Modern Data Quality Summit 2023: Recap & Highlights
Modern Data Quality Summit 2023: Recap & Highlights https://www.dqlabs.ai/wp-content/uploads/2024/07/thumbnail-data-pipeline-blog-31-1024x575.png 1024 575 DQLabs DQLabs https://www.dqlabs.ai/wp-content/uploads/2024/07/thumbnail-data-pipeline-blog-31-1024x575.pngThe Modern Data Quality Summit 2023 was a landmark event for DQLabs that brought together leading data experts, engineers, and thought leaders to discuss and share insights on the latest trends, technologies, and methodologies in data quality. As we gear up for this year’s event, let’s take a look back at the key highlights and takeaways from the 2023 summit, which set the stage for a new era of data quality and governance.
Rethinking DQ In The World Of Data Mesh
Speaker: Zhamak Dehghani, CEO – Nextdata
The summit kicked off with an insightful session by Zhamak Dehghani, focusing on the evolution of data quality within modern data infrastructure and emphasizing the importance of embedding quality from the data’s inception. Dehghani, known for her creation of the Data Mesh concept, brought a fresh perspective on how organizations can evolve their data quality practices.
Shift Left Approach
Historical reactive approaches to data quality often led to inefficiencies. Quality must be embedded from the start. In the past, organizations often addressed data quality issues reactively, leading to inefficiencies and inconsistencies. Dehghani emphasized the importance of embedding data quality practices from the inception of data creation.
Building quality into the data creation process ensures higher accuracy and relevance. The “shift left” approach involves moving data quality practices to the earlier stages of the data lifecycle. By doing so, organizations can identify and address issues before they propagate through the system.
This proactive approach reduces the risk of errors and improves the overall quality of the data. For example, in a retail organization, implementing data quality checks at the point of sale ensures that customer and transaction data is accurate and reliable. This approach reduces the risk of errors in downstream processes, such as inventory management and sales analysis.
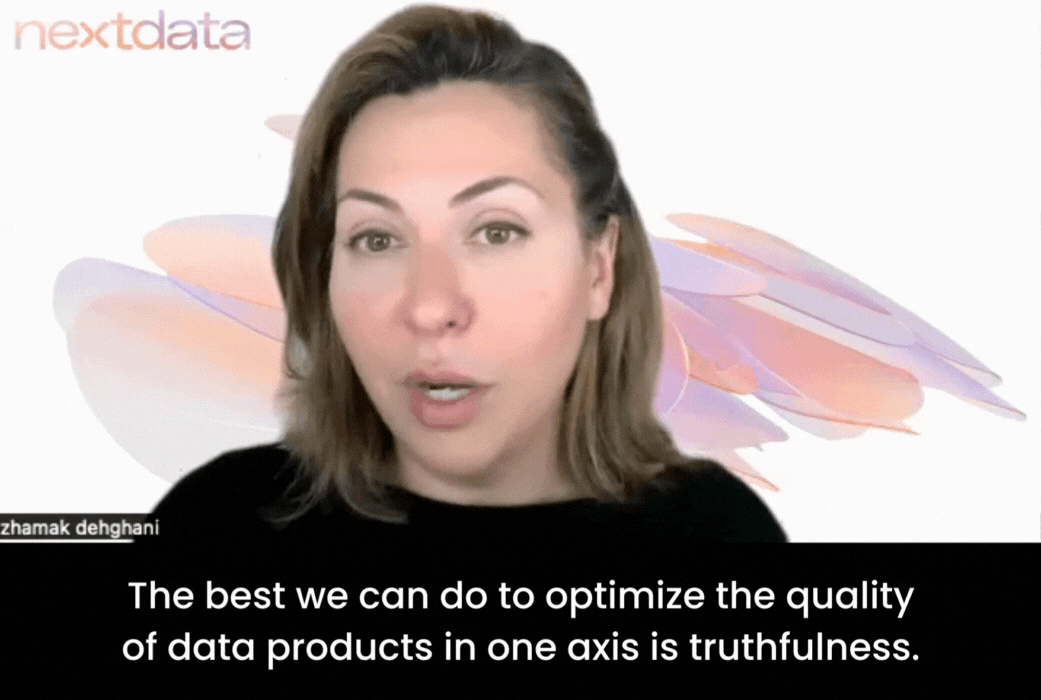
Data Quality in the AI Era
Accurate and timely data is crucial for AI applications, highlighting the need for robust data quality frameworks. In the era of artificial intelligence and machine learning, the quality of data is more important than ever. Accurate and timely data is crucial for training AI models and ensuring that they produce reliable results.
