According to Harvard Business Review, almost half of new data records contain at least one critical error. Consequently, it is understandable that 84% of CEOs are skeptical about the accuracy of the data they use for decision-making.
With systems and data sources becoming increasingly interconnected, this interdependency often introduces new challenges. Greater complexity equates to higher risk. A minor change can result in significant downstream effects. For instance, a broken data pipeline can halt operational systems or cause executive dashboards to display incorrect KPIs, misleading top management.
While data observability has been a crucial aspect of software engineering for some time, its adoption in data stewardship is a more recent development.
Gartner defines data observability as follows,
Data observability is the ability of an organization to have a broad visibility of its data landscape and multilayer data dependencies (like data pipelines, data infrastructure, data applications) at all times with an objective to identify, control, prevent, escalate and remediate data outages rapidly within expectable SLAs. Data observability uses continuous multilayer signal collection, consolidation and analysis to achieve its goals as well as to inform and recommend better design for superior performance and better governance to match business goals.
Components of Data Observability
Data observability encompasses several key components:
Data Monitoring
Continuous tracking of data pipelines, storage, and processing systems to detect anomalies, performance issues, and data quality problems. Monitoring tools provide real-time alerts and dashboards to help data engineers and analysts maintain a healthy data ecosystem. Effective data monitoring includes:
- Real-time Alerts: Notifying stakeholders immediately when anomalies or issues are detected.
- Dashboards: Visual representations of data health metrics and system performance.
- Log Analysis: Reviewing logs to diagnose and troubleshoot problems.
Monitoring helps maintain the reliability and performance of data systems, ensuring timely and accurate data delivery.
Data Lineage
The documentation of the flow of data from its source to its destination. It includes tracking data transformations, movements, and dependencies across various systems, offering insights into data dependencies and processes. Data lineage helps in understanding the data’s journey, ensuring transparency, and aiding in troubleshooting and compliance. Key benefits include:
- Transparency: Understanding how data changes over time and across different systems.
- Impact Analysis: Identifying the downstream effects of data changes or issues.
- Compliance: Ensuring data processes meet regulatory requirements.
Data Health & Quality Checks
Assessing the accuracy, consistency, and completeness of data. These checks involve automated validation rules, anomaly detection algorithms, and regular audits to ensure that data meets the organization’s standards and is fit for use. Ensuring data health and quality is crucial for reliable decision-making. Data health and quality checks include:
- Validation Rules: Automated checks to ensure data conforms to predefined standards.
- Anomaly Detection: Algorithms to detect outliers or unexpected changes in data.
- Regular Audits: Periodic reviews of data sets to identify and correct quality issues.
Role of Semantics in Data Management
Semantics in data management refers to the meaning and context associated with data, enabling more effective data integration, interpretation, and utilization. It plays an important role in enhancing the clarity and usability of data by ensuring that data is not only syntactically correct but also semantically meaningful.
Semantics in data management involves the use of metadata to describe the meaning, relationships, and constraints of data. This includes definitions, classifications, and ontologies that provide a shared understanding of data across different systems and stakeholders. Semantics ensures that data is consistently interpreted, making it possible for diverse data sources to work together seamlessly.
How Semantics Enriches Data with Context and Meaning
Semantics enriches data by providing context and meaning, transforming raw data into valuable information. This enrichment includes:
- Contextualization: Adding context to data allows users to understand its origin, relevance, and applicability. For example, knowing that a “customer” entity includes both individuals and organizations helps in correctly interpreting customer-related data.
- Disambiguation: Clarifying meanings of terms that might be ambiguous in different contexts. For instance, the term “date” can refer to a calendar date or a social engagement; semantics clarifies the intended meaning based on context.
- Enhanced Queries: Semantic data models enable more sophisticated and accurate querying capabilities. Users can perform searches based on the meaning of data rather than just its format or structure, leading to more relevant results.
- Interoperability: It facilitates data integration and interoperability across disparate systems by ensuring that data from different sources can be understood and used together. This is especially important in large organizations and collaborative environments where data comes from varied systems.
