Enhance Data Trust and Reliability with Data Quality Dashboards
Enhance Data Trust and Reliability with Data Quality Dashboards https://www.dqlabs.ai/wp-content/uploads/2024/12/enhance-data-trust-and-reliability-with-data-quality-dashboards.png 1024 575 DQLabs DQLabs https://www.dqlabs.ai/wp-content/uploads/2024/12/enhance-data-trust-and-reliability-with-data-quality-dashboards.pngWhat went wrong? Despite your preparation, the root cause is glaringly simple yet often overlooked—the lack of visibility into your data’s quality. How can you avoid this? The answer lies in leveraging Data Quality Dashboards!
A data quality dashboard provides real-time insights into the health of your data landscape. Without data quality dashboards it becomes nearly impossible to keep track of your organization’s data health. By providing a centralized view of your data health, data quality dashboards empower organizations to enable data-driven decision-making. Let’s explore in detail the importance of data quality dashboards in the modern data era.
The Need for Data Quality Dashboards
Data is continuously growing in volume and significance, and ensuring data quality has become one of the top priorities of organizations to enable data-driven decision making and create a competitive advantage. This scenario increases the importance of tracking data quality efficiently which is possible through data quality dashboards.
Following are the reasons why organizations need data quality dashboards:
1. Ensuring data quality at scale
Data is increasingly growing in volume, variety, and velocity. It’s impossible for organizations to manually track data quality of their data landscape. A data quality dashboard provides an efficient way to track data health metrics across various data dimensions like accuracy, completeness, uniqueness and freshness. Data quality dashboards also provide insights about the alerts and issues of datasets that help organizations to monitor and efficiently resolve data quality issues.
2. AI/ML initiatives
The adoption rate of AI/ML in business operations is continuously growing. One of the key success factors of these AI/ML initiatives is the quality of data being fed to these models. Only with high quality data that is accurate, complete, and timely, AI/ML models can generate reliable outputs. This makes it very important for any organization that successfully wants to drive AI/ML initiatives to utilize data quality dashboards.
3. Avoid the financial impact of poor data quality
Organizations are slowly realizing the negative impacts of poor data quality. According to Gartner “every year, poor data quality costs organizations an average $12.9 million.” Poor data quality leads to incorrect decision making, operational inefficiencies, and can damage the reputation of an organization. Organizations can easily assess and monitor the quality of their data landscape with DQ dashboards and avoid the impacts of poor data quality.
Requirements from a Good Data Quality Dashboard
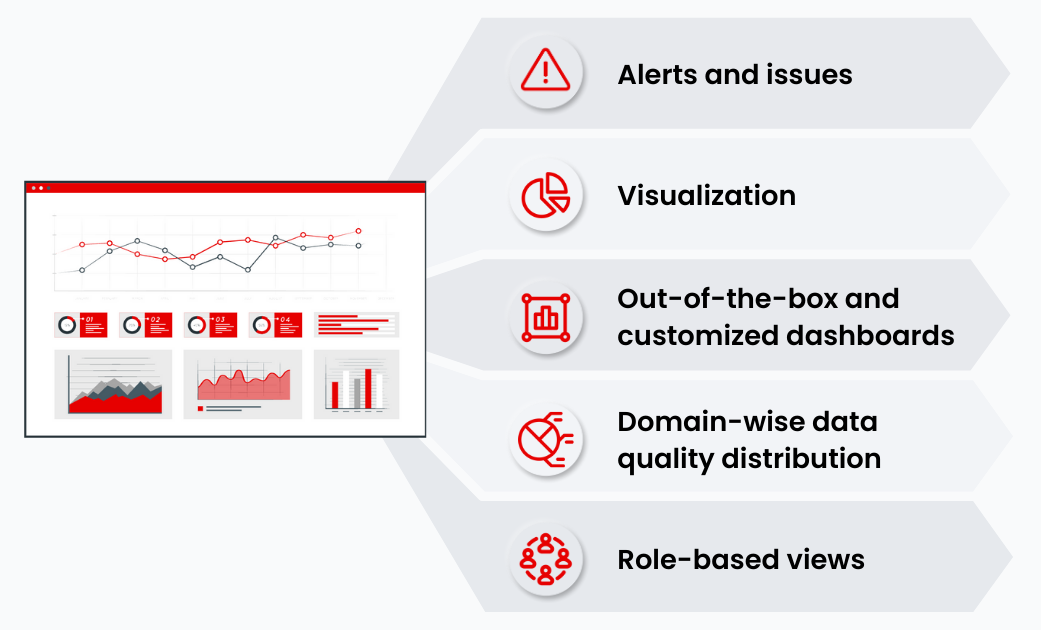
1. Alerts and issues
Data quality dashboards should provide visibility into alerts and data quality issues, ensuring that organizations can monitor their data health. Alerts can arise across multiple domains, such as customer data, financial data, or operational data, and represent potential issues like missing values, inconsistent formats, or schema changes. By centralizing these alerts, dashboards allow teams to identify trends and patterns of data issues, enabling early intervention before issues escalate.
