The Need for Data Quality Management in the Life Sciences Industry
The Need for Data Quality Management in the Life Sciences Industry https://www.dqlabs.ai/wp-content/uploads/2024/10/the-need-for-data-quality-management-in-the-life-sciences-industry-thumb-1024x575.webp 1024 575 DQLabs DQLabs https://www.dqlabs.ai/wp-content/uploads/2024/10/the-need-for-data-quality-management-in-the-life-sciences-industry-thumb-1024x575.webpLife sciences continue to make quantum leaps into their future with each passing moment. The last pandemic has made it evident that data is a key enabler for swift measures in response to market fluctuations and the stimulation of innovation. Data-driven initiatives have been invaluable in everything from accelerating clinical trials to optimizing manufacturing processes for vaccines. Hence, data-driven decision-making will, going forward, emerge as the new operating standard in life sciences.
In fact, industry leaders are increasingly recognizing the potential this approach has in aiding drug discovery, patient engagement, and cost optimization—just to name a few spheres for which this holds true.
However, most life sciences organizations work with outdated systems, fragmented data sources, and complex regulatory requirements—all requiring an approach to overcome these challenges.
Key Challenges Faced by the Life Sciences Industry
This industry has been navigating several key challenges as it seeks to leverage data-driven technologies for growth and transformation:
- Legacy Systems and Fragmented Data: Many life sciences organizations still rely on outdated IT infrastructure, which hampers data integration and accessibility. Fragmented data sources across the value chain pose significant challenges, making it difficult to extract meaningful insights efficiently.
- Non-Standardized Data Architectures and Taxonomies: The life sciences industry often suffers from non-standardized data architectures, where different departments or systems use varying formats, definitions, and classifications for similar types of data.
- Data Interoperability: The lack of standardized data formats and interoperability between systems makes collaboration and compliance difficult. Disparate systems and regulatory variations across regions further complicate data-sharing initiatives.
- Access Control Issues: Access control is a significant concern in life sciences due to the sensitive nature of the data involved, including patient information and proprietary research findings. The challenge intensifies when dealing with blinded (where certain information is withheld) versus unblinded (where all information is available) datasets.
- Regulatory Compliance: Life sciences companies must adhere to stringent regulations (HIPAA, GDPR, etc.), especially in managing patient data and clinical trials. These complex regulatory requirements increase compliance costs and complicate data management.
- Cybersecurity Risks: With the growing volume of sensitive medical and research data, the threat of cyberattacks looms large. Companies need to bolster their data privacy and security protocols to protect against breaches.
- Demand for Quality Data: High-quality data is critical for the success of strategic technology initiatives. Data leaders must implement robust Data Quality Management (DQM) practices to ensure data accuracy and reliability, enhancing clinical decision-making and patient safety.
- Slow Digital Transformation: Despite recognizing the potential of digital technologies like AI and machine learning, many organizations struggle with the speed of transformation. Limited digital maturity, combined with economic headwinds, puts pressure on companies to balance innovation with operational efficiency.
- Increased Demand for Innovation: Data leaders are under pressure to use technologies like AI, hyper automation, and cloud-native platforms to drive innovation. This demand stems from the need to enhance operational efficiency, improve patient outcomes, and adapt to evolving consumer expectations. Prioritizing investments in these technologies is essential for remaining competitive.
- Building a Distributed Enterprise Model: The shift to hybrid work environments calls for managing distributed teams. This includes integrating remote work capabilities into care delivery models and ensuring technology infrastructure supports seamless collaboration.
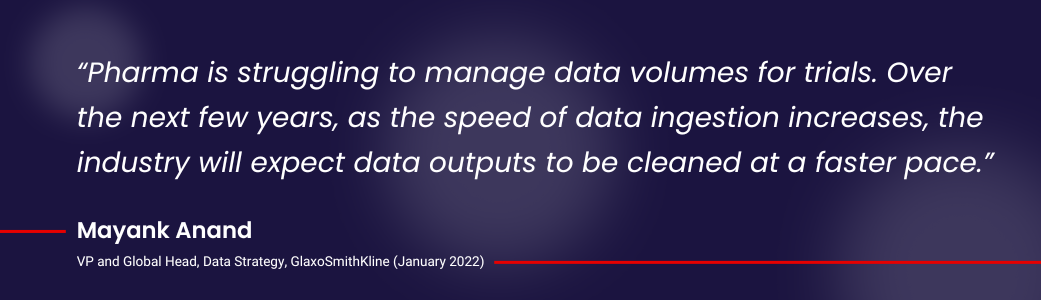
Focusing On High-Impact Use Cases For Transformative Success
To effectively tackle these challenges, companies in this industry must integrate data and analytics as core business functions rather than optional initiatives. The first step toward becoming data-driven is identifying and prioritizing use cases across the value chain to align with business goals. A structured framework can help organizations focus on high-impact use cases that accelerate value realization, measuring time-to-value through tangible benefits like reduced trial timelines and improved forecasting.
- Quick wins: Life sciences enterprises should prioritize use cases in manufacturing and marketing to quickly generate measurable profits through improved asset management, sourcing decisions, and sales force management.
- Business resilience: Investments in Health Care Professionals (HCP) targeting, signal management, and quality control will strengthen business units, enabling organizations to navigate future uncertainties.
