How do you know your data is reliable enough to support critical business decisions? If you’re not actively monitoring its accuracy, completeness, and consistency, it’s only a matter of time before problems surface.
High-quality data is essential—whether it’s being used in dashboards, executive reports, or AI models. Without proper monitoring, even small issues can slip through and lead to broken insights, compliance risks, and costly errors.
Neglecting data quality monitoring doesn’t just impact day-to-day operations. It erodes trust in your data systems, delays decisions, and can result in revenue loss or regulatory setbacks.
To avoid these pitfalls and ensure your data stays trustworthy, continuous data quality monitoring is essential. In this blog, we’ll dive into why it matters, the key metrics to track, and best practices for keeping your data in check.
But what exactly does data quality monitoring entail, and how does it work? Let’s break it down.
What Is Data Quality Monitoring?
Data quality monitoring is the practice of continuously checking your data for issues—like missing values, duplicates, or sudden changes—so you can catch and fix problems before they impact decisions.
It helps teams stay on top of data health with automated checks and alerts, ensuring that data stays accurate, consistent, and trustworthy as it moves through systems.
Are you confident in the quality of your data today? If not, monitoring is a good place to start.
Why Do You Need to Monitor Data Quality?
Data drives almost every decision a business makes—from understanding customer behavior to optimizing operations and building AI models. But data isn’t perfect. It can become inaccurate, outdated, or inconsistent over time. If you’re not actively monitoring it, these issues can go unnoticed, leading to significant problems that affect your bottom line, customer satisfaction, and even compliance.
The risks of poor data quality are real—and they can be expensive. Here’s why data quality monitoring is crucial:
1. Avoid Costly Errors and Financial Losses
The true cost of poor data quality often hits when it’s too late. Whether it’s inaccurate financial data leading to miscalculations, wrong product data causing shipping delays, or erroneous customer data resulting in lost sales, these errors can cost your company in direct financial losses, wasted resources, and damaged reputation. Continuous monitoring helps catch errors early, preventing them from snowballing into major issues.
2. Make Informed, Data-Driven Decisions
Bad data leads to bad decisions. If your data is flawed, your insights and strategies are too. Whether it’s the wrong market analysis, outdated financial forecasting, or inaccurate sales data, poor-quality data can send your organization in the wrong direction. By monitoring your data quality, you ensure that every decision is made based on accurate, reliable, and timely information.
3. Avoid Compliance Violations and Legal Risks
Data is increasingly under scrutiny from regulatory bodies. Non-compliance with regulations like GDPR, HIPAA, or CCPA due to poor data handling can lead to hefty fines, legal issues, and damage to your brand’s credibility. By continuously monitoring your data quality, you ensure that your data remains compliant with the strict requirements set by governing authorities, avoiding any risk of legal or financial penalties.
4. Prevent Negative Impact on Customer Experience
In today’s competitive market, customers expect smooth, personalized experiences. Bad data, such as duplicate records, outdated contact details, or missing preferences, leads to confusion, delays, and poor service. This not only frustrates customers but can also result in lost opportunities, churn, and a tarnished reputation. Data quality monitoring helps ensure that every customer interaction is based on accurate, current information, fostering trust and loyalty.
5. Support Reliable AI and Analytics
AI and machine learning models are only as good as the data that feeds them. Flawed data leads to unreliable predictions, poor recommendations, and skewed insights, which undermine the effectiveness of your models and the value they provide. Monitoring data quality ensures that your models operate on clean, structured, and reliable data, resulting in better insights, improved performance, and a greater return on your AI investments.