Dehghani emphasized the need for robust data quality frameworks to support AI applications. By implementing data quality practices throughout the data lifecycle, organizations can ensure that their AI models are trained on high-quality data, leading to better performance and more accurate predictions. For example, a financial institution can implement data quality checks to ensure that transaction data is accurate and up-to-date, improving the performance of fraud detection models.
Zhamak Dehghani‘s insights highlight the importance of adopting a proactive approach and integrating data quality measures into the early stages of the data lifecycle, so that organizations can ensure higher accuracy and relevance of their data.
Leveraging Semantic Layer For Data Quality, Privacy And Governance
Speaker: Alex Gorelik, LinkedIn
We also had Alex Gorelik from LinkedIn, talking about the importance of leveraging semantic layers for data classification to ensure high data quality and governance.
The Shift from Traditional Methods
Traditional manual, rule-based data classification methods are becoming obsolete. In the past, data classification often involved painstaking manual efforts, with rules applied at the field level. While effective in simpler, smaller datasets, this method struggles to keep up with the vast, complex datasets of modern enterprises. Semantic layers automate the classification process, enhancing efficiency and coverage. By applying rules at the data element level, semantic layers offer a more scalable and comprehensive solution.
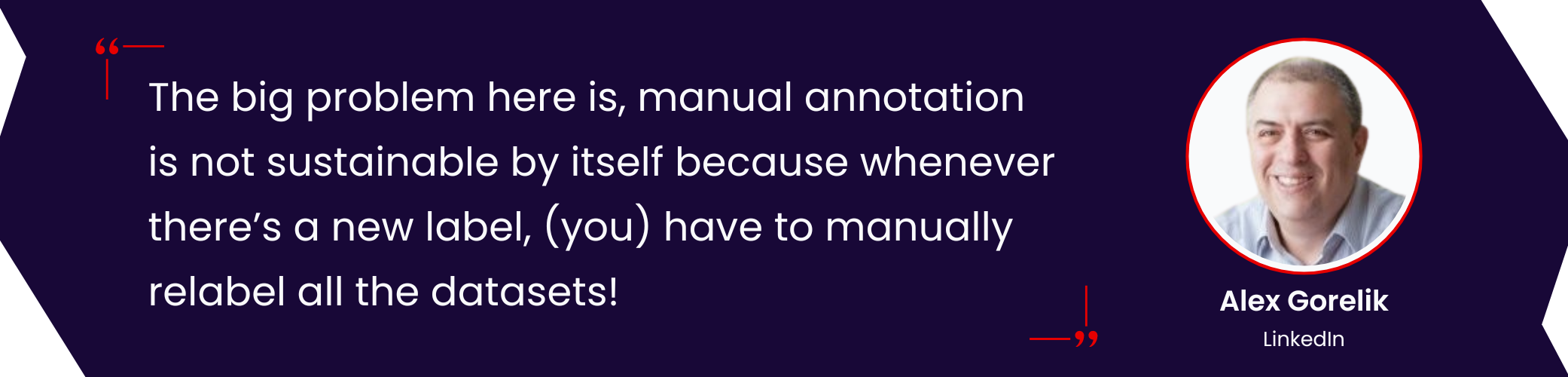
To illustrate this shift, Alex talks about LinkedIn where he works as the Distinguished Engineer on how they manage their data. With millions of users and vast amounts of data generated daily, manual classification is not feasible. Semantic layers enable LinkedIn to automate this process, ensuring data quality and governance are maintained at scale. This automation is crucial for maintaining accurate and reliable data across the platform.