- Data Governance: Semantics supports robust data governance by providing clear definitions and rules about data usage, thereby reducing risks associated with data misinterpretation and misuse.
- Enhanced Data Quality: By providing clear standards and meanings, semantic metadata helps in maintaining high data quality. It ensures consistency, accuracy, and reliability across the data lifecycle.
- Advanced Analytics: Semantic data empowers advanced analytics techniques like machine learning, allowing them to extract deeper insights from the data.
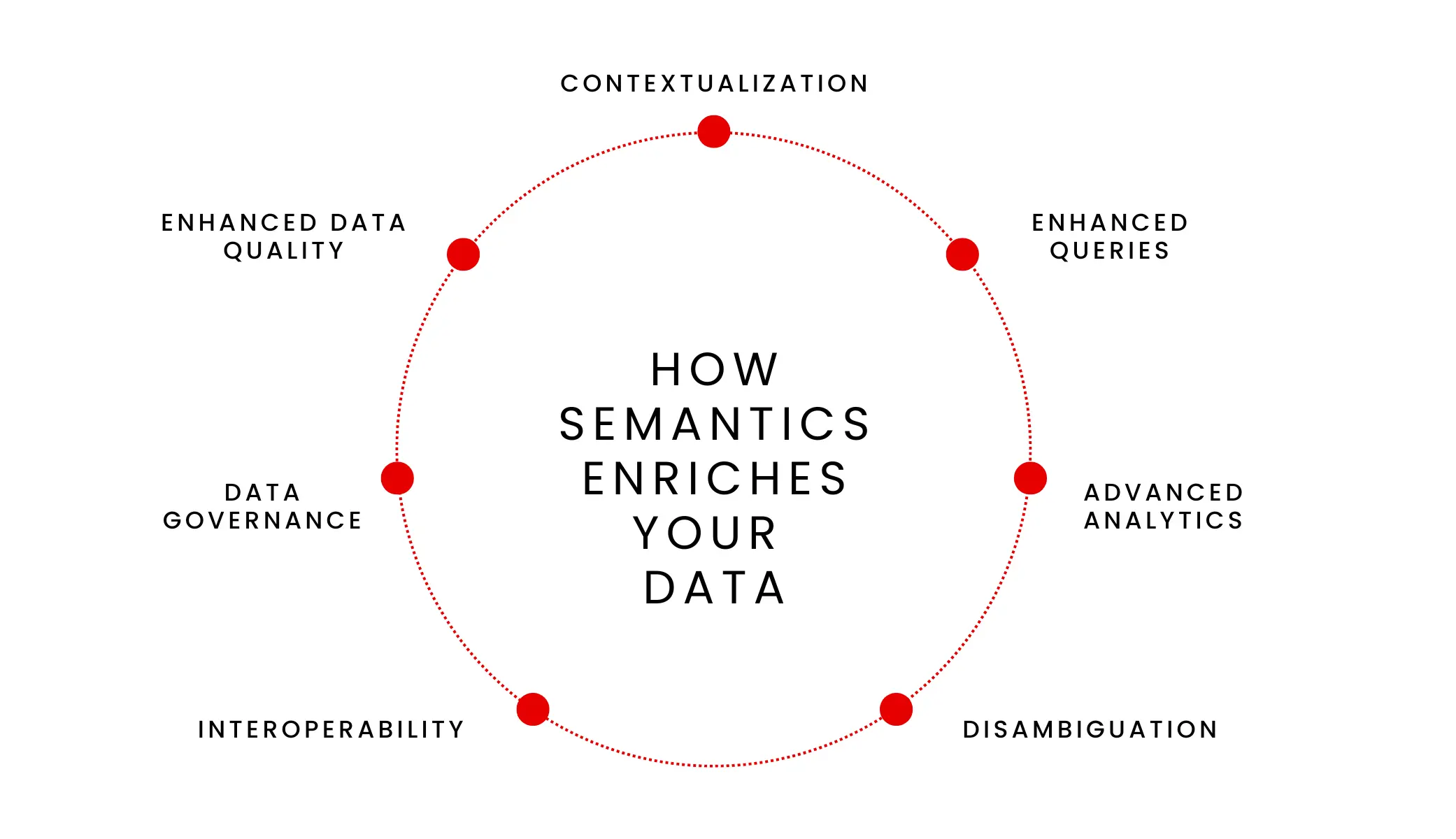
Business Benefits of a Semantic Layer
A semantic layer translates business data into familiar terms, providing a unified, comprehensive view of data across an organization. Fundamentally, it establishes a single standard for consuming and driving enterprise-wide analytics.