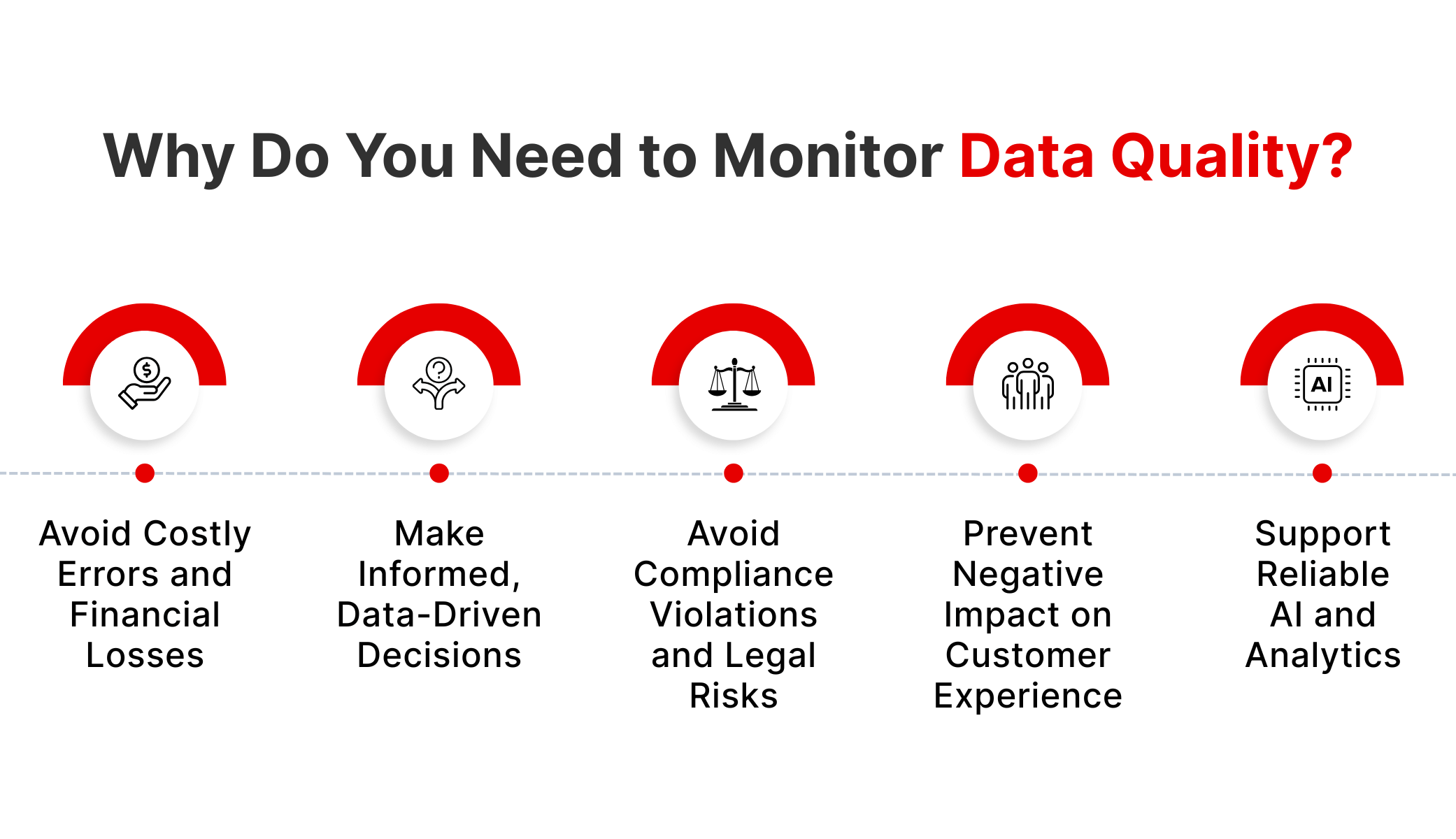
Essential Data Quality Metrics to Monitor
If you’re working with data regularly, you know it’s not always perfect. Some of it’s clean and useful. Some of it? Not so much.
That’s where data quality metrics come in. Think of them as checkpoints to make sure your data isn’t quietly derailing your decisions, your reporting, or your customer experience.