Enhancing Data Quality and Governance
Semantic layers facilitate the creation of automated rules based on data labels, allowing for quicker implementation of governance policies. For example, governance policies can be applied based on specific data elements, such as targeting advertisements without violating privacy regulations. This approach significantly improves the speed and accuracy of implementing governance measures, ensuring that data usage complies with relevant laws and standards.
By leveraging semantic understanding, organizations can create more sophisticated rules that address complex data governance needs. This capability is particularly valuable in industries with stringent regulatory requirements, such as finance and healthcare. For instance, in healthcare, semantic layers can help ensure patient data is classified and governed according to HIPAA regulations, protecting patient privacy while enabling data-driven insights.
Addressing Classification Challenges
Automation is essential for handling complex data environments, capturing labels at data creation, and improving accuracy and recall. Modern data environments present numerous classification challenges, including multi-label fields, enumerations, derived fields, data obfuscation, and aggregation. Traditional methods often fall short in these scenarios, leading to inaccuracies and inefficiencies.
Automating data classification becomes essential as manual annotation is unsustainable for large datasets. Advanced suggestions and validation techniques improve accuracy and recall, ensuring high-quality data. By combining deterministic and machine learning approaches, modern data quality tools can cater to diverse use cases, from privacy protection to comprehensive data cataloging.
Field Classification
Recognizable fields, such as phone numbers and names, can be identified through formats or value sets. However, difficult to recognize fields require more sophisticated methods, such as alignment by member ID. Tools like FalDisco align reference and target fields, identifying patterns across types to enhance classification accuracy. This distinction between these fields is crucial in complex data environments.
For instance, in financial services, recognizing and classifying account numbers or transaction IDs accurately is vital for regulatory compliance and fraud detection. Automated, high-recall classification techniques are crucial for handling these complexities and ensuring data quality.
As data volumes and complexity continue to grow, semantic layers offer a scalable solution that enhances data classification, governance, and overall data quality.
Watch these sessions on demand where data experts dive into their topics with industry examples. Get free access here!
Data Quality Contracts
Speaker: Ananth Packkildurai, Data Engineering Weekly
Ananth’s session focused on the role of data quality contracts in modern data engineering, highlighting how these agreements ensure high standards in data production and consumption.
Understanding Data Quality Contracts
Data quality contracts formalize the agreement between data producers and consumers, detailing structural and behavioral aspects of data. These contracts are collaborative agreements that define two critical aspects: the structural side (schema, fields, data types, and relationships) and the behavioral side (data quality, observability, delivery frequency, and SLAs). Essentially, a data quality contract is a formalized promise from the producer to maintain certain standards, ensuring that consumers receive data that meets their needs.
For example, in a retail organization, data producers might include sales data from various channels (online, in-store, mobile), while consumers might be business analysts, marketing teams, or data scientists. A data quality contract ensures that the sales data is accurate, timely, and meets the specific needs of each consumer group.
Why Machine-Readable Formats Matter
Data contracts are typically written in machine-readable formats like Protobuf, Avro, YAML, and JSON. This is crucial because it allows computers to easily understand and process the data, facilitating automation and integration with other systems.
Using machine-readable formats ensures that data contracts can be seamlessly integrated into data pipelines and workflows. This automation reduces the risk of human error and ensures consistency in data quality across different systems and applications. For instance, in a financial institution, using machine-readable formats for data contracts ensures that transaction data is accurately processed and reported, minimizing the risk of compliance issues.
The Role of Data Ownership
Clear ownership responsibilities ensure high data quality, akin to the responsibilities of owning a house. Just as owning a house involves responsibilities and limitations, owning data comes with obligations to maintain its quality and ensure it integrates well within the larger data ecosystem. Producers are responsible for delivering structured and high-quality data, while consumers define what quality means from their perspective. This clear delineation of ownership is essential in complex data environments.
