8 Key Data Quality Elements that Enable Real-Time Data Governance
8 Key Data Quality Elements that Enable Real-Time Data Governance https://www.dqlabs.ai/wp-content/uploads/2024/12/8-key-data-quality-elements-that-enable-real-time-data-governance-img-1024x575.png 1024 575 DQLabs DQLabs https://www.dqlabs.ai/wp-content/uploads/2024/12/8-key-data-quality-elements-that-enable-real-time-data-governance-img-1024x575.pngAccording to Gartner, by 2025, 80% of organizations aiming to scale their digital business will fail due to their inability to adopt a modern approach to data governance. Traditionally, data governance was often viewed as a reactive process, taking place after data was collected, stored, or analyzed. Governance teams would evaluate data quality, enforce rules, and ensure compliance through periodic audits and manual interventions. However, as data grew in volume, velocity, and variety, these conventional governance models began to fall short. McKinsey forecasts that global data creation will exceed 180 zettabytes by 2025. Across industries like finance, healthcare, retail, and manufacturing, data flows continuously from sources such as IoT devices, transactional systems, enterprise applications, and customer interactions. Businesses depend on accurate, timely, and actionable data to make informed decisions, enhance customer experiences, and drive innovation.
To keep up with the rapidly changing data landscape, organizations must implement robust data quality management strategies that strengthen real-time governance and ensure data integrity as it is created and updated. It must be prioritized from the moment data is created and maintained throughout its lifecycle, from producers to consumers. Without this focus, organizations cannot successfully implement or sustain a real-time governance framework.
The Role of Data Quality Management in Real-Time Data Governance
Data quality management plays a critical role in enabling effective real-time data governance by ensuring that data remains accurate, consistent, and reliable as it is continuously collected, processed, and analyzed. Gartner reveals that poor data quality drains businesses of an average of $12.9 million yearly. To address this, organizations should implement real-time data governance. This comprehensive framework ensures the effective and secure management of data in real-time environments, facilitating rapid and precise decision-making. Below are 8 key elements that illustrate how data quality management empowers this governance:
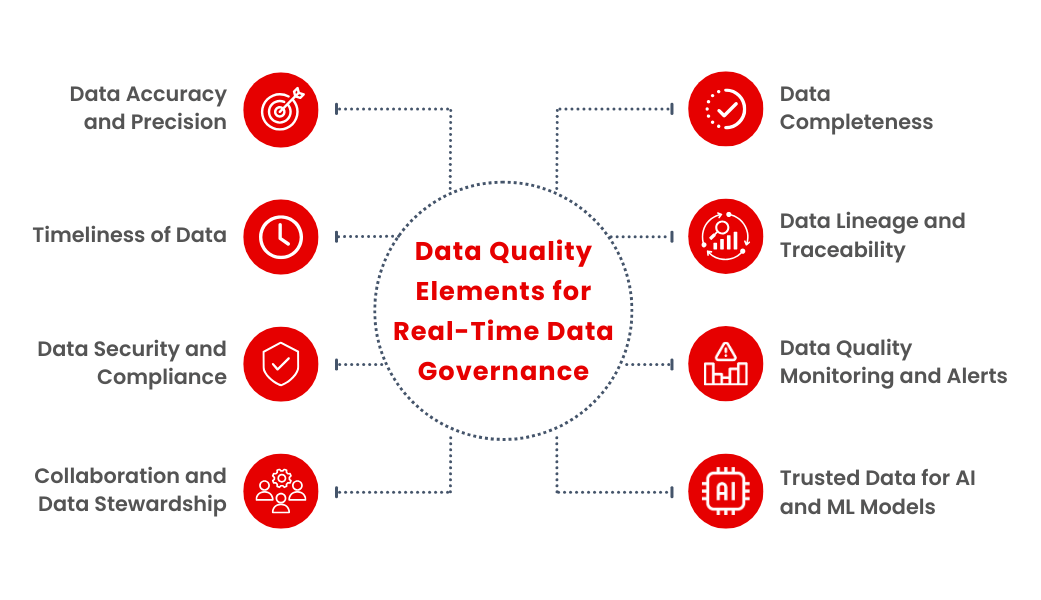
1. Data Accuracy and Precision
Real-time data governance demands high levels of data accuracy because decisions are made based on the most up-to-date information available. A recent example is the Equifax incident, where erroneous credit scores were sent to lenders, leading to higher interest rates and loan denials. Data quality management ensures that the data entering the system is accurate, reducing the risk of errors in decision-making or compliance issues. The processes involve continuous data validation, real-time data profiling, and error detection protocols, ensuring that incoming data in real-time is checked for accuracy at the point of entry. This is crucial for preventing faulty data from compromising real-time analytics or operational processes.