Moreover, effective dashboards prioritize alerts based on severity and potential impact, helping users focus on the most critical data issues. This capability of alerts prioritization reduces organizational level alert fatigue and reduces data downtime.
2. Visualization
Different modes of visualization is a critical component of any good data quality dashboard, as it makes complex data easier to understand. Dashboards should offer visual representations like charts, graphs, and heatmaps that allow users to quickly assess the health of their data assets. These visuals help users identify trends, outliers, or anomalies without having to dig through raw data, saving time and effort.
In addition to this organizations need different data dashboards, tailored to the specific needs of data and business personas. For instance, a data steward might prefer detailed visualizations of missing values, while a data engineer may prioritize schema change tracking. This flexibility ensures that users can extract meaningful insights tailored to their goals and responsibilities.
3. Out-of-the-box and customized dashboards
A good data quality platform should offer out-of-the-box dashboards designed to provide instant insights into data health. These pre-configured dashboards are built around common use cases, such as monitoring data quality dimensions like accuracy, completeness, and timeliness, and allow organizations to get started quickly without extensive setup or resources requirements.
For more advanced needs, the ability to create customized dashboards is equally important. Custom dashboards enable users to focus on specific datasets, rules, or dimensions that align with their unique business requirements. This combination of ready-to-use templates and flexibility for customization ensures that organizations can address both immediate and long-term data quality challenges effectively.
4. Domain-wise data quality distribution
Domain-specific data quality metrics are essential for a comprehensive understanding of organizational data health. Dashboards should provide granular views of data quality across key domains like customer data, financial data, and sales and marketing data. This allows teams to easily discover issues that may impact specific business functions, enabling faster resolution and better decision-making.
By providing domain specific data quality metrics, dashboards empower organizations to prioritize improvements where they matter most. For instance, improving customer data quality can directly enhance personalization efforts, while resolving issues in financial data ensures compliance and accurate reporting. Such granularity builds confidence in the reliability of data across all departments.
5. Role-based views
Good data quality dashboards cater to the unique needs of different user personas such as data leaders, engineers, analysts, and stewards. Data leaders may need high-level metrics and trends for strategic decisions, while engineers benefit from insights into pipeline performance, alerts and issues tracking, and schema changes. Tailored dashboards ensure all user personas get actionable insights relevant to their role.
By offering persona-specific dashboards, organizations empower teams to focus on what matters most to their responsibilities. Analysts and scientists can use dashboards to spot anomalies and trends, while stewards gain granular views of data quality issues like missing or invalid data. This approach improves efficiency and drives collaboration across teams.
Business Benefits of a Good Data Quality Dashboard
1. Informed decision making
High-quality data, not just data, is the foundation of sound data-driven decision-making. Data quality dashboards provide organizations with real-time insights into the health of their data assets, ensuring that decision-makers have access to reliable and accurate information. This visibility helps organizations to trust their data landscape and reduces the risk of making strategic choices on flawed data.
Furthermore, these dashboards enable organizations to proactively identify and address data issues before they impact critical decisions. This reduces the organizational data downtime and minimizes disruptions to data and business workflows. By continuously monitoring and improving data quality, businesses can build confidence in their data and make more informed, data-driven decisions that drive better outcomes.
