How to Improve Your Financial Data Quality Management
How to Improve Your Financial Data Quality Management https://www.dqlabs.ai/wp-content/uploads/2024/07/thumbnail-8-1024x575.webp 1024 575 DQLabs DQLabs https://www.dqlabs.ai/wp-content/uploads/2024/07/thumbnail-8-1024x575.webpFinancial services are a complex ecosystem built on trust and meticulous record-keeping. They undoubtedly reap significant advantages from their data resources. However, the potential business value can be diminished or even jeopardized if the data is of poor quality. Inaccurate data can lead to inefficiencies and flawed decision-making. Furthermore, financial firms risk regulatory compliance breaches when relying on subpar data. Hence, financial data quality management is cardinal due to the industry’s stringent regulatory requirements and focus on compliance and risk management.
What is Data Quality in Financial Services
Financial institutions rely on data that’s accurate, complete, reliable, and timely, especially when it comes to customers. This translates to ensuring customer information is free of errors, current, comprehensive, and trustworthy enough to guide sound decision-making. Here, data quality isn’t just about usability; it’s about ensuring data fulfills its intended purpose. Any data that fails to meet this critical standard is considered poor quality and requires verification before use.
Financial institutions, encompassing banks, insurers, mortgage lenders, investors, and creditors, rely heavily on data for numerous business processes. This data fuels:
- Financial Reporting: Accurate statements and reports for internal use and customer transparency.
- Loan Approvals: Streamlined underwriting processes for efficient loan decisions.
- Fraud Detection: Identifying and preventing fraudulent activities like stolen information or fabricated applications.
- Credit Risk Management: Predicting loan delinquency rates to mitigate financial risks.
- Risk Assessments: Evaluating operational and credit risks associated with financial decisions.
Poor data quality can significantly disrupt these processes and their outcomes. Feeding inaccurate data into these systems can have detrimental consequences, jeopardizing the credibility of financial institutions. Therefore, ensuring clean and reliable data is paramount for success in the financial sector.
Importance of Data Quality in Financial Services
In the financial services sector, data is deeply integrated into operations, making it essential for the data to be accurate and error-free. High-quality, clean data fosters trust between customers and their financial institutions, such as banks and insurance companies.
Let’s explore why financial data quality management is critical and the benefits it provides.
- Risk Assessment, Planning, and Mitigation: Financial activities inherently involve risk, whether investing in ventures, lending money, or approving loans and mortgages. Intelligent risk planning is essential for success in the financial world. Accurate data analysis and risk assessment enable better decision-making regarding expected returns, profitability, and alternative options. Correct and relevant data is necessary to avoid financial risks and potential losses.
- Fraud Detection and Prevention: Poor data quality makes banks, insurance companies, and investors more vulnerable to fraudulent activities. Data quality gaps allow fraudsters to commit identity theft, submit fake applications, bypass checks, and launch attacks on sensitive information. Clean and accurate data helps detect anomalies, which in turn can prevent fraud.
- Digitization of Financial Processes: Digital banking, online payments, and credit requests are transforming the financial industry. These services rely on high-quality data for successful implementation. Many financial professionals still use physical files due to scattered data requiring manual intervention. Improved data quality could enable this digitization of financial services.
Nearly a quarter (24%) of insurers express concern about the quality of data they rely on for risk evaluation and pricing”, reports Corinium Intelligence
- Customer Loyalty: Consolidating and matching customer records to create a comprehensive view enables personalized experiences and ensures privacy and security. Scattered data from various sources, including local files, third-party applications, and web forms, hinders the ability to provide connected customer experiences and build trust.
- Regulatory Compliance: Standards like Anti Money Laundering (AML) and Counter Financing of Terrorism (CFT) require financial institutions to review and revise their data management practices. To comply, institutions must monitor client transactions to detect financial crimes, such as money laundering and terrorism financing. Insufficient data monitoring could hamper the ability to report suspicious activities to authorities promptly.
- Facilitation of Predictive Analytics: Data science advancements allow real-time predictions and insights in finance. Investors use predictive analytics to gauge the feasibility of market investments or determine the profitability of stocks. These predictions rely on high-quality data. Accurate data enables data analysts and scientists to make reliable predictions about financial outcomes.
- Accurate Credit Scoring for Loan Approvals: When lending money, understanding the risk of the decision is crucial for investors and bankers. They must verify the applicant’s identity and credit score and calculate the loan’s value and interest rate or else it could be another Equifax fiasco! Good financial data quality management eliminates discrepancies and delays in the underwriting process, ensuring investment in the right individuals.
Ensuring financial data quality management not only improves operational efficiency but also builds trust and safeguards against risks and fraud, ultimately contributing to a more robust financial system.
