Did you know that poor data quality can cost organizations millions of dollars every year? Bad data has become more than just a nuisance—it directly impacts your company’s bottom line. Poor data quality may lead to incorrect orders, inaccurate customer details, and misleading product information which could hurt your brand value and permanently damage relationships with your customers.
It also wastes human resources. Employees often spend up to 30% of their time fixing data issues, preventing them from focusing on their actual work. On top of that, bad data exposes companies to compliance risks. Regulations like GDPR and CCPA mandate accurate, up-to-date data—and failing to meet these requirements can result in hefty fines and reputational damage.
Beyond operations and compliance, poor data quality weakens strategic decision-making. From missing market trends to misreading customer needs, businesses without clean, accurate data risk falling behind more data-savvy competitors.
To address these challenges, organizations need a comprehensive approach to their data quality management. This is where data quality dimensions come into play. Data quality dimensions provide a structured way to evaluate the various aspects of data, such as its accuracy, completeness, and consistency.
By leveraging Data Quality Dimensions as a framework, businesses can systematically identify, address, and prevent issues that compromise their data quality. This ensures that data meets the required standards for reliable decision-making. With Data Quality Dimensions in place, teams can monitor key indicators of trustworthiness and prioritize efforts where they’re needed most.
By using this framework, organizations can identify gaps, prevent errors, and maintain data integrity across systems. In this blog, we will take you through everything you need to know about data quality dimensions.
What Are Data Quality Dimensions?
Data quality dimensions are the different ways we evaluate whether data is actually useful, reliable, and ready to support business decisions. Think of them as a checklist that helps both data teams and business users speak the same language when it comes to assessing the “health” of data.
Some examples of data quality dimensions are accuracy, completeness, and consistency, among others. Each one focuses on a specific angle—like whether values are missing, if formats are inconsistent, or if the data is outdated. Together, they help paint a complete picture of data reliability.
These dimensions are actively measured using a combination of automated checks, rules, and ongoing monitoring. That makes it easier for teams to catch issues early, before they snowball into bigger problems that affect reports, operations, or customer experiences.
In the next section, we’ll take a closer look at the six core dimensions that most teams rely on—and how each one plays a role in keeping your data quality in check.
6 Key Data Quality Dimensions
Now that we’ve covered what data quality dimensions are, let’s look at six of the most widely used ones. Each dimension focuses on a specific aspect of data health—and together, they help organizations identify where things might be going wrong and how it could affect real-world outcomes.
1. Accuracy
Accuracy refers to how correctly data represents the real-world objects or events it is intended to describe. It ensures that values stored in a system match actual facts or measurements, without distortions or errors. Data accuracy ensures that the data matches the real-world facts.
Example: Think of a patient whose blood type is mistakenly entered as B+ instead of O-. If that error goes unnoticed and a transfusion is needed, the result could be life-threatening. Inaccurate data in healthcare doesn’t just skew reports—it can directly impact patient safety.
2. Completeness
Completeness means that all required data is present and none of the necessary fields are left blank. It ensures that datasets capture the full scope of information needed for a specific task or process.
Example: A CRM system missing a customer’s email address makes it impossible to send them important updates or promotions, leading to lost sales opportunities and making it harder to deliver seamless customer experiences.
3. Consistency
Consistency refers to the uniformity of data across different systems, records, or formats. It means that the same piece of information should not vary depending on where or how it is stored. This requires standardized data practices and regular reconciliation across sources. Without consistency, data integration and reporting become error-prone and unreliable.
Example: If a customer’s shipping address is “123 Oak St” in one system and “123 Oak Street” in another, an order could be delayed or misrouted due to conflicting data, leading to customer dissatisfaction and operational inefficiencies.
4. Timeliness
Timeliness reflects whether data is current and available when it is needed for decision-making or operations. It involves minimizing delays between data creation and its use, especially in dynamic environments. Maintaining timeliness may require automated data pipelines and efficient refresh cycles. It is particularly crucial in time-sensitive domains like finance, healthcare, and logistics.