- Operational efficiency: Initiatives like remote patient care, patient recruitment, and social media mining can enhance patient-centric trials, offering a competitive edge.
- Transformational change: For sustainable competitive advantage, organizations must invest in bold initiatives like accelerating drug development and optimizing manufacturing resources.
How Data Quality Management Helps
Life sciences and pharmaceutical sectors depend heavily on data for activities like drug discovery, clinical trials, and compliance with regulatory standards. Without effective Data Quality Management (DQM), organizations risk regulatory non-compliance, inefficiencies, and poor decision-making, which can ultimately hinder their ability to deliver safe and effective treatments to patients.
Enhancing regulatory compliance
Pharmaceutical companies operate under strict regulatory requirements, including Good Manufacturing Practice (GMP) and Good Clinical Practice (GCP). Regulatory bodies such as the FDA and EMA require pharmaceutical data to be accurate, complete, and traceable. DQM ensures that organizations meet these requirements by implementing robust data validation processes and maintaining data integrity throughout the drug development lifecycle. A lack of high-quality data can lead to costly delays in drug approvals, fines, or even product recalls.
Improving clinical trials
Clinical trials generate massive amounts of data from various sources, such as patient records, wearable devices, and laboratory results. Ensuring the integrity of this data is critical to reducing errors, minimizing biases, and accelerating drug development. According to research by Everest Group, data management in clinical trials helps streamline processes such as patient recruitment, remote monitoring, and protocol design.
For instance, poor data quality could result in inconsistencies that may require protocol amendments, ultimately delaying the trial. Effective DQM ensures accurate and comprehensive data collection, leading to more reliable results and faster trial completion. Ensuring that data is accurate and reliable accelerates drug discovery and enables the generation of precise hypotheses at scale. This also translates to cost and time savings, as fewer resources are wasted on inaccurate or redundant data.
Furthermore, decentralized clinical trials, which have gained momentum during the COVID-19 pandemic, rely heavily on data collected from remote sources like sensors and mobile apps. Managing this data effectively ensures that trials are conducted with fewer disruptions, improving time-to-market for new therapies.
Accelerating drug discovery
In the drug discovery phase, pharmaceutical companies use advanced data analytics, including AI and machine learning (ML), to predict drug-target interactions and identify promising compounds. However, the effectiveness of these algorithms depends on the quality of the underlying data. Data quality management processes, such as data cleansing and harmonization, are essential for feeding clean, consistent data into these models.
By ensuring that the data used for drug discovery is accurate and complete, DQM helps reduce the time and resources required to bring a drug from the laboratory to the market. This is particularly important in cases of drug repurposing, where existing drugs are tested for new therapeutic uses. High-quality data accelerates this process by improving predictive accuracy and reducing trial-and-error experimentation.
Increasing operational efficiency
The life sciences and pharmaceutical industries face operational inefficiencies stemming from data silos, legacy systems, and inconsistent data formats. Poor data quality can exacerbate these inefficiencies, leading to higher costs and longer lead times. Implementing a comprehensive DQM framework helps streamline workflows by integrating data across various departments—from R&D to manufacturing and sales.
For example, in the manufacturing phase, predictive maintenance of equipment and optimization of production schedules rely on accurate real-time data. DQM ensures that the data used to drive these processes is reliable, helping companies minimize downtime and reduce waste. In supply chain management, data quality is critical for demand forecasting, logistics optimization, and ensuring product traceability, all of which contribute to operational efficiency.
Additionally, data traceability and visibility are improved, ensuring that life sciences companies can track and validate data throughout its lifecycle. This promotes trust within the organization, as well as with external partners and regulators, reducing the risks associated with poor data management.
Ensuring data security and privacy
The life sciences industry handles sensitive medical data, making data privacy and security top priorities. Inaccurate or poor-quality data increases the risk of cyberattacks and breaches, which can compromise patient safety and corporate reputation. DQM mitigates these risks by enforcing data governance policies and ensuring compliance with regulations like HIPAA and GDPR. A robust DQM system helps secure patient information while allowing organizations to harness data for research and innovation.
DQLabs Facilitating Innovation and Compliance
DQLabs is a unified platform that significantly enhances data quality management for life sciences organizations by integrating Data Quality, Data Observability, and Data Discovery. It offers automated monitoring with over 50 checks for data health, ensuring compliance with stringent regulatory standards critical in the industry. With tools for maintaining data quality and efficient issue resolution workflows, DQLabs helps data teams to manage clinical trial data and patient information effectively. Advanced AI capabilities provide data engineers with automated anomaly detection, while data scientists can assess quality against business outcomes. For data leaders, DQLabs enhances strategic decision-making by tracking quality at asset, attribute, and KPI levels, ensuring reliable insights that drive innovation and improve patient outcomes in the rapidly evolving life sciences industry.
Join our upcoming webinar, where Wipro and Alation are partnering with DQLabs to present our Data Trust for AI solution tailored to the life sciences sector. Discover how Data Trust is essential for addressing current challenges, the importance of involving people and technology in implementing trusted AI solutions, and explore Gen AI capabilities across various functional areas.
Don’t miss this opportunity, Register today!