2. Data Completeness
Missing or incomplete data can hinder effective governance, particularly in real-time environments where decisions need to be made promptly and based on full information. Notably, 25-30% of data becomes inaccurate annually, compromising the efficiency of sales and marketing campaigns. Ensuring completeness in real-time data streams is vital for accurate oversight. Data quality management tools help identify and address gaps in real-time data feeds, flagging missing values or incomplete records. This ensures that the data used for real-time decision-making is comprehensive and reliable, supporting governance frameworks that require full datasets for regulatory compliance or operational efficiency.
3. Timeliness of Data
Real-time data governance is inherently time-sensitive, requiring data to be available as soon as it is generated. Delays or lags in data delivery can result in outdated or irrelevant insights, undermining the effectiveness of governance practices. Data quality management ensures that data is processed and made available in real time by establishing efficient workflows, data pipelines, and real-time monitoring systems. Timely data is critical for compliance, risk management, and operational governance, enabling organizations to take immediate actions when necessary.
4. Data Lineage and Traceability
Real-time data governance involves tracking how data flows and evolves through the system to ensure it adheres to policies and regulations. Without proper data lineage, it can be difficult to understand where data originated, how it was processed, and whether it meets governance standards. Data quality management incorporates data lineage tools that track and document the flow of data across systems, providing clear traceability from source to destination. This transparency is crucial for real-time data governance, as it enables auditors, analysts, and stakeholders to verify data quality, maintain compliance, and understand the impact of changes to data in real time.
5. Data Security and Compliance
In real-time environments, ensuring data security and regulatory compliance is more complex because data is constantly in motion. Real-time governance must maintain high standards of privacy, protection, and adherence to industry regulations. Data quality management ensures that data security policies, such as encryption, masking, and access controls, are integrated into the data flow. It also ensures that real-time data adheres to regulatory requirements by continuously monitoring for compliance-related issues. By embedding security and compliance checks within the data quality management process, organizations can maintain governance standards without interrupting real-time operations.
6. Data Quality Monitoring and Alerts
Real-time data environments require continuous monitoring to detect data quality issues before they impact decision-making or operations. Without proactive monitoring, data problems may go unnoticed until they cause significant issues. Data quality management includes real-time data quality monitoring tools that can detect anomalies, errors, or inconsistencies as soon as they occur. These tools often come with automated alerts, allowing data stewards or governance teams to address issues immediately, preventing potential disruptions to business processes or governance failures.
7. Collaboration and Data Stewardship
Data governance relies on clear ownership, accountability, and collaboration across departments to ensure data quality and compliance. In real-time environments, effective communication and collaboration are essential to address data issues quickly. Data quality management fosters collaboration between data owners, stewards, and governance teams by providing a framework for shared responsibilities. By defining roles and workflows for data quality, data quality management ensures that all stakeholders are aligned and can address real-time data issues effectively.
8. Trusted Data for AI and ML Models
Data governance must evolve as artificial intelligence (AI) and machine learning (ML) continue to play a significant role in modern business operations. Data quality becomes even more critical. AI and ML models rely on high-quality, accurate, and consistent data to produce meaningful insights and predictions. A recent survey found that models trained on inaccurate, incomplete and low-quality data caused misinformed business decisions that impacted an organization’s global annual revenue by 6%, or $406 million on average. By implementing effective data quality management tools, organizations can ensure that the data fed into these models is clean and trustworthy. This, in turn, improves the effectiveness of AI and ML initiatives, allowing businesses to gain more accurate predictions and better outcomes from their advanced analytics.
Next Steps to Achieving Data Quality for Effective Real-Time Data Governance
As organizations continue to embrace digital transformation, real-time data governance will play an increasingly important role in maintaining compliance, mitigating risk, and making data-driven decisions. But to make real-time data governance truly effective, data quality management is a necessity.
By ensuring data is accurate, consistent, complete, secure, and timely, data quality management provides the foundation upon which real-time governance can thrive. Without robust data quality practices, governance efforts are at risk of being undermined by faulty or incomplete data, leading to poor decisions, missed opportunities, and compliance failures.
In short, organizations that invest in data quality management solutions as part of their real-time governance model are better equipped to navigate today’s data-driven world, achieving both operational efficiency and strategic success.