Here are the key metrics to monitor:
1. Accuracy
Accuracy means your data should correctly reflect real-world values. Inaccurate data leads to mistakes that can affect decisions and operations.
Example: A retail company sends an email promotion with an incorrect name due to a typo in their customer database. This small mistake can lead to customers unsubscribing or having a negative experience. Similarly, a shipping address error could result in delayed deliveries and customer dissatisfaction.
2. Completeness
Completeness ensures your data contains all the necessary information to make informed decisions. Missing data can cause incomplete insights and hinder operations.
Example: A bank processes a loan application but doesn’t have the customer’s full income details. This missing information delays the approval process, frustrating the customer and potentially leading to lost business.
3. Consistency
Consistency means the same data should appear across all systems, reports, and sources. Inconsistent data can create confusion and lead to errors in operations.
Example: A customer’s billing address in the CRM is different from the address in the shipping system. This inconsistency could cause orders to be sent to the wrong location, leading to costly returns and poor customer experiences.
4. Timeliness
Timeliness ensures your data is up-to-date and reflects the current situation. Outdated data can lead to ineffective decision-making.
Example: A sales dashboard updated weekly helps teams track pipeline progress and adjust strategy. If it’s outdated, teams risk missing targets based on stale insights.
5. Uniqueness
Uniqueness ensures there are no duplicate records in your system. Duplicates lead to inefficiency and confusion in reporting and decision-making.
Example: A customer is listed three times in the database with slightly different email addresses. This duplication means marketing campaigns are sent multiple times to the same person, wasting resources and potentially irritating the customer.
6. Validity
Validity ensures that data follows the correct format and adheres to predefined rules. Invalid data can cause errors in processing and analytics.
Example: A customer enters an email address incorrectly, missing the “@” symbol, which may cause the system to reject the entry or silently accept it, resulting in undelivered emails and missed opportunities.
7. Integrity
Integrity ensures that relationships between data points remain accurate and connected. Broken links between data sets can lead to incomplete or misleading insights.