- Data Democratization: As data analytics becomes more widespread in organizations, relying solely on one BI or ML platform is impractical. A semantic layer platform integrates with various data platforms, protocols, and tools, decoupling data from consumption and promoting the democratization of data analytics and ML within the enterprise.
- Single Source of Truth: A semantic layer can generate complex SQL queries, resolving database loops, complex objects, and joins. By applying rules to manage database complexity, it ensures consistent results for identical queries from different users, thus providing a single version of the truth.
- Model Development and Sharing: Data scientists need raw data for insights, but businesses require processed data for decision-making. The semantic layer’s data modeling capabilities add value to raw data, enabling easy creation, sharing, and collaboration of data models and insights.
- Improved Performance and Reduced Costs: Cloud computing offers scalability and flexibility but can be costly. A firm semantic layer includes performance management systems that enhance query performance and reduce computing costs, thus facilitating quicker insights.
- Reduced Cleaning Efforts: Data cleaning is a significant part of data and analytics projects. A governance-enabled semantic layer ensures consistent data definitions, reducing the effort required for data cleaning and providing reliable insights with pre-built controls for data access, integration, and feature creation.
Benefits of Combining Semantics with Data Observability
Integrating semantics with data observability can deliver significant benefits for organizations. The combination of semantics and observability is crucial for addressing the “semantic gap” in data management.
By enriching data with contextual meaning and relationships, semantic models enable a deeper understanding of data lineage, quality, and reliability. This enhanced semantic awareness complements the visibility and monitoring capabilities of data observability tools, providing a more comprehensive view of the data ecosystem.
Semantic metadata, which describes the meaning and intent of data, helps data observability solutions identify and address semantic anomalies – issues that go beyond just broken pipelines or data quality problems. This allows organizations to truly understand if the data they are observing can be trusted and relied upon for decision-making.
Furthermore, the integration of semantics and observability empowers data teams to bridge the gap between business domains and the underlying data, encouraging better collaboration and data-driven decision-making across the organization. This holistic approach helps you maximize leverage from your data assets.
Integration of Semantics in Data Observability in DQLabs
Data observability in DQLabs uses semantics to provide a more comprehensive understanding of data across various platforms. By mapping data into familiar business terms and establishing relationships and hierarchies, DQLabs ensures that data is not only syntactically correct but also contextually meaningful. This semantic layer enables organizations to achieve a unified view of their data, facilitating better decision-making and improved analytics performance.
DQLabs, a leading provider in the field of data quality and observability, leverages the power of semantics to enhance its data management capabilities. Book a demo to know more!
DQLabs empowers users to organize data through a comprehensive set of semantic definitions. These include terms, tags, applications, dimensions, and fields. Users can assign these definitions to assets, attributes, and rules for consistent data categorization across the organization. Once defined, DQLabs automatically discovers data and field types, ensuring consistency throughout the platform. Additionally, users can define domain-specific rules to govern data behavior across the organization.
Here are the 5 ways in which DQLabs uses semantics to help with data observability:
Automated Anomaly Detection Using Semantic Rules
You can apply rules at the semantic level in DQLabs. By applying predefined semantic rules and benchmarks, DQLabs can automatically identify data points that deviate from expected behavior. These rules might include predefined conditional rules, simple query based measures or even pattern based rules using semantic terms.
