Why did Databricks Acquire Tabular
Why did Databricks Acquire Tabular https://www.dqlabs.ai/wp-content/uploads/2024/06/thumbnail-2-1024x575.webp 1024 575 DQLabs DQLabs https://www.dqlabs.ai/wp-content/uploads/2024/06/thumbnail-2-1024x575.webpThe data world was just absorbing Snowflake’s announcement of the Polaris Catalog – its new open-source catalog implementation (designed for Apache Iceberg) – when, just two days later, Databricks announced its acquisition of Tabular, a data management company for a billion dollars. Tabular is a data management company that provides a storage platform designed and developed by the creators of Iceberg at Netflix.
This has made things very interesting for the data world. In this potential ‘Iceberg War’ scenario, we’ll analyze the rationale behind the acquisition and its possible impact on the data world.
But first, we need to dive into the concept of Apache Iceberg.
Apache Iceberg: brief introduction
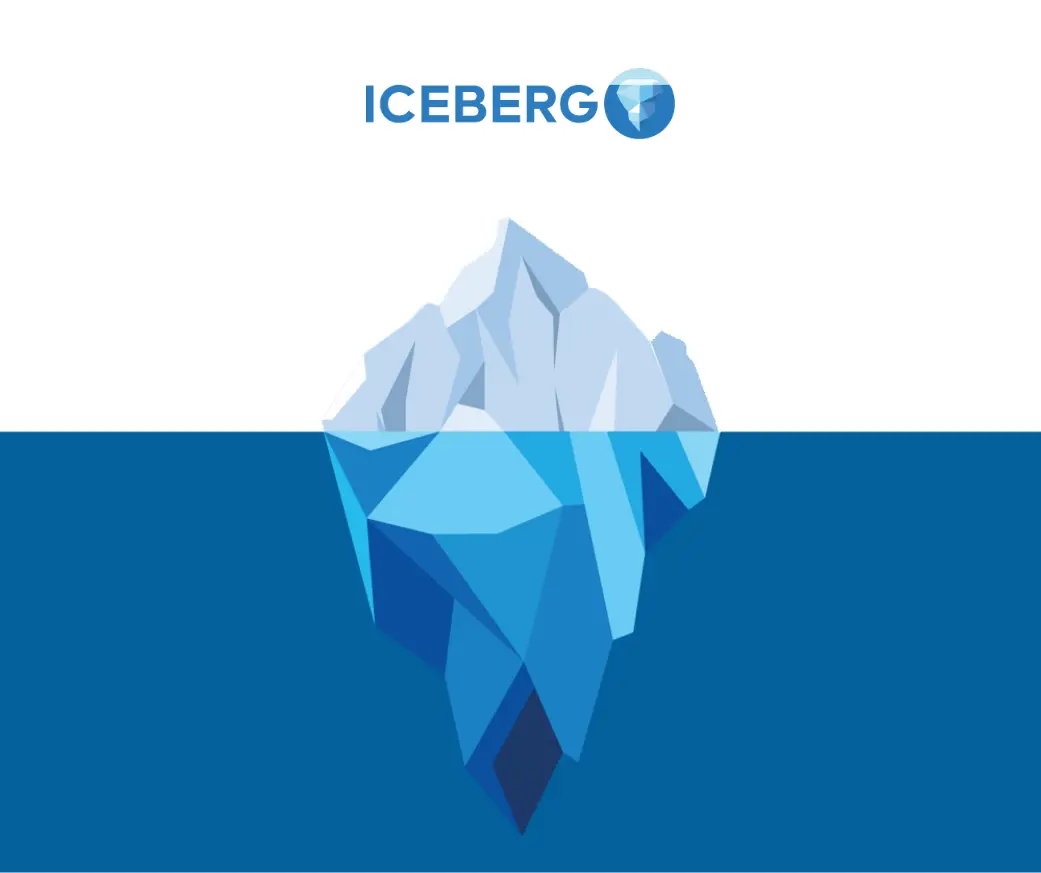
Apache Iceberg is an open-source table format designed for large analytics datasets, in order to improve the performance of an organization’s data lake operations. A data lake provides a low-cost data storage option for structured and unstructured data of any scale. However, organizations often find themselves dealing with various limitations that come with data lake implementation, including schema evolution, no transaction support (ACID), poor data accessibility, etc., making data management and accessibility really difficult. This is where Iceberg arrives as a game changer. By addressing common data management issues of data lakes, Iceberg improves data lake performance and reliability.