Example: A stock trading platform displaying outdated price updates could cause investors to make incorrect trades, resulting in financial losses—highlighting how delays in data availability can quickly translate into high-impact business risks.
5. Validity
Validity indicates whether data conforms to defined formats, rules, and business constraints. Data validation checks ensure that values fall within acceptable ranges or follow expected patterns, such as date formats or category codes. Validation can be enforced through data entry controls, schema definitions, and automated checks. Ensuring validity helps prevent system errors and enhances data reliability.
Example: A date of birth field should accept only valid formats like “1995-06-15.” If a user enters “35/15/2023,” it should be flagged as invalid, because invalid inputs can disrupt downstream systems, trigger errors, and reduce confidence in the data pipeline.
6. Uniqueness
Uniqueness means no duplicate records unless they’re allowed. It helps keep datasets clean and prevents confusion caused by repeated entries. Organizations often use primary keys or deduplication algorithms to enforce uniqueness. Regular monitoring is needed to prevent issues caused by accidental or system-generated duplicates.
Example: A retail store’s loyalty program mistakenly creates two accounts for the same customer, splitting their reward points and leading to confusion, which not only affects the customer experience but also distorts reporting and marketing efforts.
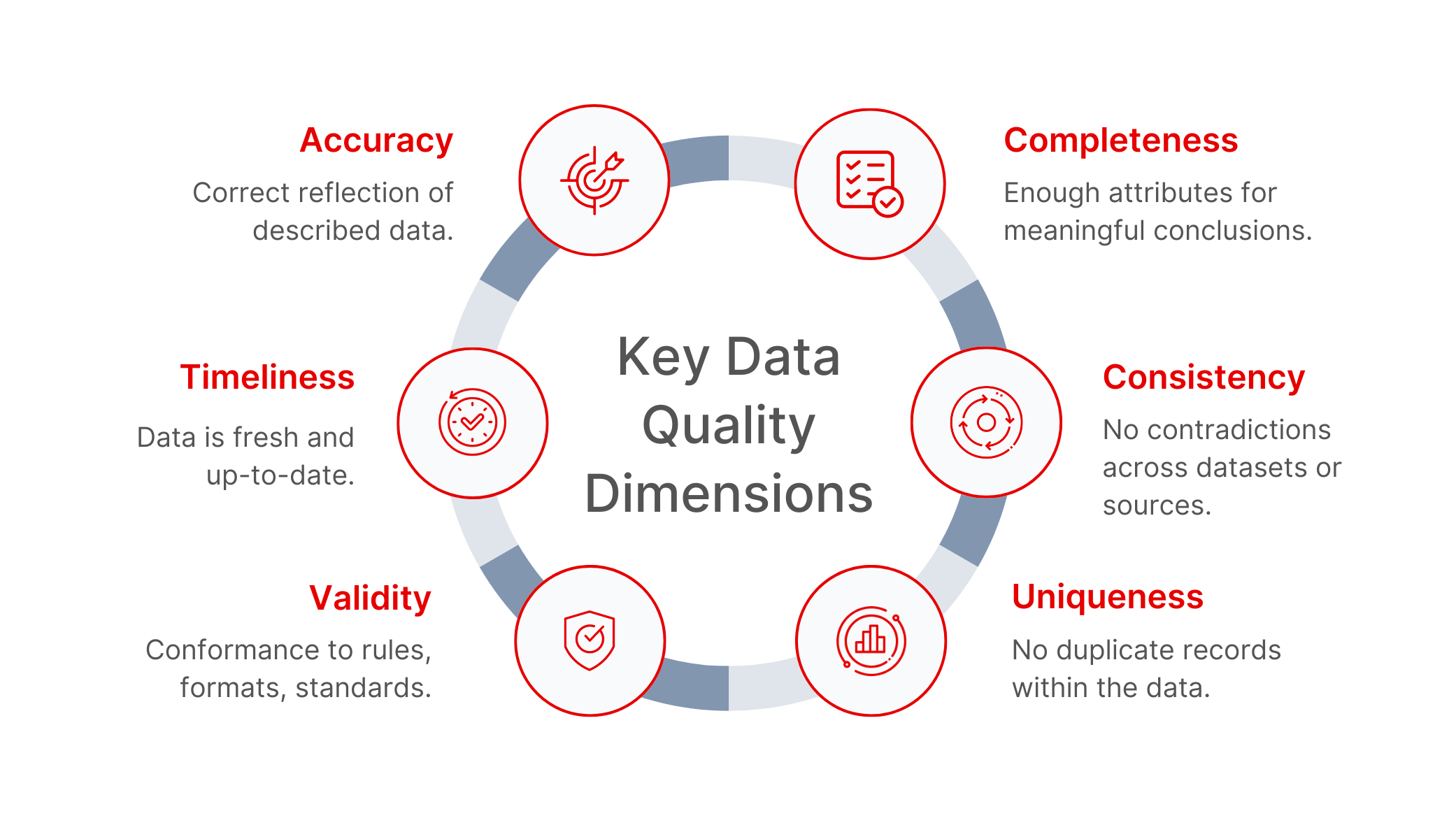
How Do You Ensure Quality and Integrity of Data?
Now that we’ve talked about why data quality matters and what dimensions help define it, let’s look at what you can actually do to make sure your data stays trustworthy, accurate, and ready to use.
Here are a few practical ways your organization can start building a solid foundation for data quality:
Establish Data Governance Policies
Develop clear guidelines that define how data is collected, stored, processed, and shared within the organization. Make sure everyone in your organization knows about these policies. Assign roles and responsibilities, making different people accountable for different parts of the data governance to make sure these policies are followed.
Provide Data Quality Training