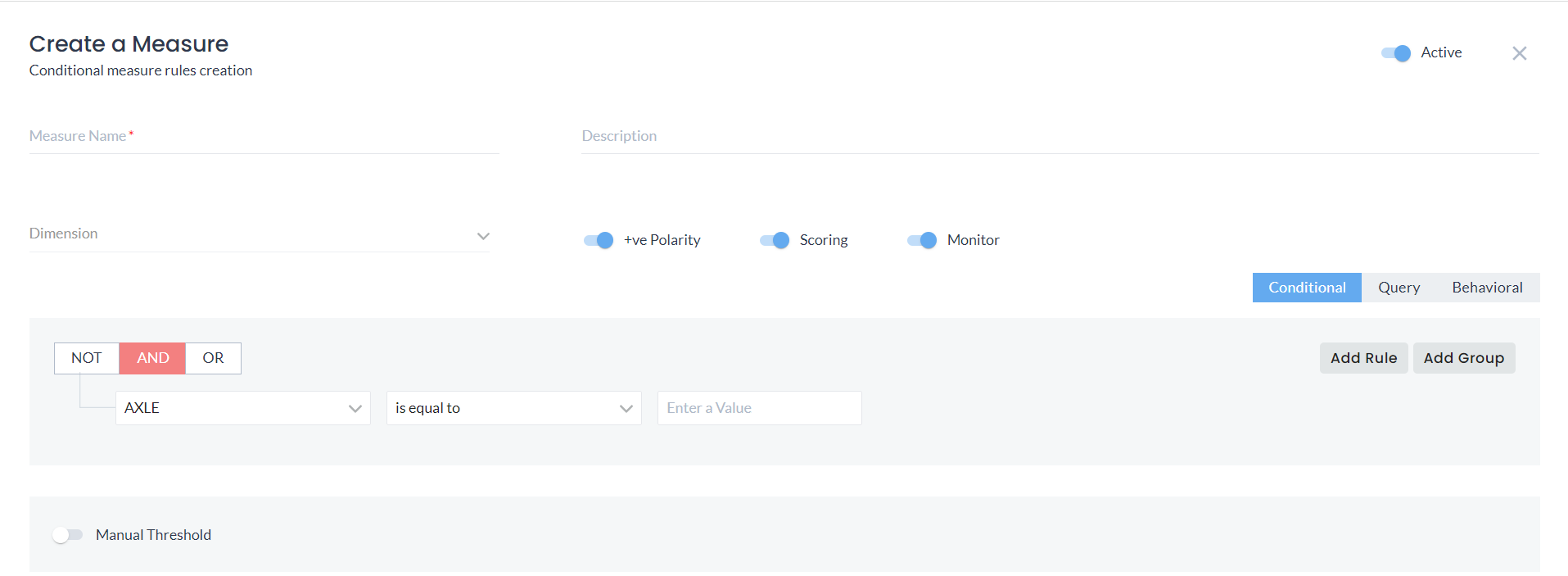
DQLabs uses semantic rules to enhance this process by identifying patterns and relationships within the data, making it easier to detect anomalies that traditional methods might miss. These rules enable DQLabs to automatically flag data points that deviate from expected patterns, allowing for timely interventions and corrections, thereby improving accuracy and reducing the effort required to address data issues.
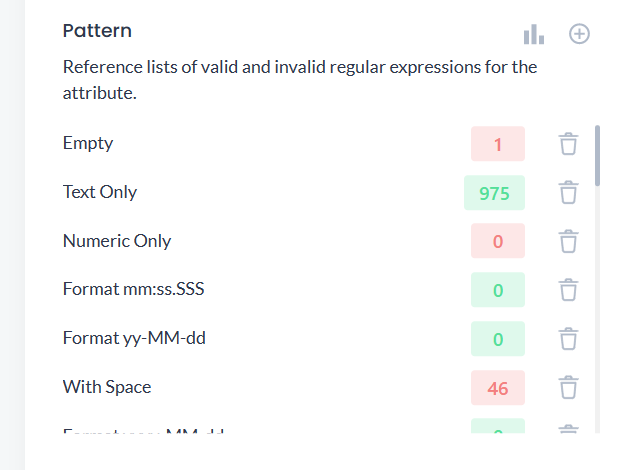
Improved Data Quality Checks Through Semantic Validation
DQLabs includes centralized data quality stewardship, enabling automatic rules discovery and profile analysis using semantics. This feature ensures data quality checks are enforced consistently across different data assets.