We will walk you through a few features of Apache Iceberg, to give you a flavor of its capability (this, however, is not an exhaustive list)
Schema evolution: When you add new columns, rename/delete existing columns, or change data types, these data operations change the schema of your datasets. Traditional data lake solutions don’t provide efficient handling of schema evolution or schema drift which causes significant data downtime. Iceberg provides the unique capability to manage schema evolution, without any additional preparation work, empowering organizations to better manage their data operations.
Transactional support: Iceberg provides transactional support for ACID operations and ensures that data manipulated by different users and applications is integrated and consistent. This enables reliability of data pipeline operations and application robustness which is not provided by traditional data lake solutions.
Time travel and rollback: Iceberg provides unique capabilities of time travel and rollback that change the way you interact with data. With these features, you can access any data at any point in the past and can even restore the data to its previous state with rollback options. These capabilities enable users to track and analyze data changes over time and empower organizations to effectively implement their data governance and regulatory compliance initiatives.
Scalability and flexibility: Iceberg provides compatibility with popular computational engines like Spark, Trino, Impala, Hive, etc. and enables scalability and flexibility of organizations’ data analytics needs.
With these features and functionalities, Apache Iceberg provides an abstraction layer over your data lake and creates a data lakehouse architecture. This provides organizations with the benefits of both, a data lake and a data warehouse. The idea behind this architecture is to leverage the data structure flexibility and low-cost storage capabilities of data lakes while combining them with enhanced data access abilities and performance optimization of data warehouses.
Databricks, Data Lakehouse and Delta Lake
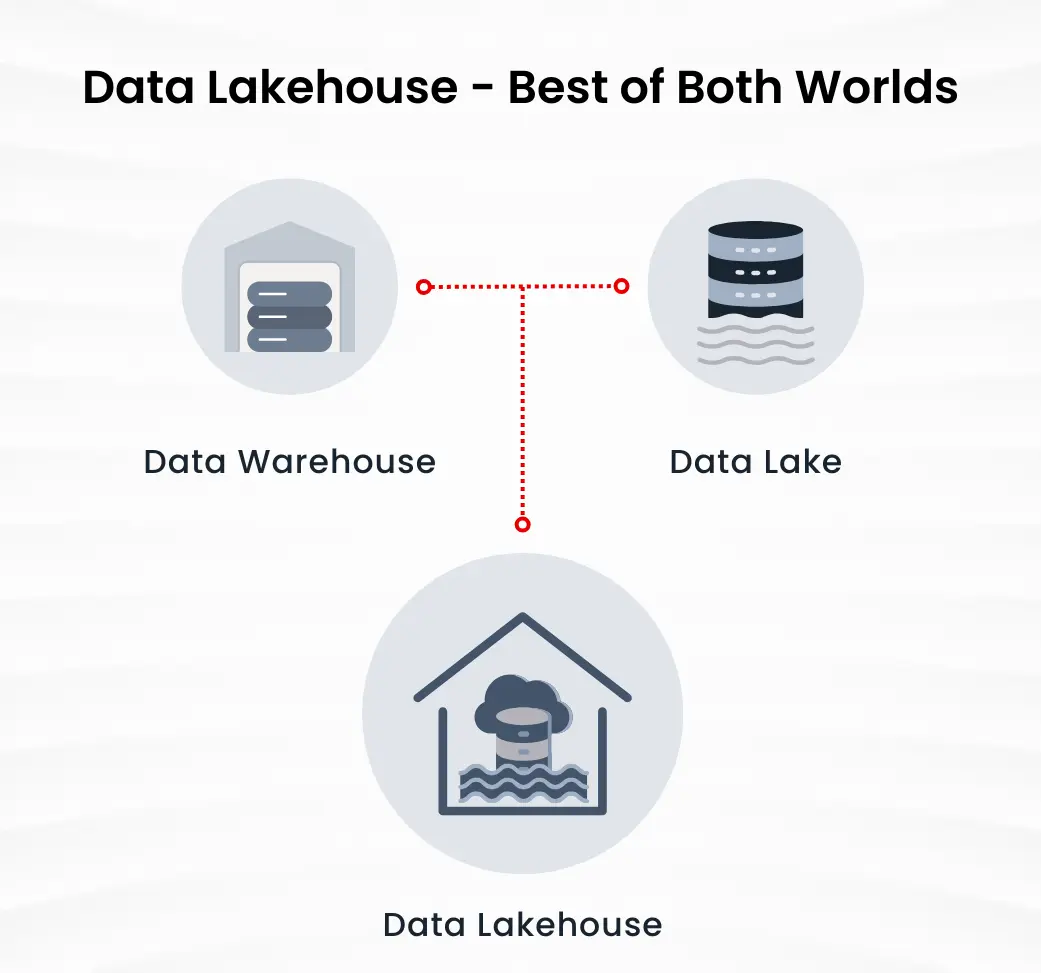
In 2020, Databricks developed the concept of a lakehouse architecture, with the vision of improving the reliability and performance of data analytics and management workloads. This brought about a synergy of data lake and data warehouse capabilities, for the first time in the industry. For this to work, it required data to be in an open format, so that different workloads and analytics engines could access the data to maximize performance and enable data democratization.
The open-source table format was the foundation of the lakehouse architecture that has enabled ACID transactions on object storage (S3, Azure Blob Storage, GCS), performance, reliability, and integrity of data lakes. To make this possible, Databricks collaborated with the Linux Foundation on the Delta Lake Project. Delta Lake is Databricks’ open table format (like Iceberg) that made lakehouse architecture a reality.
