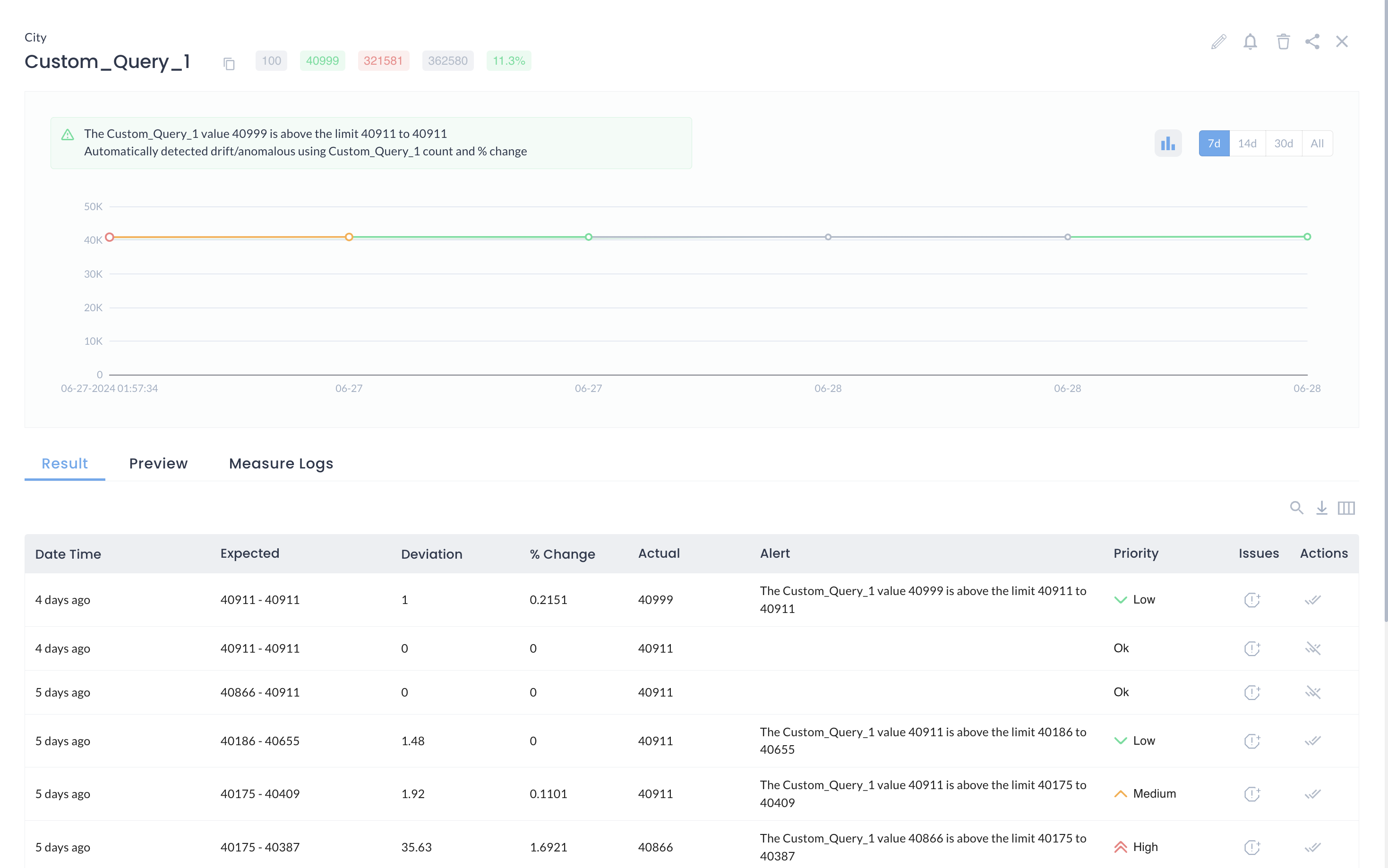
In addition to real-time monitoring, DQLabs supports batch processing for scheduled or ad-hoc data quality tasks. With DQLabs, organizations can trust that their data is accurate, reliable, and continuously monitored for any potential issues.
Collaborative and Easy to Manage Data Quality and Reliability
DQLabs offers a user-friendly platform designed for seamless collaboration and reliable data quality management, for both data producers and consumers alike. With its intuitive, role-based interface, business users can easily create and manage data quality processes with minimal tech support. The platform promotes collaboration through integrated tools like Slack and Jira, allowing teams to address issues in real-time. Reliability is ensured through robust checks that monitor data quality before it enters data warehouses, preventing the spread of bad data. DQLabs also includes circuit breaker APIs to halt data pipelines when issues are detected, safeguarding the entire data ecosystem. Customizable dashboards provide insights into data quality, with automated alerts and reports enhancing decision-making. This comprehensive approach simplifies managing data quality, promoting both collaboration and reliability across the organization.