Example: A hospital’s patient record lists the patient’s information but fails to link it to the doctor responsible for their care. This lack of integrity can cause confusion among medical staff, leading to mistakes in treatment.
These metrics help you maintain high-quality data, ensuring accurate decision-making, smooth operations, and positive customer experiences.
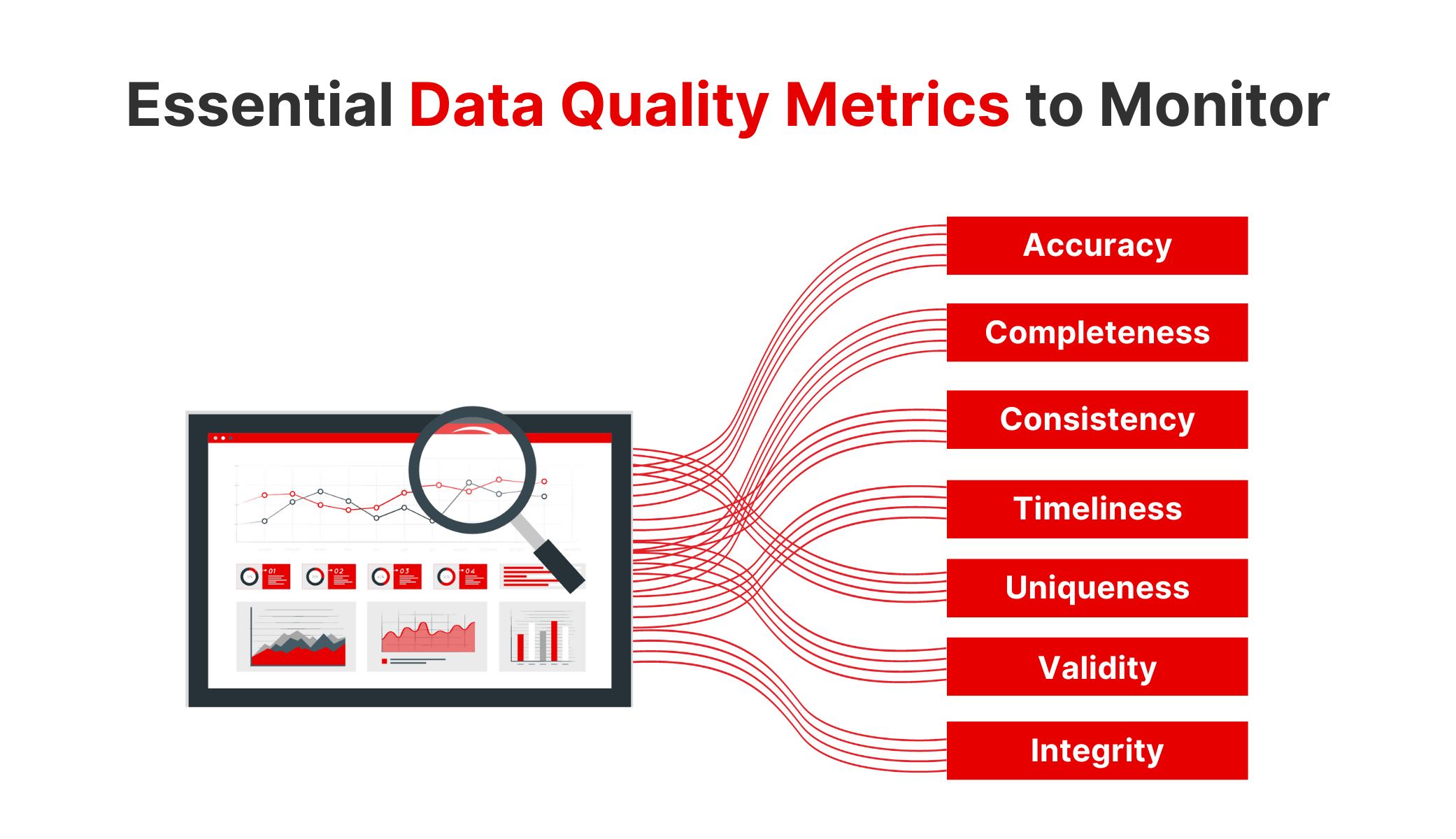
Top 10 Data Quality Monitoring Techniques
Ensuring high-quality data requires continuous monitoring using the right techniques. These techniques help detect, prevent, and resolve data quality issues before they impact business operations. Below are 10 essential data quality monitoring techniques that organizations can implement to maintain accurate, reliable, and consistent data.
1. Data Profiling
Think of data profiling as a quick scan to understand what your data looks like. Is anything missing? Are the formats all over the place? Data profiling involves analyzing datasets to assess their structure, completeness, and consistency. Data profiling is the first step of the data quality process which helps users to understand the overall structure and quality of their data.
Data Profiling helps organizations understand patterns, identify missing values, and detect anomalies that might indicate quality issues. By examining distributions, relationships, and format adherence, businesses can proactively address data problems before they escalate.
Example: A retail company profiles its customer database to identify common formatting issues, such as inconsistent date formats.
2. Data Validation Rules
Data validation rules are predefined checks that ensure data adheres to expected formats, values, and structures. These are like little gatekeepers. Users can set up these rules and ensure that the data follows certain formats or stays within certain values. These rules help catch errors such as incorrect data types, missing mandatory fields, and values outside accepted ranges. Validation can be applied at data entry points or during ETL (Extract, Transform, Load) processes to prevent bad data from flowing downstream.
Example: A banking system ensures that account numbers always have a specific length and format before processing transactions.
3. Anomaly Detection
Rule based data quality checks are essential but not scalable as we can’t configure checks to cover all possible data quality issues. Modern data quality needs anomaly detection.
