What is Data Quality? Why is it Important For AI Readiness?
What is Data Quality? Why is it Important For AI Readiness? https://www.dqlabs.ai/wp-content/uploads/2024/04/1-1024x575.png 1024 575 DQLabs DQLabs https://www.dqlabs.ai/wp-content/uploads/2024/04/1-1024x575.pngIn today’s data-driven world, artificial intelligence (AI) promises to revolutionize everything from healthcare diagnostics to personalized marketing. But for AI to truly deliver on its potential, it needs a solid foundation: high-quality data. Moreover, in industries such as healthcare, finance, and cybersecurity, where AI-driven insights drive critical decisions, the importance of data quality cannot be overstated. Flawed or incomplete data can have far-reaching consequences, impacting patient outcomes, financial stability, or security posture.
The “garbage in, garbage out” problem in AI
Imagine feeding a high-powered engine with dirty fuel. That’s essentially what happens when you train AI models on poor-quality data. Inaccuracies, inconsistencies, and missing values can lead to biased models, inaccurate predictions, and ultimately, failed AI initiatives.
A study by Gartner revealed that data quality issues are the leading cause of poor AI project performance, costing businesses millions. Forrester further emphasizes that dirty data can lead to biased AI models, resulting in discriminatory outcomes and ethical concerns.
Data Quality: Critical Foundation to Unlocking AI’s Potential
Data quality refers to the reliability, accuracy, consistency, and relevance of data for its intended use. It encompasses various dimensions, including completeness, timeliness, validity, and consistency. Essentially, high-quality data is data that is fit for purpose, free from errors, and aligned with organizational objectives. Clean, accurate, and reliable data is the fuel that powers effective AI models.
Benefits of good data quality for AI
Here’s what is good data quality brings to the table:
- Improved accuracy and reliability: High-quality data allows AI models to learn from accurate patterns and make reliable predictions. This translates to better decision-making across the organization.
- Reduced bias: Cleansed and unbiased data helps mitigate the risk of AI models perpetuating existing societal biases. This is crucial for ensuring ethical and responsible AI implementation.
- Enhanced efficiency: When data is readily available and well-organized, AI models can train faster and deliver results quicker. This streamlines workflows and accelerates time-to-insight.&nsbp;
How to ensure data readiness for AI?
Poor-quality data can lead to biased, inaccurate, or unreliable AI models, undermining their effectiveness and potentially leading to erroneous decisions. So, how do you ensure your organization is prepared to leverage AI effectively?
Data profiling and assessment
Data profiling involves analyzing the current state of your data to understand its completeness, accuracy, consistency, and format. By conducting a thorough assessment of your data, you can identify any existing issues or anomalies that may affect the performance of AI models. For example, you can discover missing values, inconsistent data formats, or inaccuracies that need to be addressed before proceeding with AI implementation.
Example: A financial services company is planning to implement AI-powered credit scoring models. Before deploying these models, the company conducts data profiling and assessment to evaluate the quality of its customer data. During this process, they discover inconsistencies in the formatting of customer addresses and missing values in certain fields. By addressing these issues through data cleansing and standardization, the company ensures that its AI models receive high-quality input data, leading to more accurate credit risk assessments.
Data cleansing and standardization
Data cleansing involves the process of detecting and correcting errors or inconsistencies in your data. This may include addressing missing values, removing duplicate entries, or correcting inaccuracies. Standardization ensures that data adheres to a defined format or structure, making it easier to process and analyze.
Example: A retail company collects customer data from various sources, including online transactions, in-store purchases, and loyalty programs. However, this data is often riddled with errors, such as misspelled names, inconsistent address formats, and duplicate entries. To ensure the accuracy of its AI-powered customer segmentation models, the company implements data cleansing and standardization techniques. This involves using algorithms to identify and correct errors, as well as enforcing consistent formatting rules across all customer data sources.