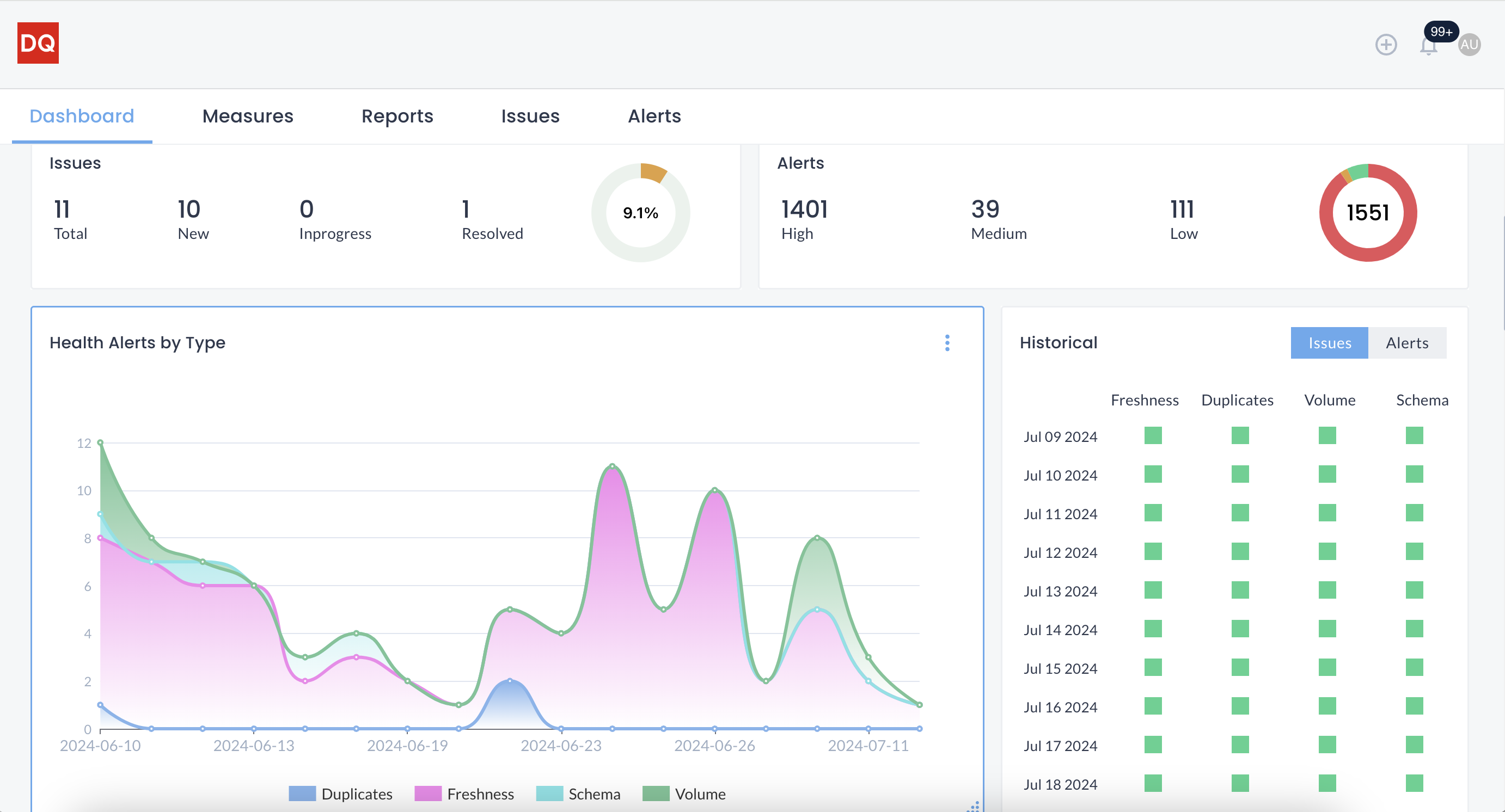
Get Trusted Data For Your Healthcare Analytics With DQLabs
DQLabs’ intuitive interface, real-time monitoring, and proactive incident management help organizations ensure data reliability and streamline operations. With the ability to handle data from diverse sources, detect anomalies, and provide real-time insights, DQLabs supports healthcare professionals in making informed decisions based on accurate and high-quality data.
To see how DQLabs can enhance your organization’s data quality management and improve patient outcomes, request a demo today.