DQLabs uses semantic validation techniques to verify data accuracy, consistency, and completeness. By using predefined semantic rules, DQLabs can automatically validate data against business-specific criteria, ensuring that the data meets the required quality standards. This process helps in reducing errors and inconsistencies, providing more reliable data for analysis and decision-making.
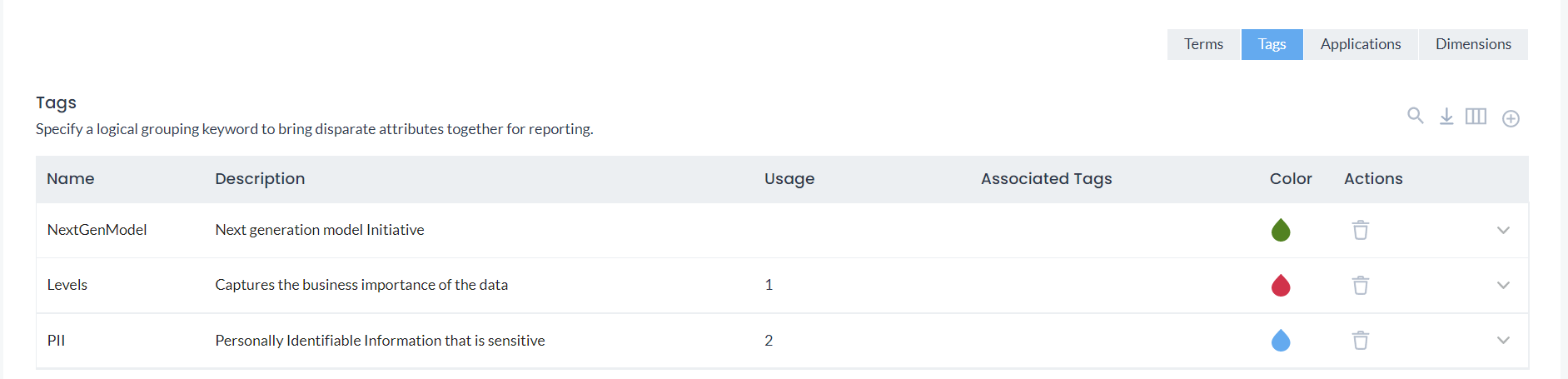
Tracking Data Flow with Semantic Annotations
Understanding data lineage is essential for maintaining data integrity and compliance. DQLabs uses semantic tags, domains, terms and applications to track the flow of data from its source to its destination. You can view DQScores based on these tags. This level of transparency is necessary for impact analysis, troubleshooting, and regulatory compliance, as it enables organizations to understand how data changes over time and across different domains and systems.