Collaborative Data Quality
In a modern data stack, a single producer often serves multiple consumers, each with different needs and quality standards. Maintaining data quality requires input from various stakeholders, including data engineers, data scientists, analysts, product managers, and ML engineers. Each role contributes to defining, maintaining, and improving data quality standards. Data contracts ensure that each team’s specific requirements are met, improving overall efficiency and data quality.
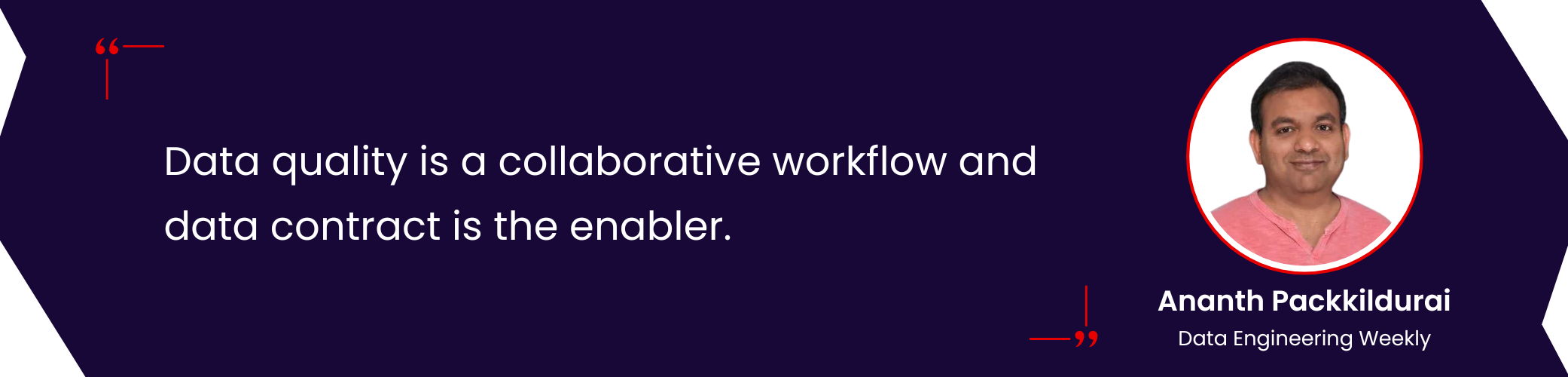
Modern Data Stacks and Data Contracts
The advent of modern data stacks has simplified data creation, accessibility, and productivity. Tools like Snowflake, DBT, and others have democratized data usage, making it easier for different roles within an organization to leverage data effectively. This evolution underscores the importance of robust data contracts to manage the complexities of data ownership and quality in a decentralized environment.
For instance, a company using Snowflake for data warehousing and DBT for data transformation can implement data contracts to ensure consistent data quality across their modern data stack. These contracts formalize expectations and responsibilities, reducing the risk of data quality issues and improving overall data governance.
In conclusion, data quality contracts are pivotal in ensuring high standards in data engineering. They formalize the responsibilities of data producers and consumers, fostering a collaborative approach to maintaining data quality. As modern data stacks continue to evolve, the role of data contracts becomes even more critical in managing the intricate relationships within the data ecosystem.
Using dbt testing? Learn how you can improve dbt testing with DQLabs here.
Why Today’s DQ Is Not Your Father’s DQ?
Speaker: Chad Sanderson, Data Quality Camp
Chad Sanderson, a well-known figure in the data community, shared his perspectives on the evolving challenges in data quality and the importance of clear ownership models. Sanderson’s influence in the industry is well recognized, particularly through his prolific social media presence, amassing over 50,000 followers on LinkedIn in a short span. His forward-thinking and sometimes provocative views have garnered significant attention.