Frequent Data Quality Issues Found
Having explored the significant benefits of data quality in financial services, let’s delve deeper. We’ll examine the specific challenges faced by different sectors within finance, such as banking and insurance companies, when data quality falls short.
DQ ISSUE | EXAMPLE |
Inaccurate data | A customer’s full legal name is misspelled in the loan agreement. |
Missing data | Two out of fifteen covenants in a loan agreement are left blank. |
Duplicate records | Duplicate customer records enable multiple loan applications. |
Inconsistent units | International transactions use local currencies instead of a standard unit like the US dollar. |
Inconsistent formats | Customer phone numbers are recorded in different formats, with some having international codes and others missing area codes. |
Outdated information | Transactions are delayed in appearing in customer records, leading to potential errors in computations. |
Incorrect domain | The currency codes used are not part of the ISO standard. |
Inconsistency | Different exchange rates are applied to various customer segments within the organization. |
Irrelevance | Employees must apply multiple filters, sorting, and prioritization to obtain the necessary information. |
How Can Financial Services Improve Data Quality?
Improving data quality in financial services requires a comprehensive approach. Key elements include encouraging a data-centric culture, understanding prevalent quality issues, and using technology-driven solutions. Here are a few effective strategies financial services organizations can implement to significantly improve their data quality.
1. Building a Culture of Data Quality
- Leadership Buy-in: Secure the commitment of senior management by highlighting the negative impact of poor data quality on metrics like customer churn and regulatory compliance. Showcase data profiling reports to illustrate the specific data issues plaguing your institution.
- Quantify the Cost: Utilize the Friday Afternoon Measurement (FAM) method to estimate the financial losses incurred due to inaccurate data. This helps you build a case for data quality initiatives and get budgetary support from the top executives.
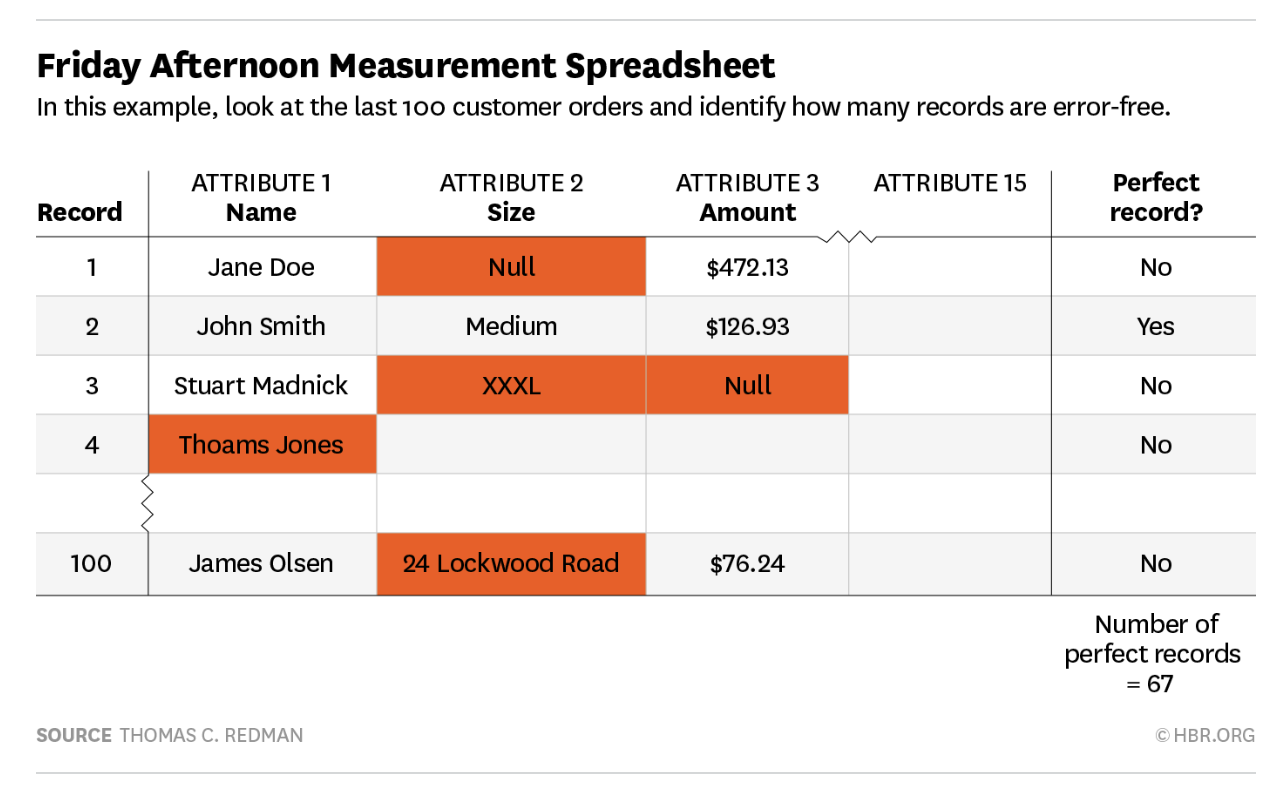
- Employee Training: Implement data quality training programs for all employees who interact with customer data. These programs should educate staff on best practices for data entry, storage, and security, fostering a data-centric culture across the organization. Here are some tips for implementing effective employee training:
-
- Regular Training Sessions: Conduct regular sessions to keep employees updated on customer data quality standards and best practices.