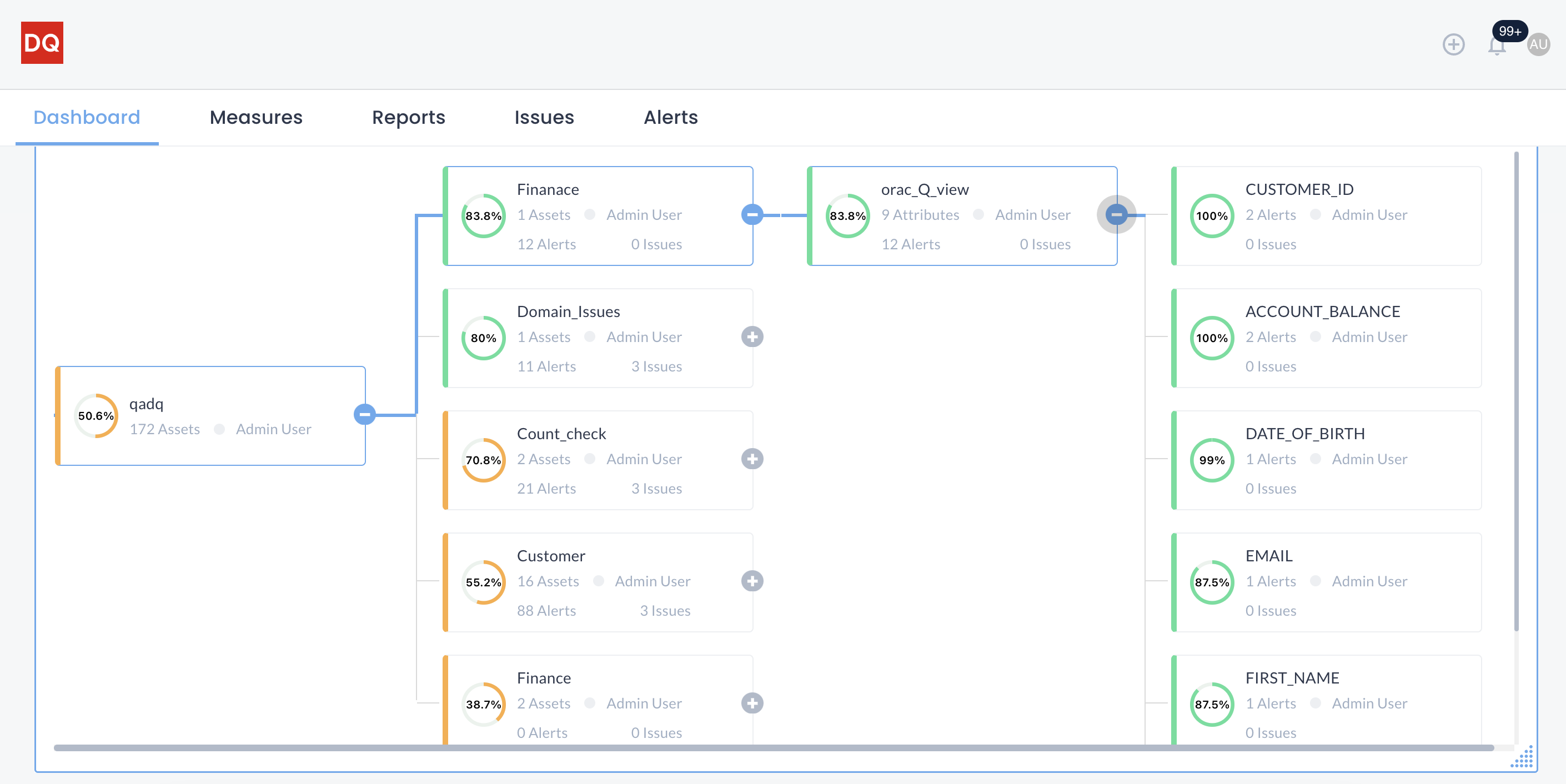
Improving Metadata Management
Effective metadata management is critical for data observability, and DQLabs enhances this by using semantic technologies to organize and describe data assets. The semantic layer enriches metadata with contextual information, facilitating easier discovery, access, and understanding of data. Automated metadata and semantic discovery with auto-tagging enable efficient handling and classification of data, improving overall data governance and usability. This approach ensures metadata is not only maintained but also enriched, making it easier to manage and retrieve across different data assets.