Evolution of Data Quality
The core challenges of data quality have evolved, with the lack of clear ownership being a significant issue. Data often moves through various stages—from source systems to data engineers and analysts—without a clear sense of responsibility, leading to inconsistencies and errors. Chad emphasized that while the issues surrounding data quality have been discussed for decades, the core challenges have evolved.
In the past, data quality issues were often seen as isolated problems that could be addressed through technical solutions. However, the complexity of modern data environments has highlighted the need for a more integrated approach. Clear ownership models ensure that each stage of the data lifecycle is managed effectively, reducing the risk of quality issues.
Data Contracts as a Solution
Similar to API specifications, data contracts define the schema and expectations, ensuring clear ownership and accountability. These contracts provide a formalized structure for managing data quality, making it easier to identify and address issues. For example, in a SaaS company, data contracts can define the expected structure and quality of customer data, ensuring that all teams involved in data processing and analysis adhere to the same standards. This approach reduces the risk of errors and ensures that data is reliable and consistent.
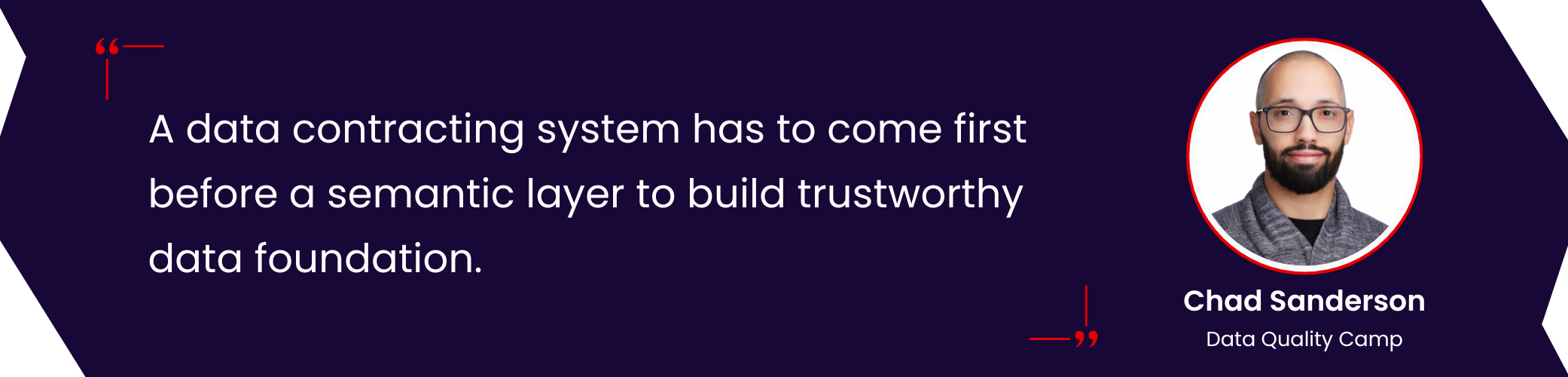
Cultural Shift
Organizations need to integrate engineering best practices into data governance to improve data quality incrementally. Chad highlighted the need for a cultural shift in how organizations approach data quality. Integrating engineering best practices into data governance processes ensures that data quality is managed proactively rather than reactively.
This shift involves adopting practices such as continuous integration and delivery, automated testing, and monitoring. By treating data quality as an integral part of the development process, organizations can improve the accuracy and reliability of their data over time.
Modern Approach to Metadata Management
Speaker: Olga Maydanchik, Metadata Architecture Expert
Olga Maydanchik discussed the critical role of metadata management in maintaining data quality and governance in modern data infrastructure. Maydanchik’s expertise in metadata architecture provided valuable insights into how organizations can enhance their data quality practices through effective metadata management.
Importance and Uses of Metadata Management
Effective metadata management supports various stages of the data lifecycle, from creation to archival. Metadata management involves capturing, storing, and using metadata to enhance the quality and governance of data. Effective metadata management supports various stages of the data lifecycle, from creation to archival.
