Are your data teams struggling to find the root cause of your data quality issues? Do you struggle with a lack of visibility of whether your data is maintained as per data governance and compliance standards? Does your sales team find it difficult to trust your data because of the lack of consistency in the sales dashboard? Does your data migration planning team lack projections of how changes in the schema will affect downstream data usage?
If you are struggling with any of these problems, you must understand and adopt the concept of robust data lineage practices.
A data lineage provides a visual representation of your enterprise data journey from its inception till its final consumption including different data transformations, edits, and movements. This empowers organizations to efficiently track the journey of their data assets and easily locate issues around their data systems’ downtime.
Leveraging metadata with data lineage
Data lineage provides the ability to track the entire flow of the data lifecycle from the upstream data producer to the downstream consumer, as well as the transformations that take place in the process. In the process of data transformation (i.e. making raw data consumption-ready, including, data ingestion, integration, storage, and standardization), a lot of operational metadata is being consumed. This includes ETL/ELT processes, and performance metadata, among others. Organizations with data lineage tools can track and manage this metadata, helping them to improve their understanding of data engineering processes and improve data quality and reliability.
Different types of data lineage and their usage
There are majorly three different types of data lineage – design, business, and operational data lineage.
Design lineage: Design lineage provides information about the data sources and flow of data, data creation, and data modeling during the design phase of the data systems. It provides a blueprint for the overall connectivity of data systems. For example, design lineage would provide information that the customer data is sourced from an ERP system, and structured in a Relational database, to provide support for CRM functionalities for the sales department consumption.
Operational lineage: Operational lineage provides a more granular trace of the data, by providing technical details of all data movements and transformation activities. It tracks the flow of data as it moves through different systems and various data operations in near-real time. This helps users to see how data is stored, processed, and transformed within their data environment for various purposes.
Business lineage: Business lineage provides information about the data flow for business information by filtering out technical lineage and only showing the data elements that have direct business relevance.
In addition to this, at a more granular level, there are two different types of data lineage – table level and column level:
Table-level lineage: Table-level lineage is the most basic and simplest form, providing information about how different databases or data warehouse tables relate to one another. Table-level lineage provides information about how data is sourced, transformed, and consumed.
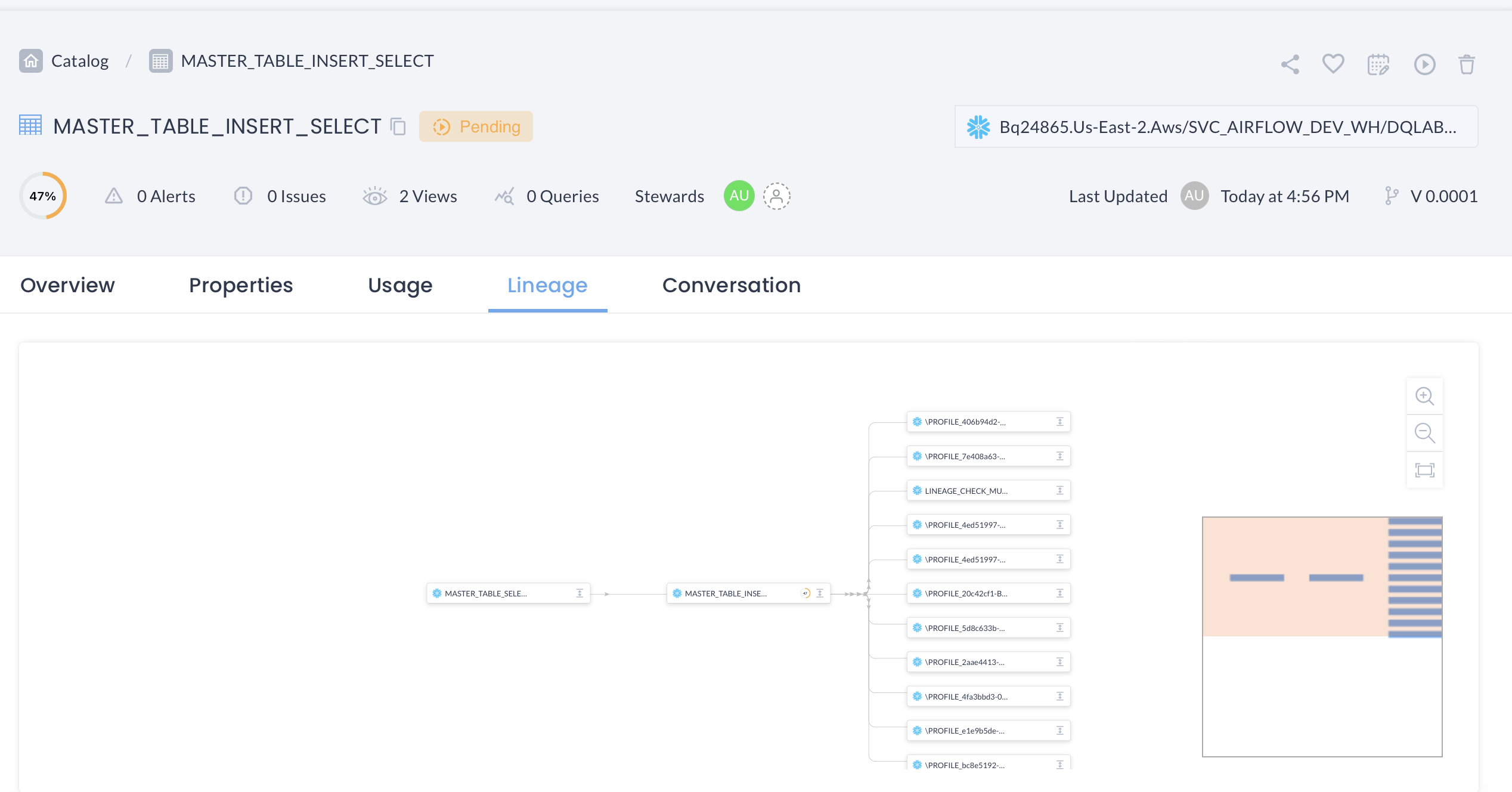
Column-level lineage: Table-level lineage does not show granular column-level information, which is why there is a need for column-level lineage. This provides more granular column-level information on how data flows, transforms, and is consumed within each specific column. Column-level lineage is important for resolving data quality issues. It empowers data engineers to track the root cause of data quality issues. It also projects the impact of schema changes on data quality, which may eventually affect downstream users.