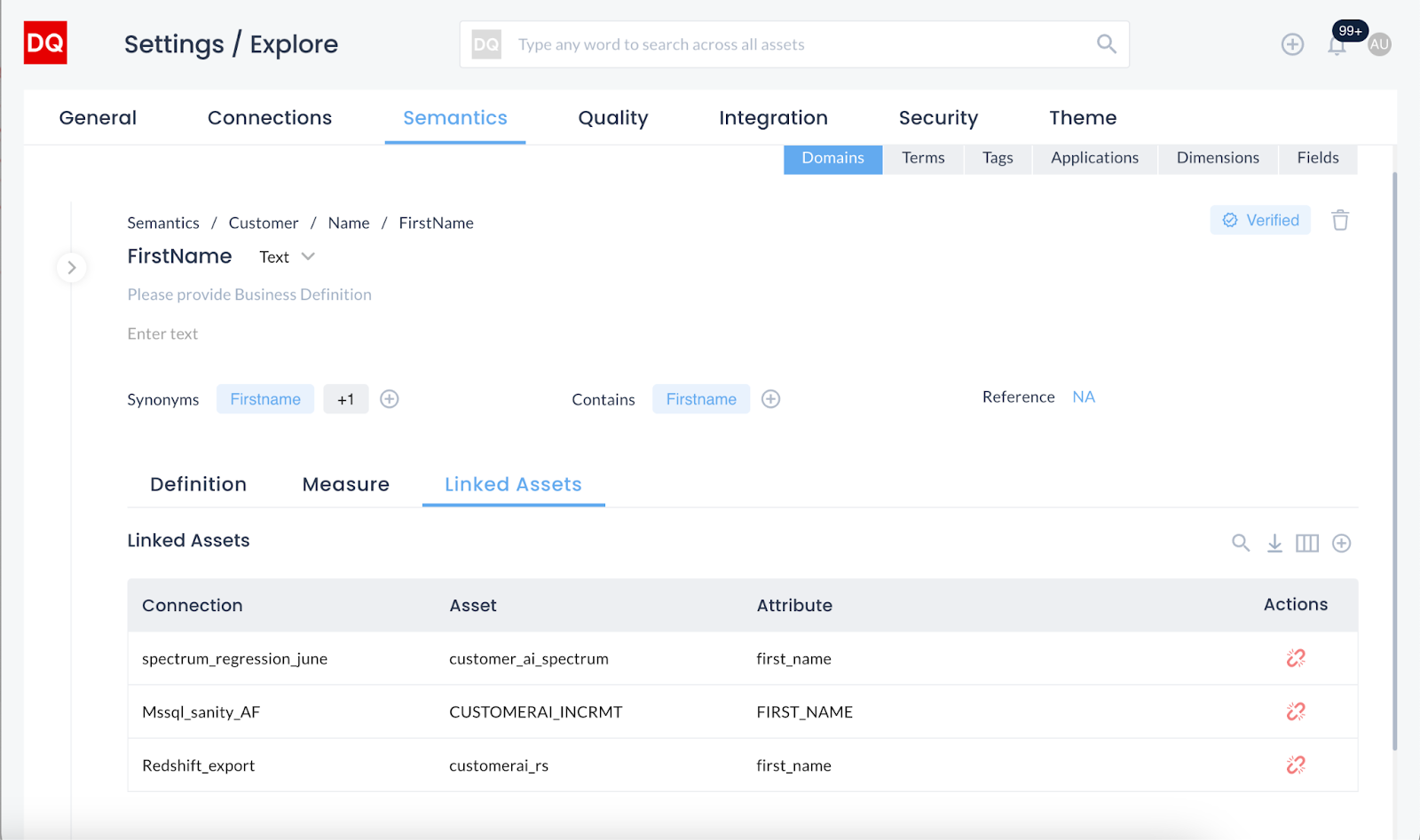
Observability Dashboards Using Semantics
The Observe dashboard provides effortless visibility into issues and alerts right out of the box. Monitor health alerts seamlessly. Designed for efficiency, the Observe dashboard lets you quickly identify the priority, severity, and duration of an issue or alert, ensuring you know exactly what requires immediate attention. With semantics, the ease of looking into your alerts is further simplified by sorting these alerts using semantic tags, terms, domains and application, so you can quickly get to action.