- Role-Specific Training: Implement training tailored to different roles, such as customer service and account management.
- Create a Data Quality Culture: Promote a culture of data quality through internal communications and incentives for good practices.
2. Establishing a Data Governance Framework
Consider the following when creating a data governance framework:
- Define Roles and Responsibilities: Clearly outline who owns and manages different data sets. Establish accountability for data accuracy and compliance with regulations.
- Data Rules and Standards: Develop clear guidelines for data collection, storage, and usage. These rules should ensure consistency, minimize errors, and protect sensitive information.
- Regular Monitoring and Auditing: Schedule routine data audits to identify and address potential issues. Utilize automated tools to streamline the auditing process and ensure consistent data quality monitoring.
- Communication and Education: Keep all staff informed about data governance policies and procedures. Regularly communicate updates and best practices to promote data stewardship across the organization.
Having clear procedures in place, can help you keep customer data consistent and accurate. You can read a detailed account of the complete plan on how to improve data quality here.
3. Implementing Data Quality Controls
- Multi-Level Approach: Implement data quality checks at different stages. Here’s a breakdown:
- Initial Level: Start with quick fact-checking and fixing visible data quality issues, ensuring the dataset is complete, accurate, and standardized.
- Second Level: Perform deeper statistical analysis to compute standard variations of numerical values and catch anomalies. Data profiling is a good technique for this level.
- Third Level: Ensure proactive financial data quality management using complex machine learning and AI tools to predict potential data quality issues in real-time.
- Data Profiling: Leverage data profiling tools to expose errors, inconsistencies, and missing data within your datasets. Analyze data patterns, outliers, and anomalies to identify areas for improvement.
DQLabs offers deep data profiling with automatic tracking of row counts, data freshness, schema consistency, and duplicate detection at the metadata level. Dive deep into column-level profiling to ensure your data is always fit for purpose → Try now
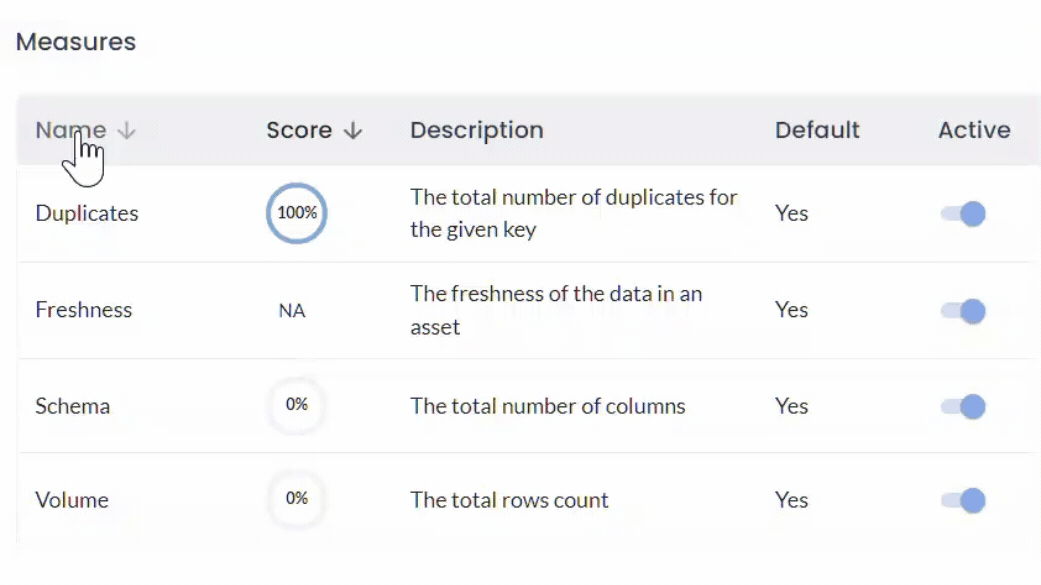
- Automated Data Validation: Invest in data quality management platforms that offer automated data validation features. These tools can perform real-time data checks, error correction, and duplicate removal, significantly reducing manual effort and human error.
- Data Deduplication: Address the challenge of duplicate records by employing data quality frameworks that match and consolidate duplicates. Use a combination of unique identifiers and fuzzy matching techniques to ensure accurate consolidation.