Not everyone instinctively knows how to manage data the right way. That’s why offering short, practical training sessions on collecting data, spotting errors, and following best practices can make a big difference. When your team feels confident in handling data, they’re more likely to keep it clean and reliable.
Maintain Accurate and Up-to-Date Documentation
Keep comprehensive records of data sources, processes, and systems. Detailed documentation, including data lineage and transformation histories, helps people understand where your data is coming from, how it is being changed or processed, and who are the people involved. Having all this documented ensures transparency in data handling.
Implement Data Validation Techniques
A lot of data issues can be avoided by putting some basic checks in place. Simple validation techniques—like making sure an email address is in the right format, or that someone’s age isn’t listed as 200—help catch mistakes before they become bigger problems. These checks can be automated to flag anomalies early and reduce cleanup later.
Establish Feedback Loops
Create mechanisms for end-users to report data inaccuracies or inconsistencies. This helps users to report any mistakes they spot in the data. Encouraging open communication allows for the timely identification and correction of errors. This ensures that errors can get fixed quickly instead of sitting there messing things up.
Utilize Data Cleansing Tools
You don’t have to fix everything manually. There are plenty of tools that can automatically detect duplicates, format mismatches, or inconsistencies across datasets. Automating data cleanup can save your team time and help maintain data quality at scale.
Monitor Data Quality Metrics
Regularly assess data quality using metrics like completeness, accuracy, consistency, timeliness, and uniqueness. With Regular check-ups you can catch problems early and keep the data in good shape. By consistently measuring data quality through clear, well-defined metrics, you can track progress, spot trouble areas, and make smarter decisions to strengthen your data health. Continuous monitoring lets you detect issues early—so you can take quick action and maintain the integrity of your data over time.
Implementing these strategies can significantly enhance data quality, leading to more reliable analyses and better business outcomes.