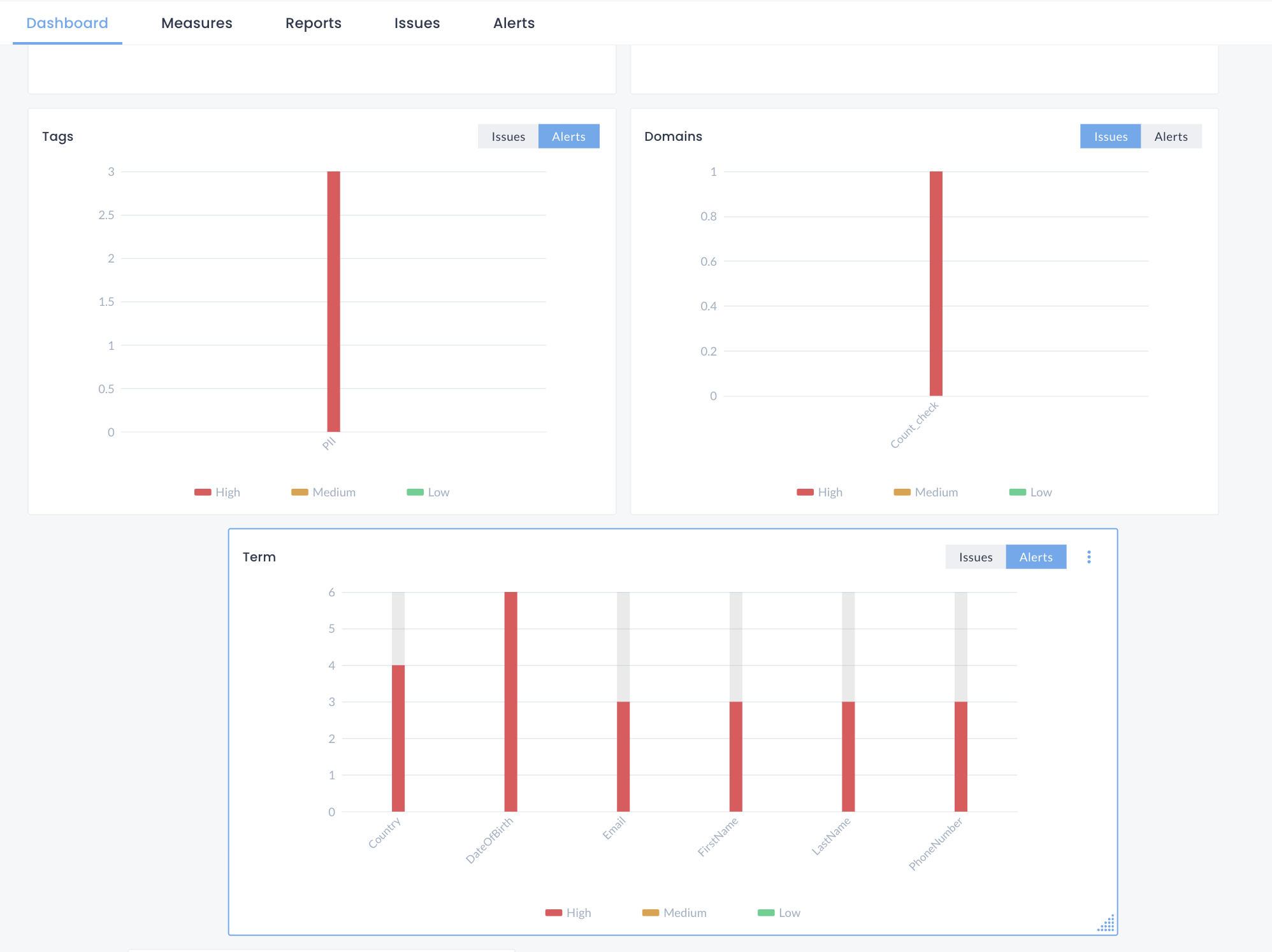
Conclusion
Incorporating semantics into data observability with DQLabs significantly enhances data management by providing deeper insights and more robust data quality measures. Automated anomaly detection, semantic validation, and detailed data flow tracking ensure that your data is accurate, reliable, and comprehensible. Additionally, improved metadata management and advanced discovery capabilities empower organizations to leverage their data effectively, driving better decision-making and innovation.
The benefits of integrating semantics into your data observability framework are clear. DQLabs’ comprehensive approach not only simplifies data management but also elevates your data strategy to new heights.