4. Leveraging Technology for Continuous Improvement
-
- Data Quality Tools: Invest in modern data quality solutions like DQLabs with augmented data quality and data observability to automate various data quality tasks. Tools like this will help you streamline processes for real-time data checks, error correction, and duplicate removal.
- Standardized Data Formats: Implement standardized data formats and protocols across the organization. This eases seamless data integration and reduces errors coming from incompatible data structures.
- Data Security and Privacy: Prioritize data security and privacy by employing robust access controls. Implement role-based access control (RBAC) and data encryption to safeguard sensitive customer information. Additionally, stay updated on data privacy regulations (like GDPR and CCPA) to ensure compliance and maintain customer trust.
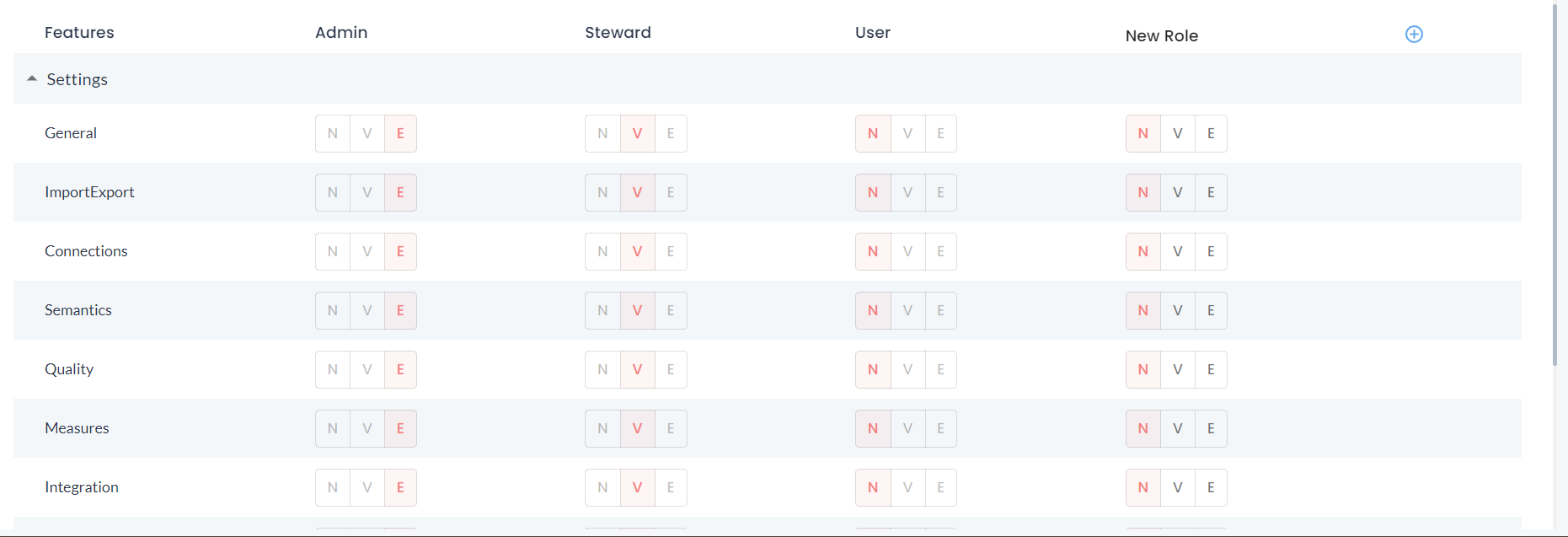
By implementing these steps, financial institutions can establish a strong foundation for data quality.
Wrapping it up
With growing stringent norms and regulations in the financial services industry, maintaining high data quality is critical for accurate decision-making and operational efficiency. Predictive and continuous data quality solutions provide an effective means to streamline processes and ensure reliable, real-time results. By leveraging these advanced tools, financial institutions can monitor foreign exchange rates, track intraday positions, identify anomalies in security reference data, manage credit risk in bank lending, accelerate cloud adoption, and monitor fraud and cyber anomalies in real time.
These solutions automate and optimize the labor-intensive task of data monitoring, using machine learning and predictive analytics to detect errors and inconsistencies without manual intervention. This proactive approach not only enhances data integrity but also significantly reduces the time and resources required for data management and regulatory compliance.
By implementing robust data quality frameworks and technologies, financial institutions can mitigate risks associated with poor data quality, improve customer trust, and gain a competitive edge. As financial services increasingly rely on digital platforms, the importance of accurate, high-quality data cannot be overstated. Investing in predictive data quality solutions ensures that financial organizations can deliver trustworthy results, enhance operational efficiency, and stay ahead in a rapidly evolving market.
Prioritize data quality with DQLabs for the longevity of your financial institution. Schedule a personalized demo today to know how DQLabs solves the unique challenges of working with financial data.