Anomaly detection uses statistical methods, machine learning, or predefined thresholds to identify unusual patterns in data. It helps detect errors, fraud, and operational issues by flagging values that deviate significantly from expected norms. This technique is particularly useful in industries where data inconsistencies can lead to major financial or security risks.
Example: An e-commerce platform detects an unusual spike in transactions from a single user, flagging it as potential fraud.
4. Duplicate Detection and Deduplication
Sometimes the same record shows up more than once. That can lead to confusion and waste space. Duplicate detection helps find these repeated records.
Duplicate detection helps identify redundant records that can cause inconsistencies and inflate data storage. Deduplication involves merging or removing these duplicates while preserving critical information. This technique is essential for maintaining clean and accurate customer databases, preventing duplication of efforts, and improving analytics accuracy.
Example: A CRM system detects and removes duplicate customer profiles caused by minor variations in names and email addresses.
5. Data Lineage Tracking
Data lineage tracking maps the journey of data from its source through various transformations and systems. It provides transparency into how data changes over time, helping organizations identify errors, ensure compliance, and maintain trust in their data. This technique is critical for regulatory reporting and auditing.
Data lineage helps users to see exactly where the data came from, where it’s been, and what happened along the data transformation journey. If something looks off in your final report, data lineage helps you trace the steps back and find out where things went wrong. It’s a lifesaver when you’re trying to prove compliance or just explain how a number ended up the way it did.
Example: A healthcare provider tracks patient data from entry through multiple databases to ensure accuracy and compliance.
6. Real-Time Data Monitoring
Real-time data monitoring involves continuously scanning incoming data for errors, inconsistencies, or anomalies as it is generated. This approach ensures immediate detection and resolution of issues, reducing the risk of faulty data affecting business operations. It is especially useful for applications where timely and accurate data is crucial, such as financial trading or online transactions.
Now think of it like this: instead of waiting until the end of the day to check if something went wrong, real-time monitoring is like having someone keep an eye on your data. With real-time monitoring, you can catch bad data as it flows in, so you can act fast without wasting any time.
Example: A stock trading platform monitors price updates in real-time to prevent erroneous trades based on outdated information.
7. Metadata Management
Metadata management involves maintaining detailed information about data attributes, including definitions, formats, sources, and usage policies. Proper metadata management improves data discoverability, reduces misinterpretations, and ensures consistency across teams and systems. Organizations can use metadata catalogs to enhance data governance and compliance.
Put simply, metadata provides the instruction manual for your data. It tells you what each field means, how it’s formatted, where it comes from, and more. Super helpful for avoiding confusion. Good metadata keeps everyone on the same page, whether they’re building dashboards or auditing a report.
Example: A data governance team documents field descriptions, formats, and updates frequencies to prevent misinterpretation.
8. Data Quality Dashboards and Reports
Data quality dashboards provide a visual representation of key metrics, helping teams track data health over time. These dashboards enable quick identification of trends, anomalies, and areas needing improvement. Automated reports can also be scheduled to provide regular insights into data quality status and drive informed decision-making.
Example: A logistics company tracks delivery accuracy and timeliness through a dashboard that highlights missing or incorrect shipping details.
9. Automated Data Quality Checks
Automated data quality checks use scripts, AI-driven tools, and machine learning models to scan large datasets for common issues like missing values, duplicates, and formatting errors. Automation reduces manual effort, speeds up issue detection, and enhances overall data reliability by ensuring continuous monitoring.
Example: A financial institution runs nightly automated checks to ensure all transaction records are complete and error-free.
10. Root Cause Analysis and Issue Resolution
Root cause analysis (RCA) involves identifying the underlying reasons behind recurring data quality issues. Instead of just fixing symptoms, RCA helps organizations implement long-term solutions by tracing problems back to their source. This approach minimizes future errors and enhances overall data governance.
Example: A marketing team investigates why customer phone numbers are often incorrect and finds that a web form lacks validation checks.