Olga highlighted key uses such as data lineage for regulatory compliance and change impact analysis, sensitive data discovery, and integrating business concepts into metadata to enhance data analysis. She explained that metadata management is crucial throughout the data lifecycle, which includes stages like data creation, ingestion, storage, transformation, quality management, access, consumption, and eventual deletion. By capturing business, technical, and operational metadata, organizations can improve data governance and ensure data quality.
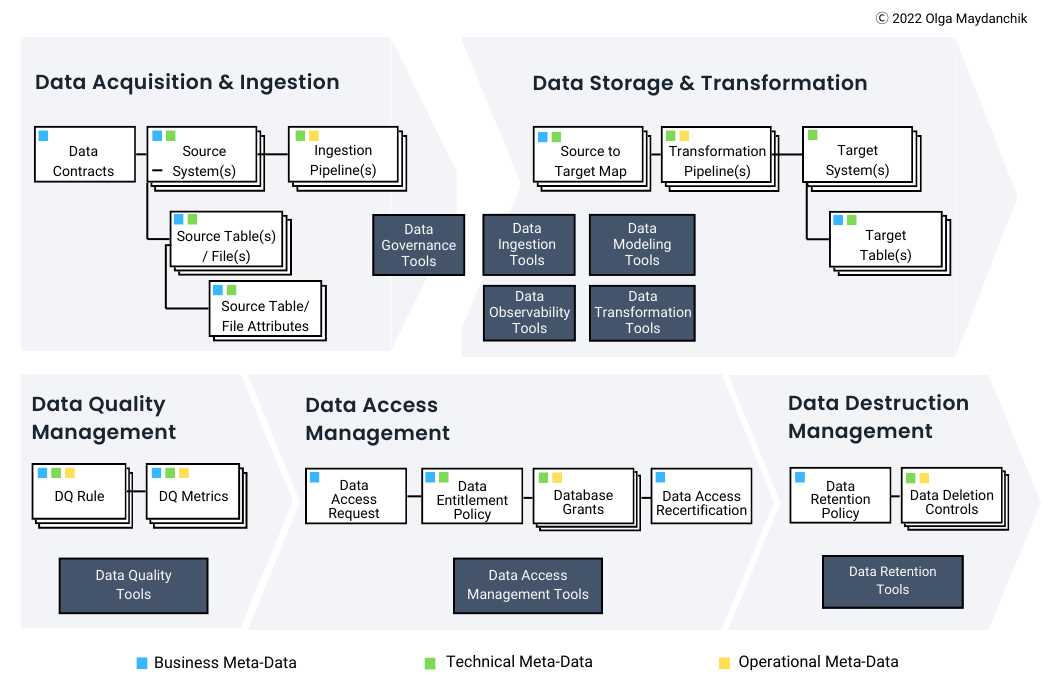
Addressing the Challenges with Metadata
Olga discussed the challenges of metadata silos and the limitations of data catalogs, which often fail to provide a complete and consistent metadata set. She presented a case study of a company transitioning to the cloud, aiming to prevent their data lake from becoming a data swamp. The company implemented data contracts, data classification tools, and a metadata repository to manage and unify metadata effectively.
The proposed architecture included automated processes to scan and classify new data, maintain a historical metadata repository, and integrate with the enterprise data catalog. This approach ensured cohesive metadata management across the data lifecycle.
Olga concluded by emphasizing the importance of including data quality metrics and alerts in metadata management. By leveraging metadata, organizations can create dynamic data quality rules and improve data governance, ultimately leading to better decision-making and efficient data management.
We’re Back This Year to Discuss AI Readiness!
The insights and innovations shared at the Modern Data Quality Summit 2023 have set a high bar for data quality and governance. As we prepare for the 2024 summit, we invite you to join us for another round of enlightening discussions, expert insights, and practical solutions on how to get your data AI-ready.