2. Centralized view of data quality
Data quality dashboards act as a centralized hub for monitoring the health of organizational data. They consolidate key metrics, alerts, and insights into a single interface, making it easier for teams to assess data reliability and track issues across various datasets and domains.
This centralized approach enables proactive data management by highlighting potential problems early. Teams can prioritize issues, address root causes, and ensure that data quality remains consistent, ultimately reducing the time and effort spent on the data issues resolution process.
3. Increased collaboration
Data quality dashboards provide a single source of truth for better collaboration between data producers and consumers. These dashboards offer shared visibility into the data quality landscape, making it easier for teams to align on data contracts, resolve discrepancies, and drive continuous improvement.
Collaboration is further enhanced when business and technical teams can use dashboards tailored to their specific needs. For example, business users can track trends and insights, while technical users like data engineers can focus on alerts and root-cause analysis. This alignment ensures that data is effectively utilized across the organization.
4. Enhanced AI initiatives
AI and ML models thrive on high-quality data. Dashboards equipped with advanced quality metrics enable organizations to continuously monitor the data being fed into these models, ensuring accuracy, completeness, and timeliness. This builds trust in AI-generated insights and improves the reliability of predictions.
Moreover, data quality dashboards help organizations identify and resolve data issues that could otherwise derail AI projects. By ensuring that data meets the highest quality standards, organizations can accelerate their AI/ML initiatives and achieve their AI-readiness goals with confidence.
5. Regulatory compliance