Monitor Your Data Quality with DQLabs
DQLabs helps organizations ensure data quality by providing an AI-powered, unified platform for managing data quality. As a modern data quality solution, DQLabs is designed to continuously monitor, cleanse, and enrich your data so that it remains accurate and useful. DQLabs provides built-in and customizable data quality checks for all six key data quality dimensions, so your data isn’t just monitored—it’s measured against the standards that actually matter to your business. Here are some key ways DQLabs assists with data quality:
Automated Data Profiling: Data profiling is the first step toward understanding the quality of your data and identifying issues before they become bigger problems. DQLabs uses artificial intelligence to automatically detect anomalies, errors, and missing values in datasets. It can then help clean and standardize data using configurable rules and self-learning algorithms, reducing manual data cleanup efforts. This automation ensures your information is consistently accurate and up-to-date.
Semantic Data Discovery and Integration: The platform employs a semantics-driven engine to discover data across various sources and understand its context. By creating a smart data catalog and applying a semantic layer, DQLabs helps unify data from different systems with consistent definitions. This means everyone in your organization is working with the same high-quality data, improving consistency and trust.
Real-Time Data Monitoring (Data Observability): DQLabs provides real-time observability into your data pipelines and databases. It continuously monitors data flows for issues like anomalies, delays, or quality degradations. If a problem arises (for example, a sudden spike in missing data or an out-of-range value), DQLabs alerts your team and even initiates automated remediation. Catching issues early prevents bad data from proliferating through your operations.
Data Quality Dashboards and Insights: DQLabs offers intuitive dashboards that give you a clear view of your data quality metrics and trends. You can easily track improvements, pinpoint problem areas, and demonstrate compliance with data standards. These insights help data stewards and business users alike understand the health of the data and take action to maintain its quality.
Conclusion
Maintaining high-quality data is crucial for businesses that want to make informed decisions, streamline operations, and stay compliant with regulations. By focusing on data quality, organizations can move beyond fixing issues as they arise and instead manage their data strategically.
By addressing the six key data quality dimensions—accuracy, completeness, consistency, timeliness, validity, and uniqueness—businesses can better manage their data, minimizing costly errors. Poor data quality often leads to inefficiencies, compliance risks, and missed opportunities, making a well-structured approach to data management essential.
With AI-driven solutions like DQLabs, businesses can automate data profiling, real-time monitoring, and cleansing to maintain reliable and actionable data. Investing in a modern data quality platform not only enhances operational efficiency but also strengthens data-driven decision-making, ensuring long-term business success.
FAQs
-
How do different data quality dimensions impact business operations?
Each data quality dimension plays a crucial role in business efficiency:
- Accuracy ensures reliable decision-making by preventing errors in reporting and analytics.
- Completeness avoids disruptions by ensuring all necessary data is available.
- Consistency eliminates discrepancies across systems, reducing inefficiencies.
- Validity prevents processing errors by enforcing proper formats and rules.
- Uniqueness eliminates duplicate records, improving customer insights and operational efficiency.
Several industries rely on By maintaining high standards across these data quality dimensions, businesses can optimize processes, enhance customer satisfaction, and reduce compliance risks.
-
Which data quality dimension is the most important?
It really depends on what you’re using the data for.
- If you’re in healthcare or finance, accuracy is usually the top priority.
- For marketing and sales, completeness might matter more—you need full info to reach people or qualify leads.
- In fast-paced industries like logistics or eCommerce, timeliness is key. Old data can throw everything off.
- If your team works with multiple tools or systems, consistency and uniqueness help keep everything in sync.
So while all data quality dimensions are important, which one matters most will vary based on your use case.
-
How can organizations continuously monitor data quality dimensions?
There are a few things that help here:
- Set up automated checks to catch missing, incorrect, or duplicate data early.
- Use dashboards and alerts to monitor quality in real time, so issues don’t go unnoticed.
- Make sure teams agree on standards for what good data looks like, and apply those rules regularly.
- Encourage feedback from people working with the data—sometimes they spot problems before tools do.
A good data quality platform can make this easier by keeping an eye on key data quality dimensions in the background and letting you know when something’s off.