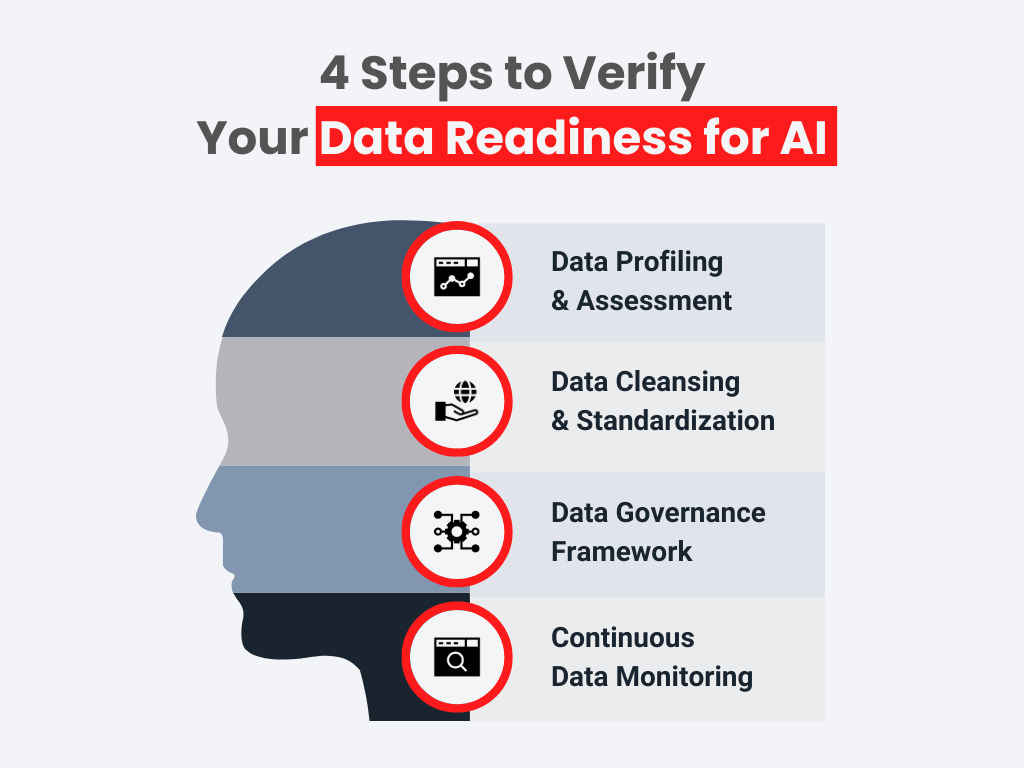
Data governance framework
Establishing a robust data governance framework is essential for maintaining data quality and integrity. This framework defines clear ownership, accountability, and access controls for data management processes. It ensures that data is handled responsibly and ethically throughout its lifecycle, from collection to disposal.
Example: A healthcare organization collects vast amounts of patient data, including medical records, diagnostic tests, and treatment histories. To ensure compliance with privacy regulations and maintain data integrity, the organization implements a comprehensive data governance framework. This framework includes policies and procedures for data access, usage, and security, as well as mechanisms for monitoring and enforcing compliance. This allows the organization to protect patient confidentiality and privacy thus preventing AI abuse.
Continuous data monitoring
Data quality is not a one-time effort but requires ongoing monitoring and maintenance. Regular assessments of data quality allow organizations to proactively identify and address issues before they impact AI initiatives. This may involve implementing automated monitoring tools, establishing data quality metrics, and conducting periodic audits.
Example: An e-commerce company relies on AI algorithms to personalize product recommendations for its customers. To ensure the accuracy of these recommendations, the company implements continuous data monitoring processes. This involves monitoring key data quality metrics, such as customer engagement rates and conversion rates, and conducting regular audits to identify any deviations or anomalies. By proactively addressing data quality issues, the company maintains the reliability and effectiveness of its AI-powered recommendation engine.
Role of Comprehensive Tools & Technologies
While prioritizing data quality is the first step towards a strong foundation for AI, achieving true AI readiness requires a comprehensive approach throughout the entire data lifecycle. This goes beyond just acquiring and storing data; it involves implementing robust data governance frameworks, ensuring clear data lineage and traceability, and enforcing strict data quality standards. Advanced technologies like machine learning and natural language processing can further automate data quality assessment and remediation efforts. By prioritizing clean data across all stages, you’re not just building a foundation for AI, but fostering a culture of data-driven decision-making across the organization.
Understanding the quality of your data is a crucial first step on your AI journey. By establishing a data governance practice that prioritizes accuracy and reliability, you can ensure your AI models are built on a strong foundation. Remember, high-quality data is the foundation for unlocking the true potential of AI in your organization.
This blog has explored the importance of data quality, but for a deeper dive into assessing your data, consider data quality solutions available from providers like DQLabs. DQLabs offers a Modern Data Quality Platform that addresses the complex challenges faced by modern enterprises. Our platform utilizes automation, contextual insights, and advanced analytics to empower organizations to gain a deeper understanding of their data. This, in turn, can drive informed decision-making and fuel AI-driven innovation across your organization.