Industries like finance and healthcare operate under stringent compliance requirements, where even minor data errors can lead to severe consequences. Data quality dashboards provide organizations with capabilities to monitor and manage compliance-related metrics, ensuring adherence to regulatory standards.
By proactively identifying gaps and anomalies, these dashboards help organizations mitigate risks and maintain audit-ready data. This not only ensures compliance but also safeguards the organization’s reputation and fosters trust with regulators and customers alike.
Data Quality Dashboards with DQLabs
DQLabs, the modern data quality and observability platform provides out-of-the-box data quality dashboards for various different personas.
Discover dashboards
These dashboards are designed for Data and business leaders. They come with prebuilt templates tailored for end users, focusing on search and discovery to help uncover valuable assets, enhance data literacy, and foster a strong data-driven culture.
The discover page provides the details of number of sources, data assets, total attributes and number of data quality checks enabled. This gives a comprehensive overview of the organization’s data quality landscape.
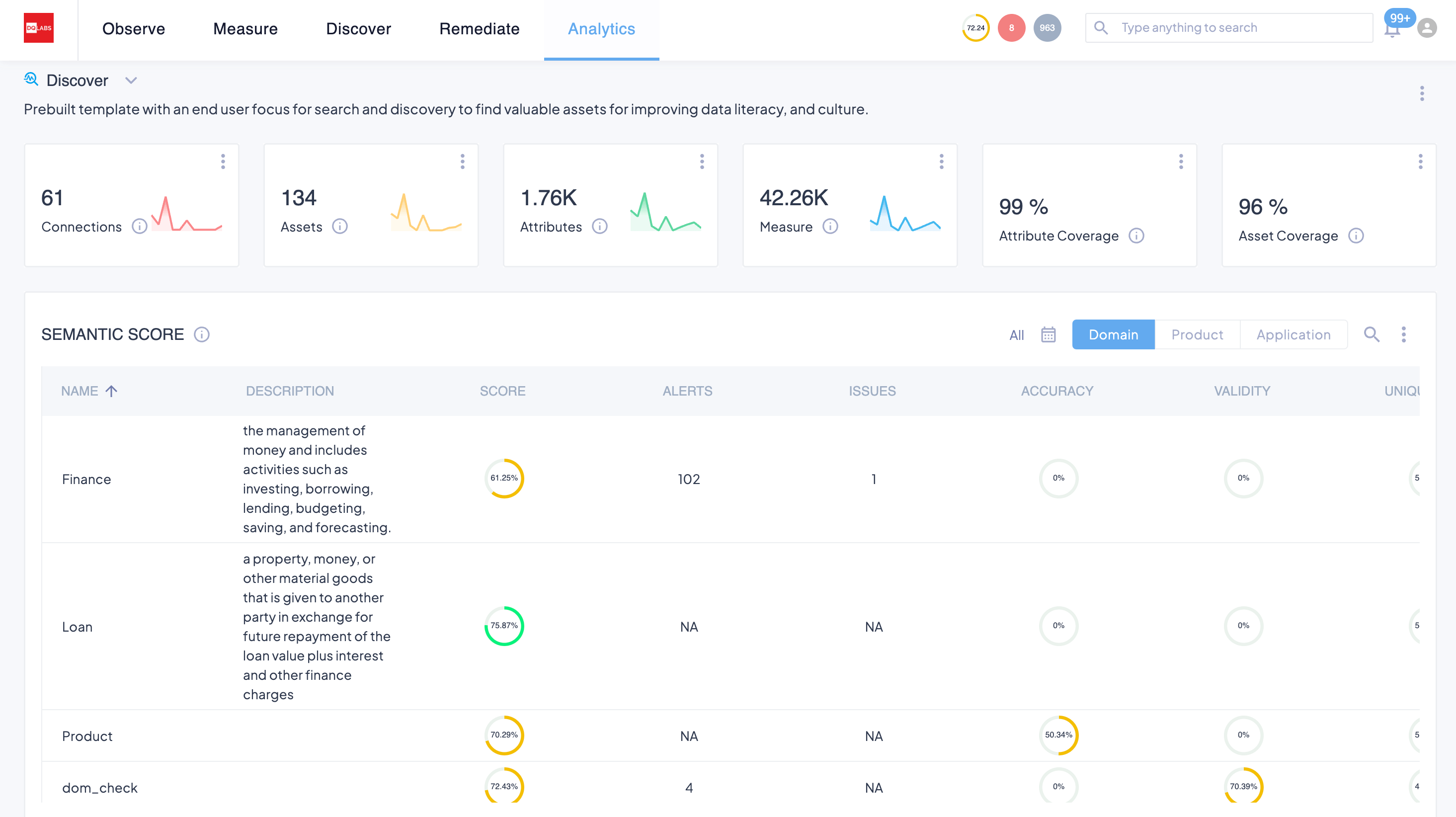
Measure dashboards
Measure dashboards cater to data scientists, data analysts, and data stewards personas. These dashboards provide focused quality metrics and alerts for centralized and comprehensive stewardship and agile data governance.
Here are some of the key measure dashboards that provide critical insights into data quality:
- Organizational score: This chart displays the data quality score over a period of time for various semantics components: Domain, product, application, and tags.
- Asset score by dimension: Represents the top 10 and bottom 10 assets in the organization based on their data quality score. It helps users to quickly identify high and low performance assets for specific data quality dimensions.
- Overall Quality: DQLabs provides a spider chart that highlights the data quality across various dimensions.