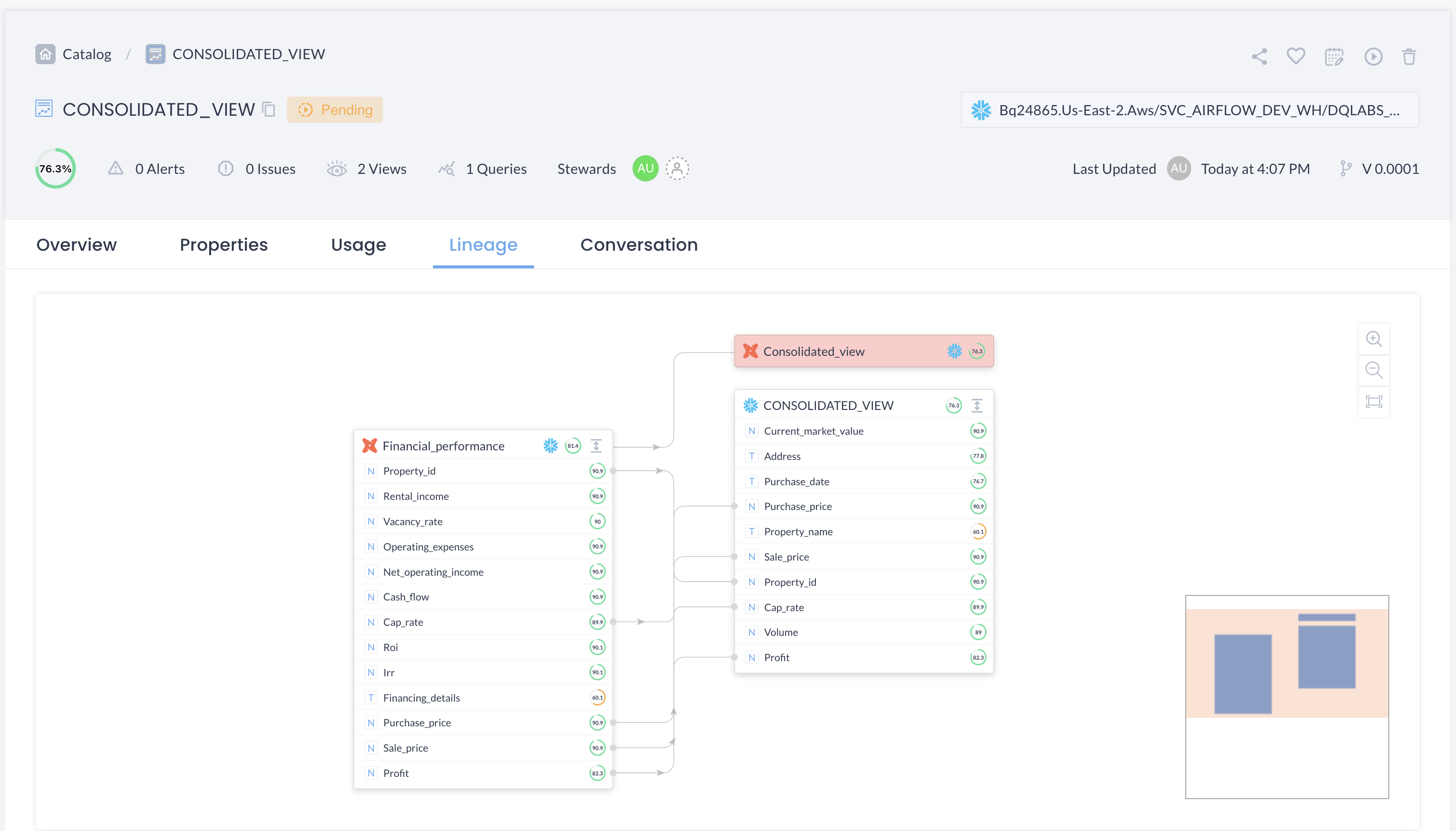
Data lineage and data quality: As we have established, data lineage empowers organizations to visually understand their data landscape. This provides a comprehensive view of important elements including, when the data was created, what transformations it went through, who are its consumers, etc. In this way, data lineage provides all the touchpoints of data and the connectivity of these touchpoints with each other, which is crucial information for the data quality supply chain.
Data quality tools provide technical data quality checks and business-level quality checks to ensure data accuracy and reliability, but without the visibility of lineage, it becomes very difficult to track data quality issues and errors. But wait, don’t allow yourself to be confused, here! Data lineage won’t solve your data quality issues, but it will guide you towards the right path to issue resolution. By looking at the data lineage, data engineers can track the journey of data, understand where data might be broken, and figure out the root cause of data downtime. Trying to track data quality without data lineage is like trying to find your way out of a dark jungle, without so much as a guiding light! It is no surprise that data lineage serves as that guiding light.
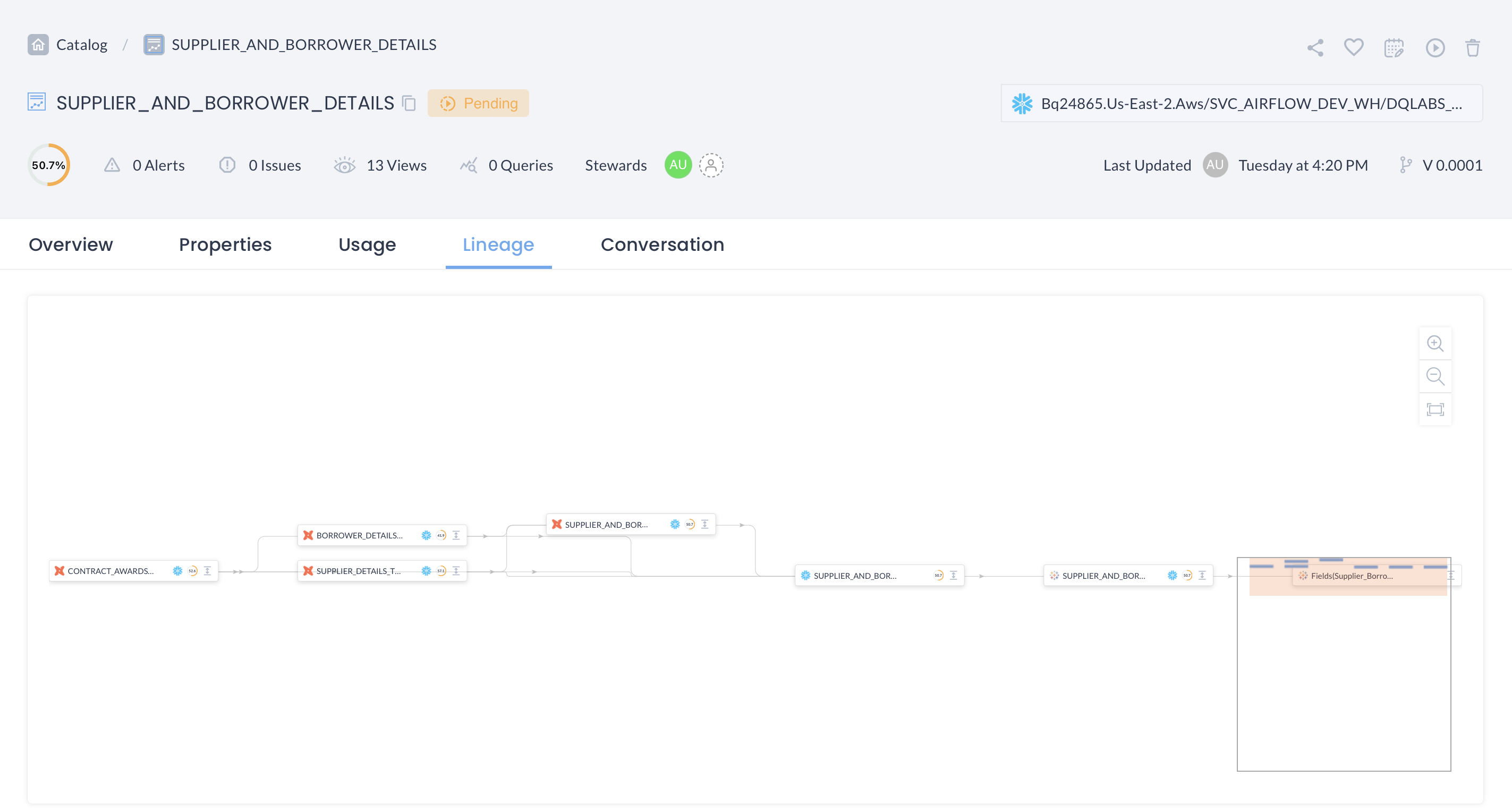
Data lineage benefits
Increased trust in data: Data lineage provides transparency and visibility of the end-to-end flow of data, from source to target data destinations. It empowers data and business users to track their data assets and helps to develop trust for data usage.
Improved data governance: Organizations need data lineage to effectively implement their data governance initiatives. Different data types need different kinds of governance policies. Policies to use PII (personally identifiable information) are significantly different from the policies governing the usage of operational data or sales data. By providing visibility into the origin and destination of each type of data, data lineage makes it easy to enable such governance initiatives. For example, if a marketing dataset (say, for email campaigning) has PII information, data stewards, with the visibility brought by data lineage, can easily flag this and take corrective action.
Enhanced data quality: Data quality and data observability tools, with their effective checks and alert mechanisms, ensure that users address data quality issues in a proactive manner. However, at times, getting to the root cause of data quality issues can be very challenging. Data lineage, by providing a visual journey of the data flow, empowers data teams to detect and remediate data quality issues. For example, when a marketing analytics dashboard malfunctions, data engineers can look at the lineage, right from the upstream source system, all the way till the data lands in the analytics dashboard. This accelerates the process of detecting where data looks broken and fixes data quality errors.
Downstream impact assessment: Some of the common causes of data downtime issues in organizations include changes in data, changes in schema, and transformations that affect the data consumption for downstream users. With data lineage, organizations can visually see how changes in the upstream data impact downstream users. This enables organizations to reduce their data downtime.