By implementing these data quality monitoring techniques, organizations can proactively maintain clean, trustworthy, and high-value data for better decision-making and operational efficiency.
How to Implement Data Quality Monitoring
To keep your data accurate, consistent, and reliable, you need a structured approach to monitor its quality over time. This isn’t a one-off task; it’s an ongoing process that requires setting up the right processes, tools, and governance to track and improve data quality. Here’s how you can implement a solid data quality monitoring strategy:
- Define Your Data Quality Metrics Start by figuring out what “high-quality data” means for your organization. This can vary, but common metrics to focus on include accuracy, completeness, consistency, and timeliness. Having clear, measurable goals helps you track and improve performance over time.
- Identify Your Critical Data Assets Not all data is created equal. Focus your monitoring efforts on the data that drives business decisions or is crucial for compliance. These are the assets that matter the most, and where you should invest your monitoring efforts.
- Choose the Right Monitoring Tools Select the tools that best suit your needs. You can use built-in capabilities of your data platform, third-party solutions, or even build a custom system. The key is finding a tool that seamlessly fits into your existing tech stack and helps you track data quality effectively.
- Set Up Automated Data Quality Checks Automation is your friend here. Set up automated checks to catch errors like missing values, inconsistent data, or unusual spikes in real time. These rules will handle the heavy lifting, saving you time and reducing human error.
- Implement Strong Data Governance Policies Clear roles and responsibilities are crucial. Create guidelines on how data quality issues should be resolved and escalated. This helps ensure accountability and keeps the process smooth, especially when issues arise.
- Track Data Lineage and Metadata Understanding the full journey of your data helps you pinpoint where things go wrong. Implement data lineage tracking so you can trace issues back to their source and fix them faster, rather than playing catch-up later.
- Create Dashboards and Set Alerts Data quality dashboards give you a visual overview of trends and potential issues. Pair them with automated alerts to notify you when thresholds are breached, ensuring you can act fast before small problems turn into big ones.
- Continuously Improve with Feedback Loops Data quality isn’t a “set it and forget it” job. Business needs change, so should your monitoring strategy. Regularly review the effectiveness of your processes, gather feedback, and adjust your approach as necessary to keep your data in top shape.
By following these steps, you’ll have a continuous process in place to monitor and improve data quality, strengthening your data quality management strategy.
Choosing the Right Data Quality Monitoring Tool
Selecting the right data quality monitoring tool is essential for ensuring that your data is accurate, reliable, and ready for use. The ideal platform should offer automated monitoring, real-time alerts, and a complete view into the health of your data. It should also integrate well with your existing tech stack, scale as your data grows, and provide insights that help improve data quality over time.
But it’s not just about features. A good tool should actually make your life easier. It should reduce the need for manual checks, help you catch issues before they become problems, and give your team confidence in the data they use every day. Think of it as more than a system—it’s a partner that helps you stay on top of your data without extra effort.
As you evaluate your options, there are a few key things to keep in mind. From ease of use to the ability to surface meaningful insights, every detail counts. This is where DQLabs shines, offering a smart, scalable solution built to keep your data quality in check and your business moving forward.
Below are the key factors to consider when choosing a data quality monitoring tool—and why DQLabs stands out as the best choice.
1. Comprehensive Data Quality Coverage
A good tool should monitor multiple dimensions of data quality, including accuracy, completeness, consistency, timeliness, and validity. DQLabs provides out-of-the-box and customizable data quality checks across all six dimensions for comprehensive data quality monitoring.
2. Agentic AI-Driven Data Quality Monitoring
Today’s data environments move fast—you need a tool that keeps up. DQLabs’ Agentic AI-driven data quality and observability platform not just detects issues but learns from patterns, recommends fixes, and improves data quality over time with minimal manual effort.