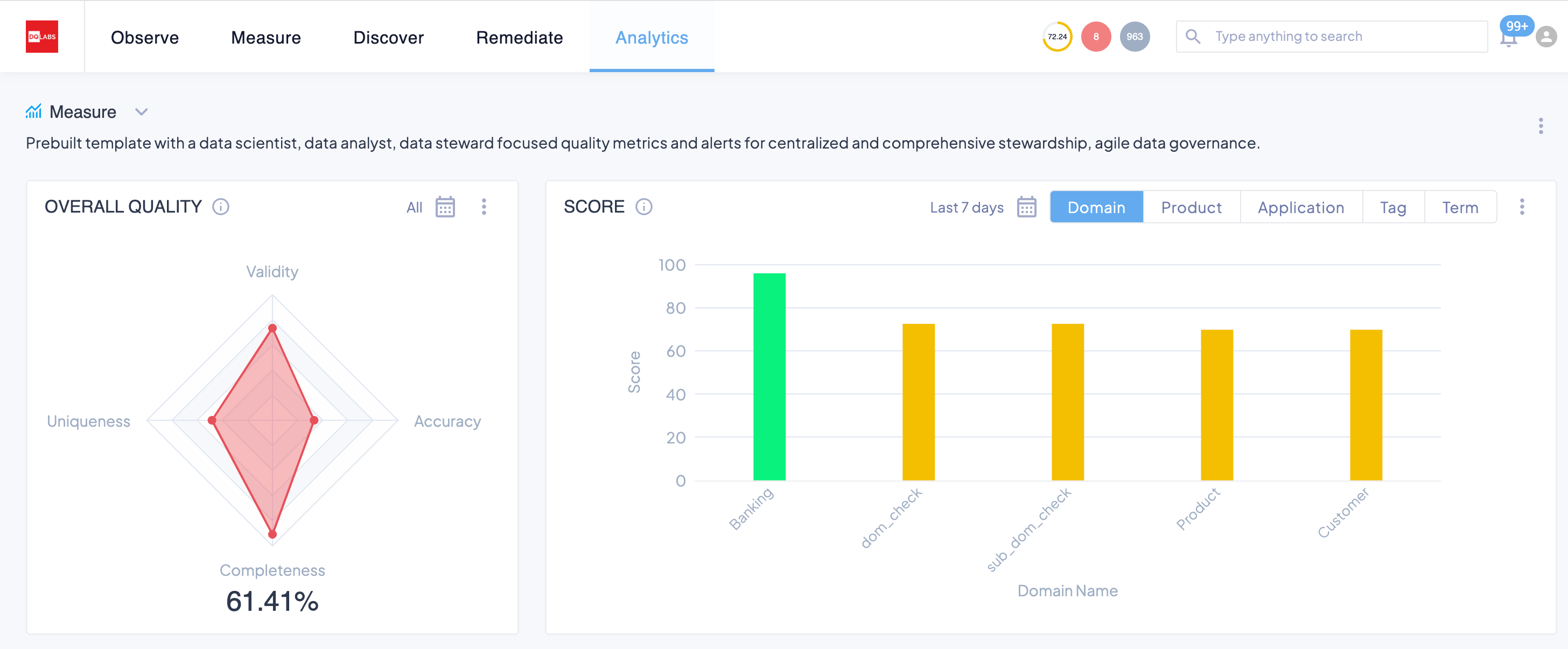
Observe Dashboard
These dashboards provide focused observability metrics and alerts with monitoring for full insights into the data ecosystem for data engineering personas. The Observe dashboard offers visibility into issues and alerts with out-of-the-box ease. These dashboards empower data engineers to cut to the chase of the priority, severity, and longevity of an issue or an alert, so they know immediately what needs attention.
Here are some of the key observe dashboards that deliver comprehensive visibility into your data ecosystem:
- Alerts by category: This dashboard provides the total number of alerts tracked over a period of time. The dashboard highlights the alerts across different health measures like – frequency, distribution, reliability, and statistics.
- Alerts by dimensions: This chart provides the number of alerts across key data quality dimensions – accuracy, completeness, uniqueness, validity, and timeliness. This helps users to segregate and analyze alerts for specific dimensions.
- Alert to issues: The alerts to issues funnel chart represents the total number of alerts created and how many of those alerts are being converted into issues and finally resolved. This helps users to track the progress of alerts and issues of their data landscape.