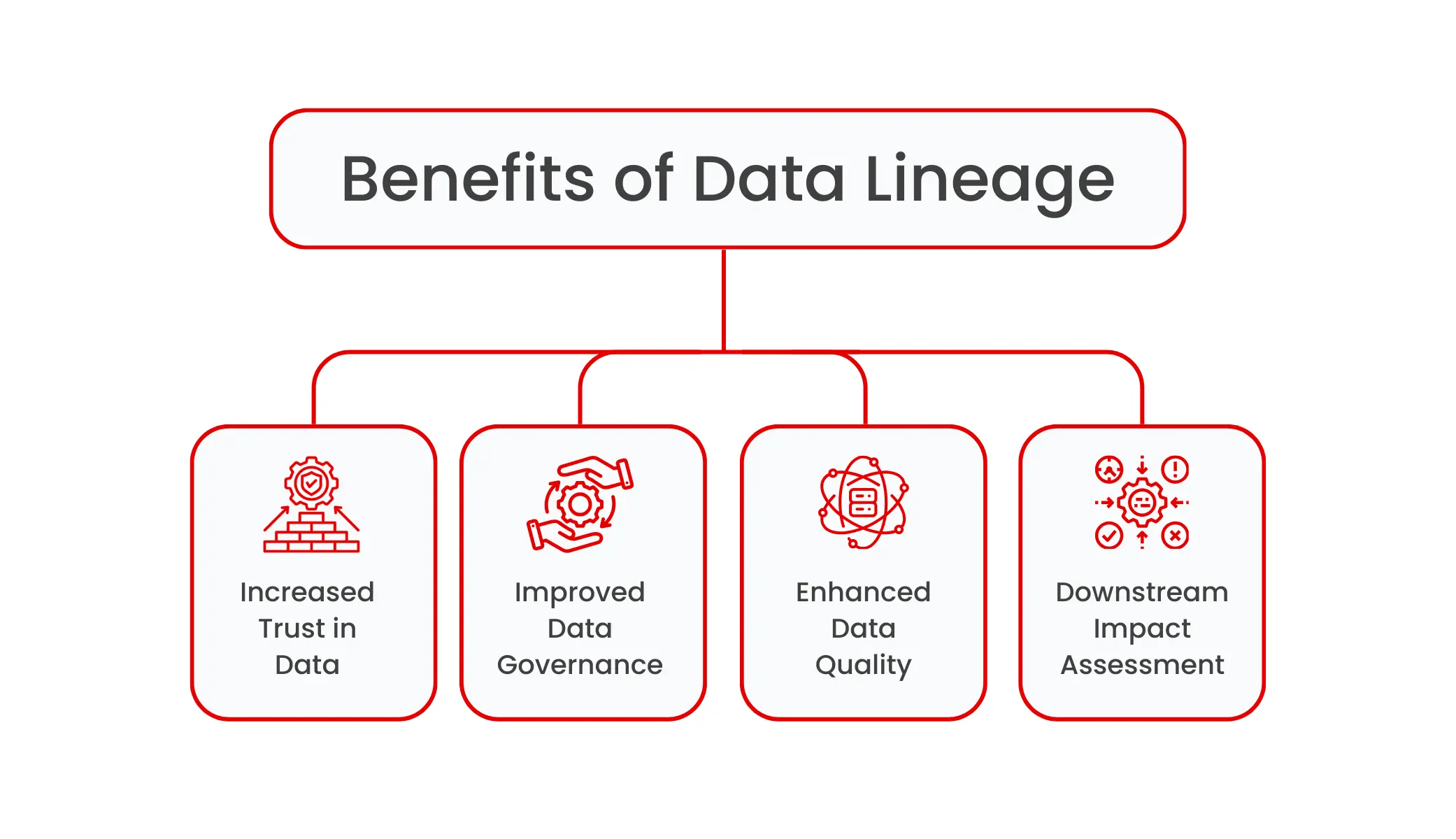
Integrations with your Modern Data Stack
To get complete benefits of your data lineage practices, you should integrate your data lineage tools with different tools from your modern data stack. A typical modern data stack includes data ingestion and integration tools (Fivetran, Streamsets, dbt), a data storage layer (AWS S3, MySQL, Oracle, Snowflake), data processing engines (Spark, Flink), and a data and consumption layer (BI and analytics dashboards). By using data lineage tools to integrate & collect metadata from your modern data stack, you will have comprehensive and complete visibility of your data landscape.
Why DQLabs
DQLabs, the Modern Data Quality Platform, empowers users with the ability to visualize the flow of data and also view the associated issues and alerts for the data. DQLabs provides data source, table, and field-level lineage to provide a comprehensive visual understanding of your data environment. By having a clear understanding of the data lineage, organizations can better manage their data assets, monitor their data quality, meet regulatory requirements, and enable informed decision-making based on trustworthy data.
Conclusion
Adopting robust data lineage practices is crucial for organizations struggling with data quality issues, governance challenges, and lack of trust in data accuracy. Data lineage provides a clear visual roadmap of data’s journey from its origin to its various destinations, encompassing transformations and ensuring transparency. This visibility not only aids in identifying root causes of data quality issues promptly but also enhances overall data governance by aligning data usage with regulatory standards.
Furthermore, data lineage also enables effective downstream impact assessment, allowing organizations to predict and mitigate disruptions caused by changes in data or schema during upstream operations. This proactive approach reduces data downtime and improves operational efficiency. Integrating data lineage tools with modern data stacks enhances this capability by providing comprehensive visibility across data pipelines and systems.
Ultimately, data lineage serves as a guiding light for data teams, enabling them to navigate complexities in data management with clarity and confidence. By leveraging data lineage effectively, organizations can optimize their data management processes, enhance data reliability, and foster greater trust in data-driven decision-making throughout the organization.