Ryan Blue and Daniel Weeks (co-founders of Tabular) developed the Iceberg Project at Netflix around the same time as the Delta Lake project. Since then, Delta Lake and Iceberg have become popular open-source formats for lake house architecture. Even though Delta Lake and Iceberg have the same open-source format, organizations have to choose one of the formats because of their incompatibility for workloads which defeats the purpose of a truly open lakehouse.
The vision behind the acquisition
With the acquisition of Tabular, Databricks will have the capabilities of two of the most popular open-source data formats for lakehouse architecture. Tabular is perceived as one of the largest contributors to Iceberg, with its unique value proposition for making Iceberg workloads cost-effective and easier to manage. This will give them an advantage over their competitors, like Snowflake, in the current data and AI landscape. Databricks has envisioned the interoperability between Iceberg and Delta Lake to reduce the current incompatibility between the two formats. This will open new possibilities for organizations’ data and analytics workloads and won’t create a lock-in with one particular format.
Valuation and IPO
Databricks is also working towards a potential IPO. The powerful addition of Tabular will surely increase its brand value, with a possible perceived competitive advantage and this could shoot up its valuation, ahead of the impending IPO.
Interoperability
In 2023, Databricks introduced Delta Lake Uniform, to increase the interoperability, i.e., the capability to work with popular open-source table formats like Delta Lake, Iceberg, Hudi, etc. With the addition of Tabular, Databricks will accelerate the process of making two of the most open-source table formats more compatible and enable enhanced interoperability of analytics workloads.
No more Delta Lake v/s Iceberg!
Until now, for all lakehouse architecture implementations, there was a split between Delta Lake and Iceberg. Databricks, with this acquisition, will probably try to address this issue, by working with open-source communities and making these formats compatible and providing users with unprecedented feasibility for their data and analytics initiatives.
Conclusion
The acquisition of Tabular by Databricks marks a significant development in the data management industry, particularly regarding lakehouse architectures. By bringing the expertise of Tabular and planning to create a synergy between Iceberg and Delta Lake, Databrick has strengthened its position in the data management space. This decision has also accelerated the realization of a truly open and interoperable data ecosystem, a privilege that the industry hasn’t been able to enjoy so far. With this unique vision, Databricks has unlocked new possibilities for organizations especially to drive even more innovation and growth in the data world.