3. Automated Monitoring and Anomaly Detection
Rule-based data quality checks are not scalable as they can’t cover all possible data anomalies. Organizations need a modern data quality tool that uses AI/ML-driven techniques to detect anomalies, missing data, and inconsistencies without manual intervention. DQLabs continuously tracks data health using AI-powered automation, identifying issues before they impact business decisions.
4. Seamless Integration with Data Ecosystem
The tool must integrate with databases, data warehouses, ETL pipelines, and cloud environments to monitor data at every stage. DQLabs supports integrations with leading platforms like Snowflake, Databricks, AWS, and more, making it easy to deploy across your data landscape.
5. Real-Time Alerts and Actionable Insights
Effective monitoring tools should provide instant notifications and detailed reports to help teams resolve issues proactively. Teams need modern data quality tools that don’t just flag issues but also show how serious those issues really are. DQLabs offers real-time alerts (along with their issue severity) and intuitive dashboards, ensuring that users can take immediate action and prioritize high-priority issues for effective resolution.
6. Scalability and Performance
As data volume grows, the monitoring tool should scale effortlessly while maintaining high performance. DQLabs is built to handle large-scale data environments, providing scalable monitoring without compromising speed or efficiency.
7. Data Lineage and Root Cause Analysis
Understanding the origin of data issues is essential for effective resolution. Data lineage helps users detect and investigate data quality issues by providing the complete journey of data, from the data source to the final consumption layer. DQLabs offers built-in data lineage tracking, helping teams trace errors back to their source and fix them faster.
8. User-Friendly Interface and Customization
A tool should be easy to use, with customizable rules and dashboards that fit different business needs. DQLabs provides a no-code/low-code interface, allowing both technical and non-technical users to set up monitoring workflows effortlessly.
By choosing DQLabs, businesses get an intelligent, AI-driven, and scalable solution that not only detects data quality issues but also autonomously improves data health, ensuring clean, trustworthy, and AI-ready data.
Conclusion
In today’s complex data landscape, ensuring reliable and high-quality data is more critical than ever. By implementing a structured data quality monitoring strategy—powered by key metrics, best practices, and AI-driven automation—businesses can proactively prevent errors, enhance decision-making, and drive operational efficiency. With solutions like DQLabs leveraging Agentic AI to continuously learn and optimize data quality, organizations can stay ahead of anomalies and maintain trust in their data. Investing in robust data quality monitoring is not just a best practice; it’s a competitive advantage in the AI-driven era.
FAQs
-
What is data quality monitoring?
Data quality monitoring is the ongoing process of assessing and tracking data to ensure it remains accurate, complete, consistent, and reliable. It involves detecting anomalies, identifying inconsistencies, and ensuring data integrity across various sources to support better decision-making and operational efficiency.
-
Why is data quality monitoring important?
Poor data quality can lead to incorrect business insights, compliance risks, and operational inefficiencies. Data quality monitoring helps organizations proactively detect and resolve issues like missing values, duplicates, and inconsistencies, ensuring high-quality data for analytics, reporting, and AI applications.
-
What are the key challenges in data quality monitoring?
- Data Volume & Complexity – Large-scale, multi-source data environments make monitoring difficult.
- Lack of Standardization – Inconsistent data formats and governance practices lead to errors.
- Real-Time Data Issues – Ensuring data accuracy in streaming or real-time environments is challenging.
- Manual Effort & Scalability – Traditional approaches rely on manual checks, making them inefficient.
- Root Cause Identification – Detecting and fixing the source of data issues can be complex.
-
How can businesses improve their data quality monitoring?
- Automate Data Checks – Use AI-driven tools for anomaly detection and rule-based monitoring.
- Implement Data Governance – Establish policies to standardize and enforce data quality.
- Set Clear Data Quality Metrics – Define KPIs like accuracy, completeness, and timeliness.
- Enable Real-Time Monitoring – Leverage observability platforms to detect issues instantly.
- Encourage Collaboration – Involve data engineers, analysts, and business teams in data quality initiatives.