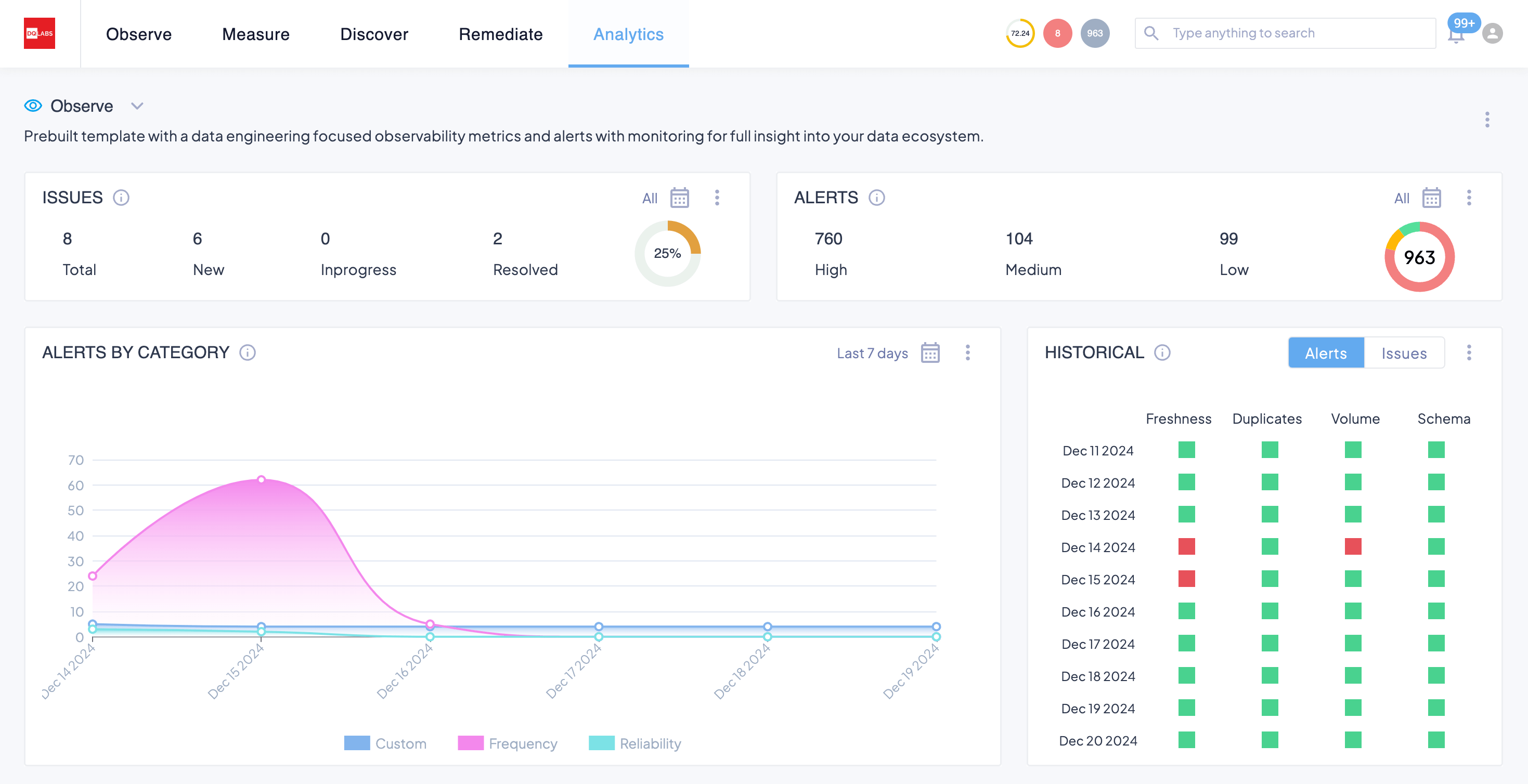
In addition to the out-of-the-box dashboards, DQLabs also provides customizable dashboards. These custom dashboards allow users to understand Data Quality in a way that makes sense as per their own visualization requirements.
Next Steps
Ensuring reliable data quality is critical for organizations aiming to make data-driven decisions, achieve AI-readiness, and maintain regulatory compliance. Data quality dashboards play a key role in enabling organizations to monitor, measure, and improve their data health in real time. By providing actionable insights, visualizing key metrics, and identifying issues early, data quality dashboards empower teams to proactively address data challenges and build trust in their data.
DQLabs offers a modern and comprehensive platform with out-of-the-box and customizable data quality dashboards tailored for various personas—data leaders, analysts, stewards, and engineers. With DQLabs, organizations can gain a centralized view of their data quality landscape, resolve issues efficiently, and ensure data reliability across all domains.
Take control of your data quality with DQLabs. Monitor your data health, resolve issues faster, and build trust across your data ecosystem—schedule a personalized demo today!