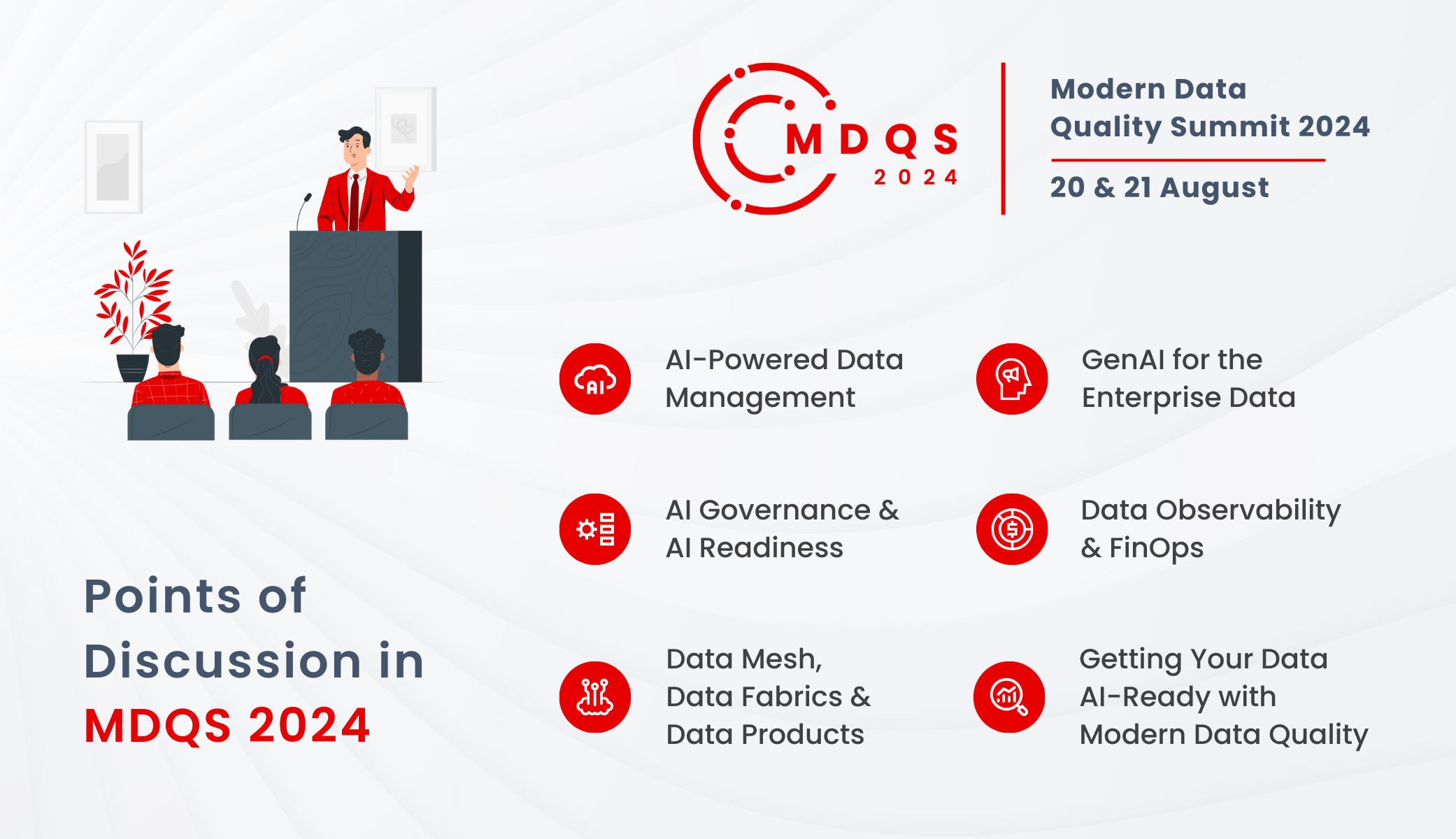
Register now for the
Modern Data Quality Summit 2024 Be a part of the future of data quality!